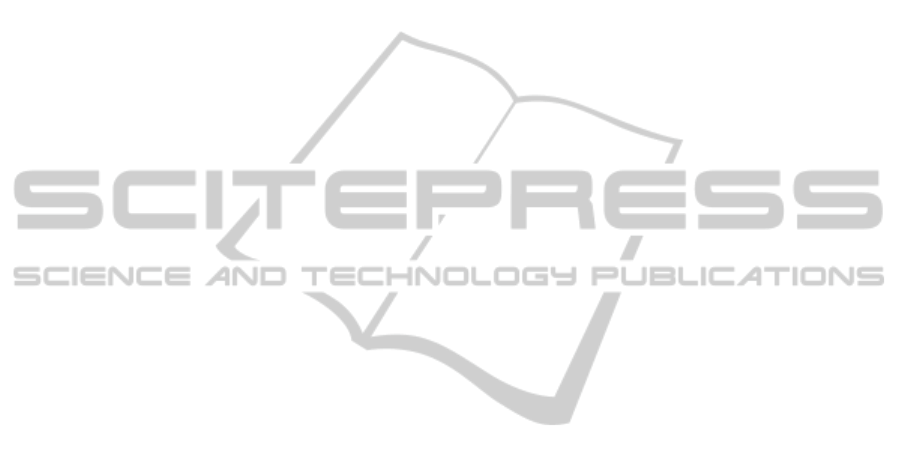
6 CONCLUSIONS
The proposed simulator can estimate the postopera-
tive knee flexion at initial contact, given the preopera-
tive gait, physical examination and a surgery. On test,
63.21% of the N = 193 limbs are estimated with an
acceptable regression error (see section 5). In addi-
tion, the mean RMSE is 9.25
◦
, which means that the
expected error of regression is also acceptable (infe-
rior to 10
◦
).
The developed system is able to give good estima-
tions independently of the preoperative gait parame-
ters and the type of surgery. However, around a third
of the patients are not well estimated. For the applica-
tion, it is important to apply surgery only if a good re-
sult can be asserted. Conversely, it is crucial to avoid
a surgery plan if a bad outcome is most likely. For
this reason, it would be interesting to detect a priori
patients that will be badly estimated. With this strat-
egy, patients more likely to be badly estimated, could
be rejected by the simulator. For example, if a new
patient is too far from the training patients in the in-
put space, no estimation will be given.
In order to improve estimation, a prior cluster-
ing of the patients could be applied, so the regression
method would be able to adapt to the type of subject.
Finally, this study predicts the outcomes of or-
thopaedic surgery with real gait parameters, and not
with qualitative parameters that are too relative and
ambiguous for such a sensitive application.
Further work will focus on estimating the whole
postoperative knee flexion gait cycle signal and not
only the initial contact point.
ACKNOWLEDGEMENTS
This work is part of a project funded by the Fondation
Ellen Poidatz, the Fondation Bettencourt Schueller
and the Region Ile de France. The authors would like
to thank the UNAM medical and technical team of the
Fondation Ellen Poidatz, who recorded all data used
in this work.
REFERENCES
Walking in graphs. http://www.utdallas.edu. Accessed:
2014-10-09.
Armand, S., Watelain, E., Roux, E., Mercier, M., and Lep-
outre, F.-X. (2007). Linking clinical measurements
and kinematic gait patterns of toe-walking using fuzzy
decision trees. Gait & Posture, 25(3):475–484.
Arnold, A. S., Liu, M. Q., Schwartz, M. H., Õunpuu, S.,
and Delp, S. L. (2006). The role of estimating muscle-
tendon lengths and velocities of the hamstrings in the
evaluation and treatment of crouch gait. Gait & Pos-
ture, 23(3):273–281.
Bishop, C. (2006). Pattern Recognition and Ma-
chine Learning. Information Science and Statistics.
Springer.
Charlton, I., Tate, P., Smyth, P., and Roren, L. (2004). Re-
peatability of an optimised lower body model. Gait &
Posture, 20(2):213–221.
Desailly, E. (2008). Analyse biomécanique 3D de la marche
de l’enfant déficient moteur. Modélisation segmentaire
et modélisation musculo-squelettique. PhD thesis,
Université de Poitiers.
Dreyfus, G., Martinez, J.-M., Samuelides, M., Gordon, M.,
Badran, F., and Thiria, S. (2008). Apprentissage statis-
tique. Eyrolles, Paris, 3rd edition.
Duda, R., Hart, P., and Stork, D. (2001). Pattern Classifica-
tion. Wiley-Interscience, 2nd edition.
Gage, J., Schwartz, M., Koop, S., and Novacheck, T., edi-
tors (2009). The Identification and Treatment of Gait
Problems in Cerebral Palsy. Mac Keith Press, 2nd
edition.
Groen, B., Geurts, M., Nienhuis, B., and Duysens, J.
(2012). Sensitivity of the OLGA and VCM models to
erroneous marker placement: Effects on 3d-gait kine-
matics. Gait & Posture, 35(3):517–521.
Guyon, I. and Elisseeff, A. (2003). An introduction to vari-
able and feature selection. The Journal of Machine
Learning Research, 3:1157–1182.
Ma, F. Y. P., Selber, P., Nattrass, G. R., Harvey, A. R.,
Wolfe, R., and Graham, H. K. (2006). Length-
ening and transfer of hamstrings for a flexion de-
formity of the knee in children with bilateral cere-
bral palsy TECHNIQUE AND PRELIMINARY RE-
SULTS. Journal of Bone & Joint Surgery, British Vol-
ume, 88(2):248–254.
Noonan, K. J., Halliday, S., Browne, R., O’Brien, S., Kayes,
K., and Feinberg, J. (2003). Interobserver variability
of gait analysis in patients with cerebral palsy. Journal
of Pediatric Orthopaedics, 23(3):279–287.
Reinbolt, J. A., Fox, M. D., Schwartz, M. H., and Delp,
S. L. (2009). Predicting outcomes of rectus femoris
transfer surgery. Gait & Posture, 30(1):100–105.
Sebsadji, A., Khouri, N., Djemal, K., Yepremian, D., Hareb,
F., Hoppenot, P., and Desailly, E. (2012). Description
and classification of the effect of hamstrings lengthen-
ing in cerebral palsy children multi-site surgery. Com-
puter Methods in Biomechanics and Biomedical Engi-
neering, 15(sup1):177–179.
Steinwender, G., Saraph, V., Scheiber, S., Zwick, E. B.,
Uitz, C., and Hackl, K. (2000). Intrasubject repeata-
bility of gait analysis data in normal and spastic chil-
dren. Clinical Biomechanics, 15(2):134–139.
ICPRAM2015-InternationalConferenceonPatternRecognitionApplicationsandMethods
342