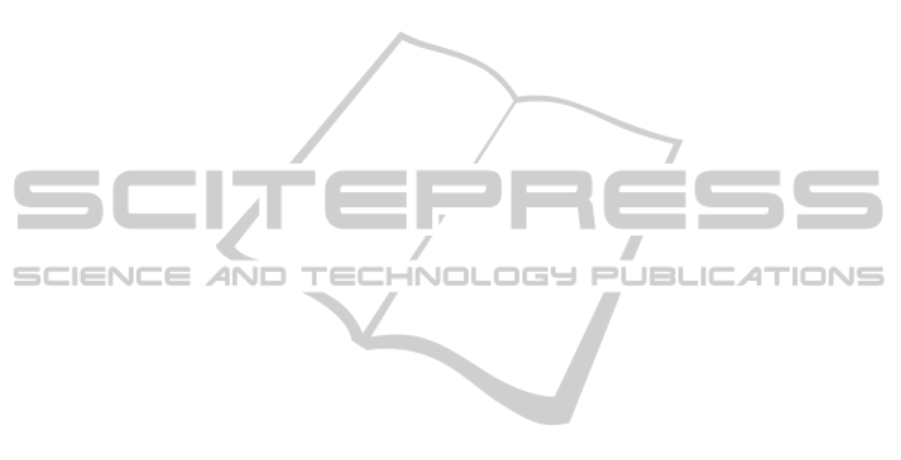
– For an inter-subject based approach I, the
single-lead dissimilarity array size is already
relatively large (T ∗ S
p
∗ L = 375). Increasing
it further does not contribute to the rise in in-
formation. Thus the EID degrades slightly.
• Lastly, the lowest EER for authentication origi-
nated from B
I,II,III
−EE. However it also presents
a high EID. The lowest EID is shown by I
I,II,III
−
CC which also gave a good and second lowest
EER value.
6 CONCLUSIONS AND FUTURE
WORK
The number of possible ECG representations is end-
less and so far none has managed to stand out at the
expense of all the others. In this paper a new ECG rep-
resentation space is developed and integrated into an
already existing biometric system. This feature space
is built through dissimilarity computation, where the
new features are a direct and pairwise comparison be-
tween those present in two signals, which here were
taken via metrics.
Moreover, the computation of this novel repre-
sentation can be extended to various types of ECG
configurations or signals, underlining its versatility.
The current study extended its usage to multi-lead
ECG signals, where an EER rate of 1.53% has been
achieved, for authentication over a database of 503
subjects as well as an EID rate of 5.65%. It should
be emphasized that for authentication/identification a
single heartbeat was used. The usage of a larger num-
ber of beats for classification will likely lead to better
results. When contrasting with the original technique,
which does not compute a dissimilarity representa-
tion, this feature space returns better results, proving
the usefulness of such a representation. However, as
in previous work, the usage of more than one lead did
not significantly improve results.
ACKNOWLEDGEMENTS
This work was partially funded by Fundac¸
˜
ao para a
Ci
ˆ
encia e Tecnologia (FCT) under grants PTDC/EEI-
SII/2312/2012, and SFRH/PROTEC/49512/2009,
whose support the authors gratefully acknowledge.
We would also like to thank Joana Santos for her hard
work labelling the ECG records.
REFERENCES
Agrafioti, F. and Hatzinakos, D. (2008). Fusion of ECG
sources for human identification. In Communications,
Control and Signal Processing, 2008. ISCCSP 2008.
3rd International Symposium on, pages 1542–1547.
Biel, L., Pettersson, O., Philipson, L., and Wide, P. (2001).
ECG analysis: a new approach in human identifica-
tion. Instrumentation and Measurement, IEEE Trans-
actions on, 50(3):808–812.
Carreiras, C., Lourenc¸o, A., Fred, A., and Ferreira, R.
(2014). ECG signals for biometric applications - are
we there yet? In 11th Int. Conf. on Informatics in
Control, Automation and Robotics, pages 765–772.
Scitepress.
Chan, A. D. C., Hamdy, M., Badre, A., and Badee, V.
(2008). Wavelet distance measure for person identifi-
cation using electrocardiograms. Instrumentation and
Measurement, IEEE Transactions on, 57(2):248–253.
Connell, S. D. and Jain, A. K. (1999). Template-based on-
line character recognition. Pattern Recognition, 34:1–
14.
Conover, M. (2003). Understanding Electrocardiography.
Mosby.
de Chazal, P., O’Dwyer, M., and Reilly, R. (2004). Auto-
matic classification of heartbeats using ECG morphol-
ogy and heartbeat interval features. Biomedical Engi-
neering, IEEE Transactions on, 51(7):1196–1206.
Duin, R., Loog, M., Pe¸kalska, E., and Tax, D. (2010).
Feature-based dissimilarity space classification. In
¨
Unay, D., C¸ taltepe, Z., and Aksoy, S., editors, Recog-
nizing Patterns in Signals, Speech, Images and Videos,
volume 6388 of Lecture Notes in Computer Science,
pages 46–55. Springer Berlin Heidelberg.
Duin, R. P. and Pe¸kalska, E. (2011). The dissimilarity
representation for structural pattern recognition. In
San Martin, C. and Kim, S.-W., editors, Progress in
Pattern Recognition, Image Analysis, Computer Vi-
sion, and Applications, volume 7042 of Lecture Notes
in Computer Science, pages 1–24. Springer Berlin
Heidelberg.
Duin, R. P. and Pe¸kalska, E. (2012). The dissimilar-
ity space: Bridging structural and statistical pattern
recognition. Pattern Recognition Letters, 33(7):826 –
832.
Fang, S.-C. and Chan, H.-L. (2009). Human identifi-
cation by quantifying similarity and dissimilarity in
electrocardiogram phase space. Pattern Recognition,
42(9):1824 – 1831.
Fatemian, S. and Hatzinakos, D. (2009). A new ECG fea-
ture extractor for biometric recognition. In Digital
Signal Processing, 2009 16th International Confer-
ence on, pages 1–6.
Friesen, G., Jannett, T., Jadallah, M., Yates, S., Quint, S.,
and Nagle, H. (1990). A comparison of the noise sen-
sitivity of nine qrs detection algorithms. Biomedical
Engineering, IEEE Transactions on, 37(1):85–98.
Hamilton, P. (2002). Open source ECG analysis. In Com-
puters in Cardiology, 2002, pages 101–104.
BIOSIGNALS2015-InternationalConferenceonBio-inspiredSystemsandSignalProcessing
358