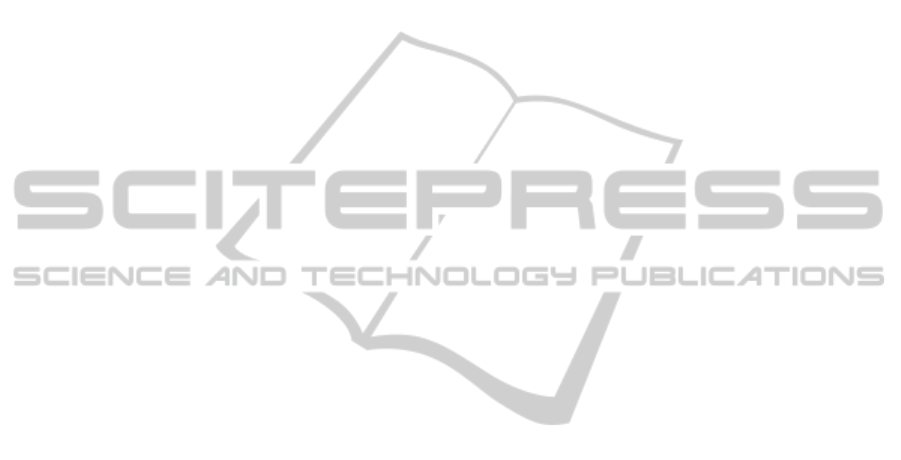
4 CONCLUSION AND PROSPECTS
We have defined quantitative measures for qualitative
concepts of depth, difficulty, complexity, and discrim-
ination. The definitions are within a specific model of
decision making at chess, but use no feature of chess
apart from utility values of decision options, and are
framed via mathematical tools that work across ap-
plication areas. For the first three, we have shown
a strong response effect on performance, though we
have not distinguished the measures from each other.
The effect shows across skill levels and persists when
restricting to controlled cases such as moves of equal
highest-depth value.
REFERENCES
Andersen, E. (1973). Conditional inference for multiple-
choice questionnaires. Brit. J. Math. Stat. Psych.,
26:31–44.
Anderson, L. and Krathwol, D. (2001). A Taxonomy for
Learning, Teaching, and Assessing: A revision of
Blooms taxonomy of educational objectives: complete
edition. Longman, New York.
Andrich, D. (1978). A rating scale formulation for ordered
response categories. Psychometrika, 43:561–573.
Andrich, D. (1988). Rasch Models for Measurement. Sage
Publications, Beverly Hills, California.
Baker, F. B. (2001). The Basics of Item Response Theory.
ERIC Clearinghouse on Assessment and Evaluation.
Bloom, B. (1956). Taxonomy of Educational Objectives,
Handbook I: The Cognitive Domain. David McKay
Co., New York.
Bransford, J. D., Brown, A., and Cocking, R., editors
(2000). How People Learn: expanded edition. The
National Academies Press, Washington, D.C.
Busemeyer, J. R. and Townsend, J. T. (1993). Decision
field theory: a dynamic-cognitive approach to deci-
sion making in an uncertain environment. Psycholog-
ical review, 100(3):432.
Chabris, C. and Hearst, E. (2003). Visualization, pattern
recognition, and forward search: Effects of playing
speed and sight of the position on grandmaster chess
errors. Cognitive Science, 27:637–648.
Donovan, M. S. and Bransford, J. D. (2005). How Students
Learn. The National Academies Press, Washington,
D.C.
Elo, A. (1978). The Rating of Chessplayers, Past and
Present. Arco Pub., New York.
Ferreira, D. (2013). The impact of search depth on chess
playing strength. ICGA Journal, 36(2):67–80.
Glickman, M. E. (1999). Parameter estimation in large dy-
namic paired comparison experiments. Applied Statis-
tics, 48:377–394.
Guid, M. and Bratko, I. (2006). Computer analysis of world
chess champions. ICGA Journal, 29(2):65–73.
Guid, M. and Bratko, I. (2011). Using heuristic-search
based engines for estimating human skill at chess.
ICGA Journal, 34(2):71–81.
Haworth, G. (2003). Reference fallible endgame play.
ICGA Journal, 26:81–91.
Hotiu, A. (2006). The relationship between item difficulty
and discrimination indices in multiple-choice tests in
a physical science course. M.Sc. thesis.
Krathwohl, D., Bloom, B., and Bertram, B. (1973). Taxon-
omy of Educational Objectives, the Classification of
Educational Goals. Handbook II: Affective Domain.
David McKay Co., New York.
Linacre, J. M. (2006). Rasch analysis of rank-ordered data.
Journal of Applied Measurement, 7(1).
Masters, G. (1982). A Rasch model for partial credit scor-
ing. Psychometrika, 47:149–174.
Morris, G. A., Branum-Martin, L., Harshman, N., Baker,
S. D., Mazur, E., Dutta, S., Mzoughi, T., and Mc-
Cauley, V. (2006). Testing the test: Item response
curves and test quality. American Journal of Physics,
74(5):449–453.
Moxley, J. H., Ericsson, K. A., Charness, N., and Krampe,
R. T. (2012). The role of intuition and deliberative
thinking in experts’ superior tactical decision-making.
Cognition, 124(1):72 – 78.
Ostini, R. and Nering, M. (2006). Polytomous Item Re-
sponse Theory Models. Sage Publications, Thousand
Oaks, California.
Rasch, G. (1961). On general laws and the meaning of
measurement in psychology. In Proceedings, Fourth
Berkeley Symposium on Mathematical Statistics and
Probability, pages 321–334. University of California
Press.
Regan, K. and Haworth, G. (2011). Intrinsic chess ratings.
In Proceedings of AAAI 2011, San Francisco.
Thorpe, G. L. and Favia, A. (2012). Data analysis using
item response theory methodology: An introduction
to selected programs and applications. Psychology
Faculty Scholarship, page 20.
Tversky, A. and Kahneman, D. (1992). Advances in
prospect theory: Cumulative representation of uncer-
tainty. Journal of Risk and Uncertainty, 5:297–323.
Webb, N. (1997). Criteria for Alignment of Expectations
and Assessments on Mathematics and Science Educa-
tion. Monograph No. 6. CCSSO, Washington, DC.
QuantifyingDepthandComplexityofThinkingandKnowledge
607