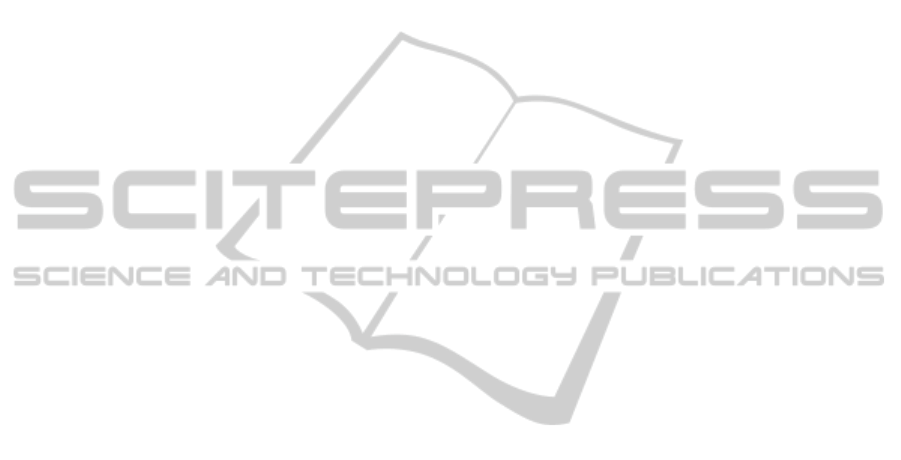
Maruani, T. and Amit-Cohen, I. (2007). Open space plan-
ning models: A review of approaches and methods.
Landscape and Urban Planning, 81(12):1 – 13.
Matthews, R. B., Gilbert, N. G., Roach, A., Polhill, J. G.,
and Gotts, N. M. (2007). Agent-based land-use mod-
els: a review of applications. Landscape Ecology,
22(10):1447–1459.
McCormack, G. R., Giles-Corti, B., Bulsara, M., and
Pikora, T. J. (2006). Correlates of distances traveled
to use recreational facilities for physical activity be-
haviors. International Journal of Behavioral Nutrition
and Physical Activity, 3(1):18.
McCormack, G. R., Rock, M., Toohey, A. M., and Hignell,
D. (2010). Characteristics of urban parks associated
with park use and physical activity: a review of quali-
tative research. Health & place, 16(4):712–726.
McPherson, E. G. (2006). Urban forestry in north america.
Renewable Resources Journal, 24(3):8–12.
Morancho, A. B. (2003). A hedonic valuation of ur-
ban green areas. Landscape and urban planning,
66(1):35–41.
Murray-Rust, D., Rieser, V., Robinson, D. T., Milii, V.,
and Rounsevell, M. (2013). Agent-based modelling of
land use dynamics and residential quality of life for fu-
ture scenarios. Environmental Modelling & Software,
46(0):75 – 89.
Nelson, E., Mendoza, G., Regetz, J., Polasky, S., Tallis, H.,
Cameron, D., Chan, K. M., Daily, G. C., Goldstein,
J., Kareiva, P. M., et al. (2009). Modeling multiple
ecosystem services, biodiversity conservation, com-
modity production, and tradeoffs at landscape scales.
Frontiers in Ecology and the Environment, 7(1):4–11.
Payne, L. L., Mowen, A. J., and Orsega-Smith, E. (2002).
An examination of park preferences and behaviors
among urban residents: the role of residential loca-
tion, race, and age. Leisure sciences, 24(2):181–198.
Pham, T.-T.-H., Apparicio, P., S´eguin, A.-M., Landry, S.,
and Gagnon, M. (2012). Spatial distribution of veg-
etation in montreal: An uneven distribution or envi-
ronmental inequity? Landscape and Urban Planning,
107(3):214–224.
Pickett, S. T., Cadenasso, M., Grove, J., Nilon, C., Pouyat,
R., Zipperer, W., and Costanza, R. (2001). Urban eco-
logical systems: linking terrestrial ecological, physi-
cal, and socioeconomic components of metropolitan
areas. Annual review of ecology and systematics,
pages 127–157.
Pouta, E. and Heikkil¨a, M. (1998). Virkistysalueiden suun-
nittelu ja hoito. Ymp¨arist¨oministeri¨o, alueidenk¨ayt¨on
osasto.
Robinson, D., Murray-Rust, D., Rieser, V., Milicic, V., and
Rounsevell, M. (2012). Modelling the impacts of
land system dynamics on human well-being: Using an
agent-based approach to cope with data limitations in
koper, slovenia. Computers, Environment and Urban
Systems. Special Issue: Geoinformatics 2010, 36(Is-
sue 2):164–176.
Roovers, P., Hermy, M., and Gulinck, H. (2002). Visitor
profile, perceptions and expectations in forests from a
gradient of increasing urbanisation in central belgium.
Landscape and Urban Planning, 59(3):129–145.
Schroeder, H. W. and Daniel, T. (1982). Preferred features
of urban parks and forests. Journal of Arboriculture,
8(12):317–322.
Shelby, B., Bregenzer, N. S., Johnson, R., et al. (1988).
Displacement and product shift: empirical evidence
from oregon rivers. Journal of Leisure Research,
20(4):274–288.
Takayama, N., Korpela, K., Lee, J., Morikawa, T., Tsunet-
sugu, Y., Park, B.-J., Li, Q., Tyrv¨ainen, L., Miyazaki,
Y., and Kagawa, T. (2014). Emotional, restorative
and vitalizing effects of forest and urban environments
at four sites in japan. International Journal of Envi-
ronmental Research and Public Health, 11(7):7207–
7230.
Tarzia, V. (2003). European common indicators: towards a
local sustainability profile. Ambiente Italia Research
Institute, Milano, Italy.
Uy, P. D. and Nakagoshi, N. (2008). Application of
land suitability analysis and landscape ecology to ur-
ban greenspace planning in hanoi, vietnam. Urban
Forestry & Urban Greening, 7(1):25–40.
Vallejo, M., Corne, D., and Rieser, V. (2014). Evolving op-
timal spatial allocation policies for complex and un-
certain environments. In Filipe, J. and Fred, A., ed-
itors, Agents and Artificial Intelligence, volume 449
of Communications in Computer and Information Sci-
ence, pages 351–369. Springer Berlin Heidelberg.
Vallejo, M., Corne, D. W., and Rieser, V. (2013). Evolving
urbanisation policies - using a statistical model to ac-
celerate optimisation over agent-based simulations. In
ICAART 2013 - 5th Conference on Agents and Artifi-
cial Intelligence, pages 1–11.
Vaske, J. J., Donnelly, M. P., and Heberlein, T. A. (1980).
Perceptions of crowding and resource quality by early
and more recent visitors. Leisure Sciences, 3(4):367–
381.
Watts, M. E., Ball, I. R., Stewart, R. S., Klein, C. J., Wil-
son, K., Steinback, C., Lourival, R., Kircher, L., and
Possingham, H. P. (2009). Marxan with zones: soft-
ware for optimal conservation based land-and sea-
use zoning. Environmental Modelling & Software,
24(12):1513–1521.
Williams, J. and Lawson, R. (2001). Community issues and
resident opinions of tourism. Annals of Tourism Re-
search, 28(2):269–290.
Wong, K. K. (2009). Urban park visiting habits and leisure
activities of residents in hong kong, china. Managing
Leisure, 14(2):125–140.
Woolley, H. (2003). Urban open spaces. Taylor & Francis.
Zucca, A., Sharifi, A. M., and Fabbri, A. G. (2008). Appli-
cation of spatial multi-criteria analysis to site selec-
tion for a local park: A case study in the bergamo
province, italy. Journal of Environmental Manage-
ment, 88(4):752–769.
ICAART2015-InternationalConferenceonAgentsandArtificialIntelligence
262