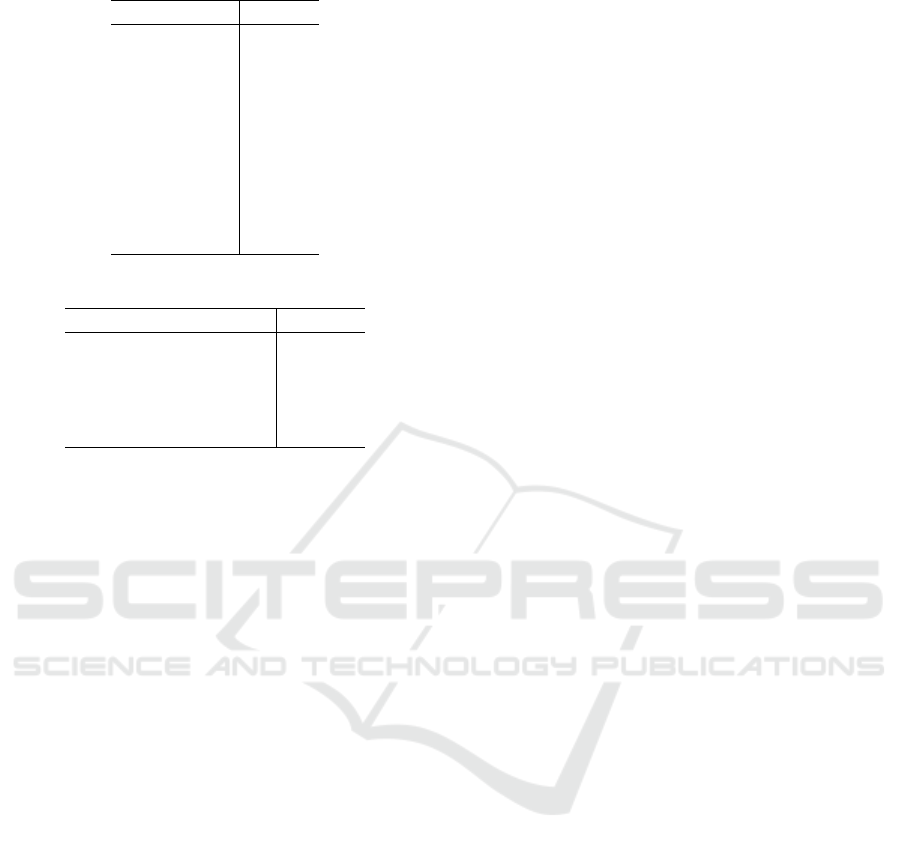
Table 2: 2D-case.
n
1
n
2
n
3
Error
1 2 3 2.5394
2 3 9 2.7503
2 3 8 2.7539
2 3 5 2.7595
2 3 10 2.7647
2 3 6 2.7662
2 3 7 2.7682
2 3 4 2.7697
1 2 5 2.7754
1 2 6 2.8143
Table 3: r-variation.
n
1
n
2
n
3
n
4
n
5
Error
3 2.95372
2 3 2.74907
1 2 3 2.56917
1 2 3 4 2.57371
1 2 3 5 9 2.56517
There is no formal rule for the choice of optimal r
(the size of significant collection). But it seems quite
natural to stop increase r if it doesn’t decrease the
estimation of the error. In the Table 3 one can find
the results for another one application of our method
to simulated data. In this table estimations of Err
for different r are listed. It is not difficult to con-
clude that the error does not decrease when r exceeds
3 (the number of significant factors). Besides, one
more reason to choose the r = 3 is that for r = 4 the
gap between the first and the second collections is just
0.0003 (the error of collection (X
1
,X
2
,X
3
,X
4
) equals
2.57371 and the error of collection (X
1
,X
2
,X
3
,X
6
) is
equal to 2.57396). It means that factors X
6
and X
4
are
not in strong association with the trait in contrast to
the factors X
1
, X
2
, X
3
.
4 CONCLUSIONS
In this paper we studied the problem of identifica-
tion of the collection of significant factors determin-
ing some disordered complex trait. We introduced
two models for the set of possible values of response
variable and developed multifactorial dimensionality
reduction approach based on estimation of error func-
tion. Using simulated data we demostrated the ef-
ficiency of our method. Further research remains a
comparison our algorithm with other methods of di-
mensionality reduction (e.g., Discriminant Principal
Component Analysis).
ACKNOWLEDGEMENTS
This work is partially supported by RSF grant 14-21-
00162.
REFERENCES
Adams, H. P., Bendixen, B. H., Kappelle, L. J., Biller, J.,
Love, B. B., Gordon, D. L., and Marsh (1993). Clas-
sification of subtype of acute ischemic stroke. Defi-
nitions for use in a multicenter clinical trial. TOAST.
Trial of Org 10172 in Acute Stroke Treatment. Stroke,
24(1):35–41.
Arlot, S. and Celisse, A. (2010). A survey of cross-
validation procedures for model selection. Statistics
Surveys, 4:40–79.
Bulinski, A. (2014). On foundation of the dimensionality
reduction method for explanatory variables. Journal
of Mathematical Sciences, 199(2):113–122.
Bulinski, A. and Rakitko, A. (2014). Estimation of
nonbinary random response. Doklady Mathematics,
89(2):225–229.
Hastie, T., Tibshirani, R., and Friedman, J. (2001). The
Elements of Statistical Learning. Springer Series in
Statistics. Springer New York Inc., New York, NY,
USA.
Iman, R. L. and Conover, W. J. (1982). A distribution-free
approach to inducing rank correlation among input
variables. Communications in Statistics - Simulation
and Computation, 11(3):311–334.
Lee, S., Epstein, M. P., Duncan, R., and Lin, X. (2012).
Sparse principal component analysis for identifying
ancestry-informative markers in genome-wide associ-
ation studies. Genetic Epidemiology, 36(4):293–302.
Ritchie, M. D., Hahn, L. W., Roodi, N., Bailey, L. R.,
Dupont, W. D., Parl, F. F., and Moore, J. H.
(2001). Multifactor-dimensionality reduction reveals
high-order interactions among estrogen-metabolism
genes in sporadic breast cancer. The American Jour-
nal of Human Genetics, 69(1):138 – 147.
Ruczinski, I., Kooperberg, C., and LeBlanc, M. (2003).
Logic regression. Journal of Computational and
Graphical Statistics, 12(3):475–511.
Sikorska, K., Lesaffre, E., Groenen, P. F. J., and Eilers, P.
H. C. (2013). Gwas on your notebook: fast semi-
parallel linear and logistic regression for genome-
wide association studies. BMC Bioinformatics, pages
166–166.
Tibshirani, R. J. and Taylor, J. (2012). Degrees of free-
dom in lasso problems. The Annals of Statistics,
40(2):1198–1232.
Velez, D. R., White, B. C., Motsinger, A. A., Bush, W. S.,
Ritchie, M. D., Williams, S. M., and Moore, J. H.
(2007). A balanced accuracy function for epista-
sis modeling in imbalanced datasets using multifac-
tor dimensionality reduction. Genetic Epidemiology,
31(4):306–315.
BIOINFORMATICS 2015 - International Conference on Bioinformatics Models, Methods and Algorithms
236