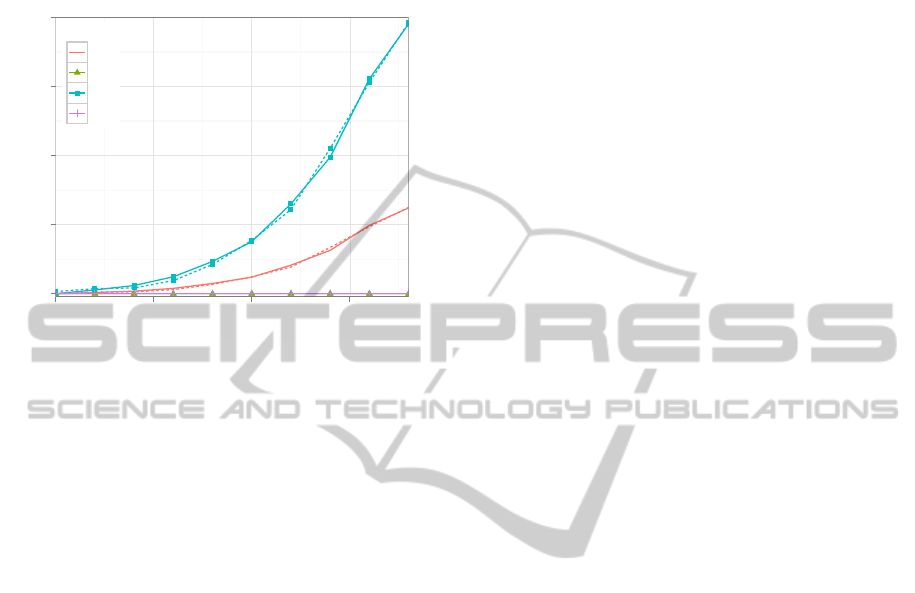
robust in terms of classification errors; both the FCR
and FAR are zero even with 90% missing data. This
is important in the discussed context as it ensures that
selective recycling lines are not contaminated.
0
25
50
75
100
0.00 0.25 0.50 0.75
Fraction of Missing Data
Error Percentage
Score
●
ERR
FCR
FRR
FAR
Figure 7: Recognition performance of the proposed
method, using ORB features. Continuous lines denote re-
sults obtained using grayscale images, while dashed lines
represent results obtained using opponent color space.
5 CONCLUSIONS
We have presented a method for recognizing specific
PCBs in waste streams via local feature matching and
geometric verification. The method achieves an open-
set recognition rate of up to 100% on a comprehen-
sive test dataset while being robust with respect to
broken PCBs. It is a key component in a recycling
appliance designed for reclaiming valuable chemical
elements and thus contributes to overcoming supply
bottlenecks and to sustainable electronics production.
Furthermore, we have performed a comprehen-
sive evaluation of local features in a new applica-
tion context, namely with respect to PCB recogni-
tion. The evaluation results show that ORB, BRISK,
FREAK, and AKAZE outperform SIFT and SURF
in this context. The differences between our find-
ings and previous results highlight the need for task-
specific test datasets. We contribute to the body of
available datasets by providing an extensive, freely
available dataset consisting of PCB images.
Moreover, we have demonstrated that utilizing
color information in the form of opponent color space
is beneficial not only to SIFT, but also to ORB,
BRISK, and FREAK.
REFERENCES
Aanæs, H., Dahl, A. L., and Pedersen, K. S. (2012). Inter-
esting interest points. International Journal of Com-
puter Vision, 97(1):18–35.
Alahi, A., Ortiz, R., and Vandergheynst, P. (2012). FREAK:
Fast Retina Keypoint. In IEEE Conference on Com-
puter Vision and Pattern Recognition, pages 510–517.
Alcantarilla, P. F., Nuevo, J., and Bartoli, A. (2013). Fast
Explicit Diffusion for Accelerated Features in Non-
linear Scale Spaces. In British Machine Vision Con-
ference, pages 13.1–13.11.
Bay, H., Tuytelaars, T., and Van Gool, L. (2006). SURF:
Speeded up robust features. In European Conference
on Computer Vision, pages 404–417. Springer.
Guerra, E. and Villalobos, J. (2001). A three-dimensional
automated visual inspection system for SMT assem-
bly. Computers & industrial engineering, 40(1):175–
190.
Heinly, J., Dunn, E., and Frahm, J.-M. (2012). Comparative
evaluation of binary features. In European Conference
on Computer Vision, pages 759–773. Springer.
Herchenbach, D., Li, W., and Breier, M. (2013). Segmen-
tation and classification of THCs on PCBAs. In IEEE
International Conference on Industrial Informatics,
pages 59–64.
Kalman, R. E. (1960). A New Approach to Linear Filtering
and Prediction Problems. Transactions of the ASME–
Journal of Basic Engineering, 82(D):35–45.
Koch, T., Breier, M., and Li, W. (2013). Heightmap genera-
tion for printed circuit boards (PCB) using laser trian-
gulation for pre-processing optimization in industrial
recycling applications. In IEEE International Confer-
ence on Industrial Informatics, pages 48–53.
Kuhn, H. W. (1955). The Hungarian method for the assign-
ment problem. Naval Research Logistics Quarterly,
2(1):83–97.
Leutenegger, S., Chli, M., and Siegwart, R. Y. (2011).
BRISK: Binary robust invariant scalable keypoints. In
IEEE International Conference on Computer Vision,
pages 2548–2555.
Li, W., Esders, B., and Breier, M. (2013). SMD segmen-
tation for automated PCB recycling. In IEEE Inter-
national Conference on Industrial Informatics, pages
65–70.
Lowe, D. G. (1999). Object recognition from local scale-
invariant features. In IEEE International Conference
on Computer Vision, volume 2, pages 1150–1157.
Lowe, D. G. (2004). Distinctive image features from scale-
invariant keypoints. International Journal of Com-
puter Vision, 60(2):91–110.
Mikolajczyk, K. and Schmid, C. (2005). A perfor-
mance evaluation of local descriptors. IEEE Trans-
actions on Pattern Analysis and Machine Intelligence,
27(10):1615–1630.
Mikolajczyk, K., Tuytelaars, T., Schmid, C., Zisserman, A.,
Matas, J., Schaffalitzky, F., Kadir, T., and Van Gool, L.
(2005). A comparison of affine region detectors. Inter-
national Journal of Computer Vision, 65(1-2):43–72.
PCBRecognitionusingLocalFeaturesforRecyclingPurposes
77