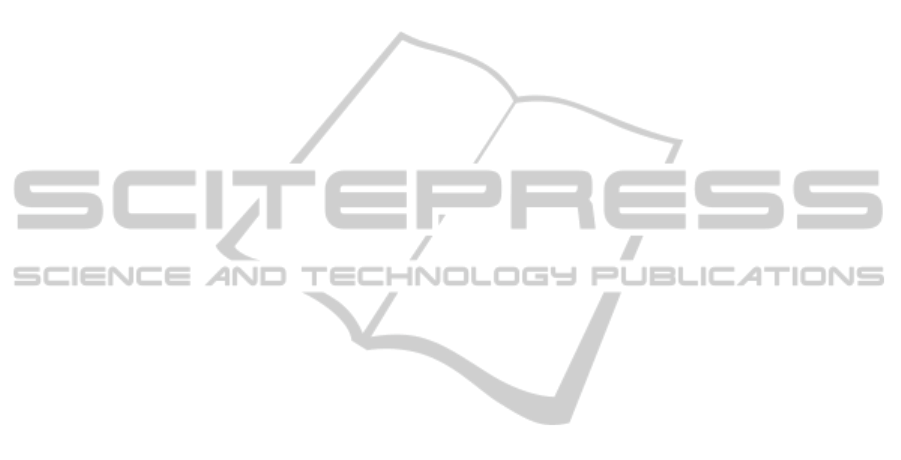
set, highest Classification Accuracy achieved by pro-
posed technique is 94.32 ± 3.52 on m-circle value 8
with Computational Time 6.54±0.10 (s). Instead,
it attains 92.26 ± 4.50 as the minimum Classifica-
tion Accuracy with Computational Time 6.87±0.11
(s) on m-circle value 2. On the other hand, method
given by (Mansouri et al., 2008) attains 85.42 ±
0.55 as maximum Classification Accuracy for m-
circle values {64,...,1024} with Computational Time
varies from {7.11±0.06,..,7.15±0.1} (s) whereas it
gives the minimum Classification Accuracy 84.70 ±
1.00 on m-circle value 2 with Computational Time
7.63±0.17 (s). On the contrary, the method pro-
posed by (Bandyopadhyay, 2005) achieves the best
Classification Accuracy rate 67.51 ± 8.38 on m-
circle values {16,...,1024} with Computational Time
from {10.13±0.09,..,10.20±0.13} (s). Although, it
gives the worst Classification Accuracy rate 67.34 ±
8.30 for m-circle value 2 with Computational Time
10.60±0.18 (s). The other method developed by
(Wang et al., 2001), exhibits 51.41 ± 0.27 as mini-
mum and maximum Classification Accuracy rate for
all the values of m-circle with Computational Time
varies from {63.39±1.20,..,63.98±1.26}(s). More-
over, exhaustive results reported in Table 5, jus-
tify the significance of proposed approach due to
the improvements in Classification Accuracy rate as
well as in Computational Time when compared with
the methods proposed by (Mansouri et al., 2008),
(Bandyopadhyay, 2005), (Wang et al., 2001).
4 CONCLUSIONS
In this paper, a novel feature extraction approach is
proposed for classifying the protein sequences into
the superfamilies. The proposed approach compute
both the local and global similarity measures for ex-
tracting relevant features corresponding to each pro-
tein sequence. The global similarity measure is cal-
culated by considering probability of occurrence of
the positional variance of each amino acid among all
the sequences within the superfamily. However, the
local similarity measure is produced by evaluating a
weighting scheme (Karchin and Hughey, 1998) of the
global probability and then assigns the weighted prob-
ability of each amino acid to the six exchange groups
(Dayhoff and Schwartz, 1978). Finally, the 6 features
are extracted corresponding to each protein sequence
which is classified using Boolean-Like Training Al-
gorithm (BLTA) (Gray and Michel, 1992).
The experimental work is carried out on two su-
perfamilies Ras and Globin to probe the efficacy of
the proposed approach on BLTA classifier in compar-
ison with other approaches (Mansouri et al., 2008),
(Bandyopadhyay, 2005), (Wang et al., 2001). More-
over, the results are analyzed and reported in terms of
four parameters-Mean, Standard Deviation, Classifi-
cation Accuracy and Computational Time with vari-
ation in m-circle values of BLTA classifier. The ob-
servation can be drawn from the experimental re-
sults, that the proposed approach extract very limited
number of features in comparison with other meth-
ods. Therefore, it outperforms on the BLTA classi-
fier and thus, achieves best Classification Accuracy
94.32 ± 3.52 with Computational Time 6.54±0.10 (s)
on m-circle value 8. Hence, its performance is much
higher in comparison to other methods (Mansouri
et al., 2008), (Bandyopadhyay, 2005), (Wang et al.,
2001) in terms of Classification Accuracy and Com-
putational Time.
REFERENCES
Bandyopadhyay, S. (2005). An efficient technique for su-
perfamily classification of amino acid sequences: fea-
ture extraction, fuzzy clustering and prototype selec-
tion. Fuzzy Sets and Systems, 152(1):5–16.
Barker, W., Garavelli, J., Huang, H., McGarvey, P., Orcutt,
B., G.Y.Srinivasarao, Xiao, C., Yeh, L., Ledley, R.,
Janda, J., F.Pfeiffer, H.W.Mewes, A. T., and Wu, C.
(2004). The protein information resource (pir). Nu-
cleic Acids Research, 28(1):41–44.
Dayhoff, M. and Schwartz, R. (1978). A model of evo-
lutionary change in proteins. In In Atlas of protein
sequence and structure. Citeseer.
Gray, D. and Michel, A. (1992). A training algorithm for bi-
nary feedforward neural networks. Neural Networks,
IEEE Transactions on, 3(2):176–194.
Iqbal, M. J., Faye, I., Samir, B. B., and Said, A. M. (2014).
Efficient feature selection and classification of protein
sequence data in bioinformatics. The Scientific World
Journal, 2014.
Karchin, R. and Hughey, R. (1998). Weighting hidden
markov models for maximum discrimination. Bioin-
formatics, 14(9):772–782.
Mansouri, E., A.M. Zou, S. Katebi, H. M. R. B., and Sadr,
A. (2008). Generating fuzzy rules for protein classifi-
cation. Iranian Journal of Fuzzy Systems.
Solovyov, A. and Lipkin, W. I. (2013). Centroid based clus-
tering of high throughput sequencing reads based on
n-mer counts. BMC bioinformatics, 14(1):268.
Vergara, J. R. and Est
´
evez, P. A. (2014). A review of fea-
ture selection methods based on mutual information.
Neural Computing and Applications, 24(1):175–186.
Vipsita, S. and Rath, S. K. (2013). Two-stage approach for
protein superfamily classification. Computational Bi-
ology Journal, 2013.
Wang, J., Ma, Q., Shasha, D., and Wu, C. (2001). New tech-
niques for extracting features from protein sequences.
IBM Systems Journal, 40(2):426–441.
BIOINFORMATICS2015-InternationalConferenceonBioinformaticsModels,MethodsandAlgorithms
224