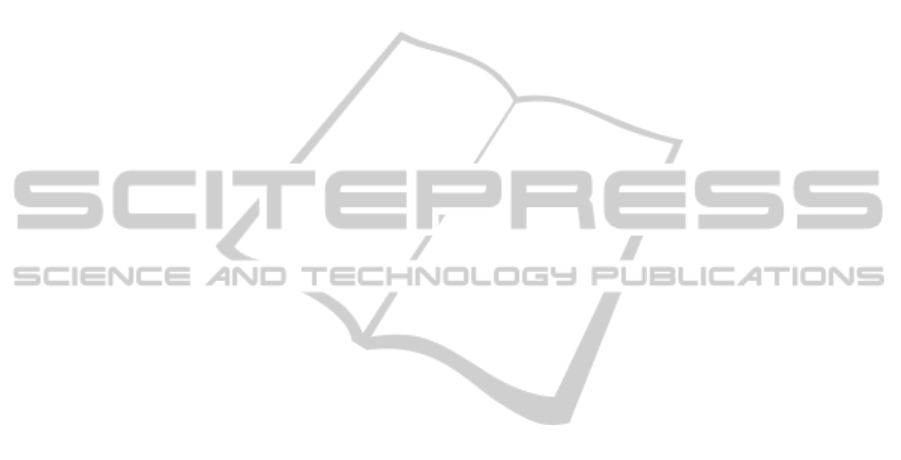
(https://github.com/NEUROINFORMATICS-GROUP
-FAV-KIV-ZCU/sehr.
The second version of archetypes is planned to use
more complex neurophysiological ontology. The next
archetype lifecycle states (candidate and published)
will come with the second version.
6 CONCLUSION
The work describes a concept of a new EHR system
based on openEHR. It is focused on measured
subjects/users, not on physicians. Its main idea is
to provide an easily extensible system, which is able
to store daily measured medical data. These data
can be further analysed and shared. The openEHR
concept leads to the new archetype proposals. The
electroencephalographydomain was the first use-case
and new archetypes derived from the EEGBase data
structure were proposed. Moreover, archetypes
respect odML terminology.
Archetypes are currently in the lifecycle state
Author’s draft. The future work will focus on
testing these archetypes. With tested archetypes
an EEG plugin for personal EHR system will be
developed. After that, new versions of archetypes
will be designed and implemented; those archetypes
will be based on more complex electrophysiological
ontology OEN. The future development of the EHR
system itself is shown in Figure 3.
ACKNOWLEDGEMENT
The work was supported by the European Regional
Development Fund (ERDF), Project ”NTIS - New
Technologies for Information Society”, European
Centre of Excellence, CZ.1.05/1.1.00/02.0090
and UWB grant SGS-2013-039 Methods and
Applications of Bio and Medical Informatics.
REFERENCES
Beale, T., Heard, S., Kalra, D., and Lloyd, D. (2005).
Archetype definition language (adl). OpenEHR
specification, the openEHR foundation.
Beale, T., Heard, S., Kalra, D., and Lloyd, D. (2006).
Openehr architecture overview. The OpenEHR
Foundation.
Bruha, P., Papez, V., Brandowski, A., Grewe, J., Moucek,
R., Tripathy, S., Wachtler, T., and Le Franc,
Y. (2013). A formal ontology for describing
experimental neurophysiology. Neuroinformatics.
Dolin, R. H., Alschuler, L., Boyer, S., Beebe, C., Behlen,
F. M., Biron, P. V., and Shvo, A. S. (2006). Hl7
clinical document architecture, release 2. Journal
of the American Medical Informatics Association,
13(1):30–39.
Frishkoff, G., LePendu, P., Frank, R., Liu, H., and Dou, D.
(2009). Development of neural electromagnetic
ontologies (nemo): ontology-based tools for
representation and integration of event-related
brain potentials. In ICBO09: Proceedings of the
international conference on biomedical ontology,
pages 31–34. Citeseer.
Grewe, J., Wachtler, T., and Benda, J. (2011). A bottom-up
approach to data annotation in neurophysiology.
Frontiers in neuroinformatics, 5.
Heather, L. (2008). openehr/adl-archetypes.
Heather, L. (2010). openehr/adl-archetypes.
Jezek, P. and Moucek, R. (2012). System for eeg/erp
data and metadata storage and management. Neural
Network World, 22(3):277–290.
May, L. (2004). The national e-health transition authority
(nehta). The HIM journal, 34(1):19–20.
McNicoll, I. (2010). openehr/adl-archetypes.
NIX, G.-N. (2014). G-node/nix.
Sato, L. and Luhn, K. (2007). Cen/iso 13606 pilot study
final report. NHS Connecting for Health [Online].
Stearns, M. Q., Price, C., Spackman, K. A., and Wang,
A. Y. (2001). Snomed clinical terms: overview
of the development process and project status. In
Proceedings of the AMIA Symposium, page 662.
American Medical Informatics Association.
HEALTHINF2015-InternationalConferenceonHealthInformatics
616