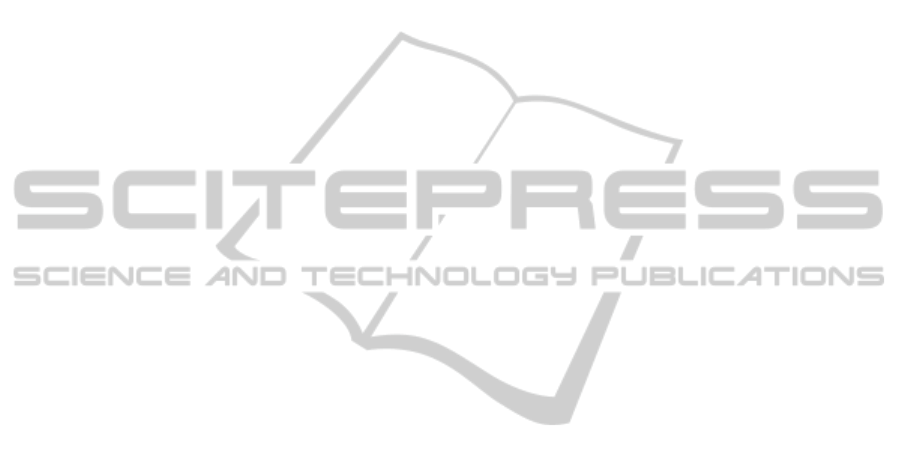
Belongie, S., Malik, J., and Puzicha, J. (2002). Shape
matching and object recognition using shape contexts.
IEEE Transactions on Pattern Analysis and Machine
Intelligence, 24(4):509–522.
Brochard, J., Khoudeir, M., and Augereau, B. (2001). In-
variant feature extraction for 3D texture analysis using
the autocorrelation function. Pattern Recognition Let-
ters, 22(6-7):759–768.
Cho, T. S., Avidan, S., and Freeman, W. T. (2010). The
patch transform. PAMI, 32(8):1489–1501.
Ciresan, D. C., Meier, U., and Schmidhuber, J. (2012).
Multi-column Deep Neural Networks for Image Clas-
sification. Proceedings of CVPR, pages 3642–3649.
Cortes, C. and Vapnik, V. (1995). Support-Vector Networks.
Machine Learning, 20(3):273–297.
DeCoste, D. and Sch
¨
olkopf, B. (2002). Training Invariant
Support Vector Machines. Machine Learning, 46(1–
3):161–190.
Deselaers, T., Keyser, D., and Ney, H. (2005). Discrim-
inative Training for Object Recognition using Image
Patches. Proceedings of CVPR, pages 157–162.
Dinu, L. P., Ionescu, R., and Popescu, M. (2012). Lo-
cal Patch Dissimilarity for Images. Proceedings of
ICONIP, 7663:117–126.
Efros, A. A. and Freeman, W. T. (2001). Image quilting for
texture synthesis and transfer. Proceedings of SIG-
GRAPH ’01, pages 341–346.
Guo, G. and Dyer, C. R. (2007). Patch-based Image Corre-
lation with Rapid Filtering. Proceedings of CVPR.
Horikawa, Y. (2004). Use of Autocorrelation Kernels in
Kernel Canonical Correlation Analysis for Texture
Classification. Proceedings of ICONIP, 3316:1235–
1240.
Horikawa-2004 (2004). Comparison of support vector ma-
chines with autocorrelation kernels for invariant tex-
ture classification. Proceedings of ICPR, 1:660–663.
Ionescu, R. T. and Popescu, M. (2014). PQ kernel: a rank
correlation kernel for visual word histograms. Pattern
Recognition Letters.
K
´
egl, B. and Busa-Fekete, R. (2009). Boosting Products of
Base Classifiers. Proceedings of ICML, pages 497–
504.
Keysers, D., Deselaers, T., Gollan, C., and Ney, H. (2007).
Deformation Models for Image Recognition. IEEE
Transactions on Pattern Analysis and Machine Intel-
ligence, 29(8):1422–1435.
LeCun, Y., Bottou, L., Bengio, Y., and Haffner, P. (1998).
Gradient-based learning applied to document recogni-
tion. Proceedings of the IEEE, 86(11):2278–2324.
LeCun, Y., Jackel, L. D., Boser, B., Denker, J. S., Graf,
H. P., Guyon, I., Henderson, D., Howard, R. E., and
Hubbard, W. (1989). Handwritten digit recognition:
Applications of neural net chips and automatic learn-
ing. IEEE Communications, pages 41–46.
Paredes, R., Prez-Cortes, J., Juan, A., and Vidal, E. (2001).
Local Representations and a Direct Voting Scheme for
Face Recognition. Proceedings of Workshop on Pat-
tern Recognition in Information Systems, pages 71–
79.
Popovici, V. and Thiran, J. (2001). Higher order autocorre-
lations for pattern classification. Proceedings of ICIP,
3:724–727.
Simard, P., LeCun, Y., Denker, J. S., and Victorri, B.
(1996). Transformation Invariance in Pattern Recog-
nition, Tangent Distance and Tangent Propagation.
Neural Networks: Tricks of the Trade.
Srihari, S. N. (1992). High-performance reading machines.
Proceedings of the IEEE (Special issue on Optical
Character Recognition), 80(7):1120–1132.
Suen, C. Y., Nadal, C., Legault, R., Mai, T. A., and Lam, L.
(1992). Computer recognition of unconstrained hand-
written numerals. Proceedings of the IEEE (Special
issue on Optical Character Recognition), 80(7):1162–
1180.
Teow, L.-N. and Loe, K.-F. (2002). Robust vision-
based features and classification schemes for off-line
handwritten digit recognition. Pattern Recognition,
35(11):2355–2364.
Toyoda, T. and Hasegawa, O. (2007). Extension of higher
order local autocorrelation features. Pattern Recogni-
tion, 40(5):1466–1473.
Wilder, K. J. (1998). Decision tree algorithms for hand-
written digit recognition. Electronic Doctoral Disser-
tations for UMass Amherst.
VISAPP2015-InternationalConferenceonComputerVisionTheoryandApplications
426