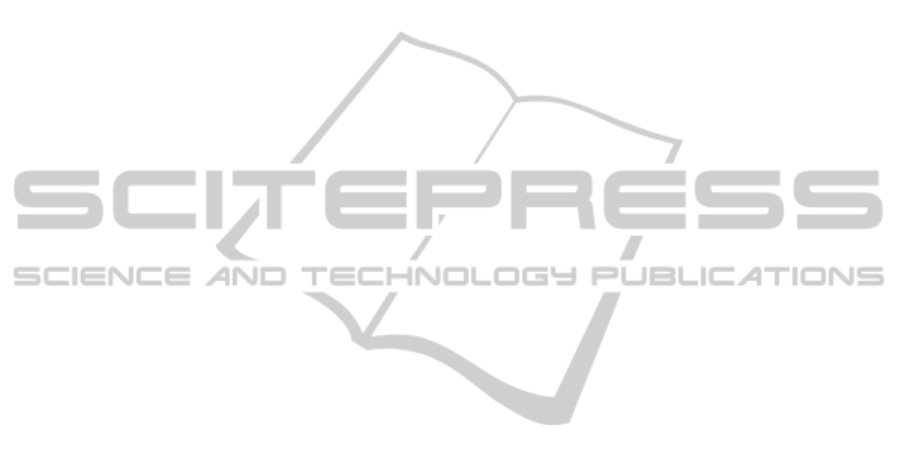
Thus, the prediction models obtained can be used
for supporting decision-making process of the
nursing team responsible for the VIP process.
Nurses can take into account a priori the
characteristics that identify a woman who belongs to
the risk group, getting an idea if the patient is a
possible “member” of this group, and consequently
take preventive measures to these patients.
6 CONCLUSION AND FUTURE
WORK
This study demonstrated that it is possible to obtain
DM classification models to predict which patient
belongs to the risk group in the VIP process. The
study was conducted using real data inherent to the
VIP process, corresponding to 1 year of activity in
CMIN.
Good results were achieved in terms of
sensitivity, being approximately 93%, in the model 4
using Support Vector Machines. The most relevant
factors to determine the risk group are the patient's
age, the number of previous VIPs, the number of
previous pregnancies (Gesta) and the number of
previous births (Para). It can be concluded that, with
the use of classification techniques applied to
historical data from pregnant women who underwent
in the process of VIP, can be predicted if a patient
fits the features of a VIP risk group.
The results are important for a possible redesign
of the VIP protocol implemented on CMIN. These
models allow improving the service, increasing the
number of successes and decrease the costs. At same
time can be applied to other institutions with the
same problem / protocols.
According to the health professionals these
models are very interesting and useful to support the
decision when a women wants to make a VIP.
This type of research can promote future
developments related with this particular area of
maternity care.
ACKNOWLEDGEMENTS
”This work is funded by National Funds through the
FCT – Fundação para a Ciência e a Tecnologia
(Portuguese Foundation for Science and
Technology) within project PEstOE/EEI/UI0752/
2014 and PEst-OE/EEI/UI0319/2014”.
REFERENCES
Abelha, A., Analide, C., Machado, J., Neves, J., & Novais,
P. (2007). Ambient Intelligence and Simulation in
Health Care Virtual Scenarios, 243, 461–468.
Bonney, W. (2013). Applicability of Business Intelligence
in Electronic Health Record. Procedia - Social and
Behavioral Sciences, 73, 257–262.
doi:10.1016/j.sbspro.2013.02.050.
Catley, C., Frize, M., Walker, C. R., & Petriu, D. C.
(2006). Predicting High-Risk Preterm Birth Using
Artificial Neural Networks. IEEE Transactions on
Information Technology in Biomedicine, 10(3), 540–
549. doi:10.1109/TITB.2006.872069.
Chapman, P., Clinton, J., Kerber, R., Khabaza, T.,
Reinartz, T., Shearer, C., & Wirth, R. (2000). The
CRISP-DM User Guide. NCR Systems Engineering
Compenhagen. Brussels: NCR Systems Engineering
Copenhagen.
Chattopadhyay, S., Ray, P., Chen, H. S., Lee, M. B., &
Chiang, H. C. (2008). Suicidal Risk Evaluation Using
a Similarity-Based Classifier. In C. Tang, C. Ling, X.
Zhou, N. Cercone, & X. Li (Eds.), Advanced Data
Mining and Applications SE - 7 (Vol. 5139, pp. 51–
61). Springer Berlin Heidelberg. doi:10.1007/978-3-
540-88192-6_7.
Cios, K., Pedrycz, W., Swiniarski, R., & Kurgan, L.
(2007). Data Mining. A knowledge Discovery
Approach. Springer.
Goebel, R., Siekmann, J., & Wahlster, W. (2008).
Advances in Knowledge Discovery and Data Mining.
Springer.
Gonçalves, J., Portela, F., Santos, M. F., Silva, Á.,
Machado, J., Abelha, A., & Rua, F. (2013). Real-time
Predictive Analytics for Sepsis Level and Therapeutic
Plans in Intensive Care Medicine. International
Information Institute.
O’Sullivan, D., Elazmeh, W., Wilk, S., Farion, K.,
Matwin, S., Michalowski, W., & Sehatkar, M. (2008).
Using Secondary Knowledge to Support Decision
Tree Classification of Retrospective Clinical Data. In
Z. Raś, S. Tsumoto, & D. Zighed (Eds.), Mining
Complex Data SE - 19 (Vol. 4944, pp. 238–251).
Springer Berlin Heidelberg. doi:10.1007/978-3-540-
68416-9_19.
Palaniappan, S., & Awang, R. (2008). Intelligent Heart
Disease Prediction System Using Data Mining
Techniques. In Proceedings of the 2008 IEEE/ACS
International Conference on Computer Systems and
Applications (pp. 108–115). Washington, DC, USA:
IEEE Computer Society.
doi:10.1109/AICCSA.2008.4493524.
Peixoto, H., Santos, M., Abelha, A., & Machado, J.
(2012). Intelligence in Interoperability with AIDA. In
L. Chen, A. Felfernig, J. Liu, & Z. Raś (Eds.), Lecture
Notes in Computer Science, Foundations of Intelligent
Systems - 31 (Vol. 7661, pp. 264–273). Springer
Berlin Heidelberg. doi:10.1007/978-3-642-34624-
8_31.
ICAART2015-InternationalConferenceonAgentsandArtificialIntelligence
600