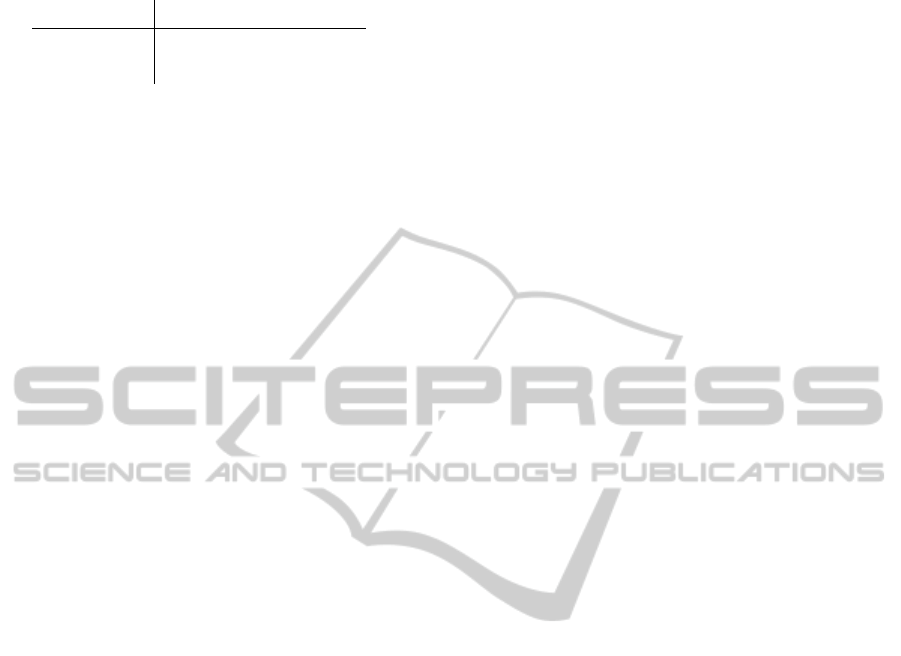
Table 3: Recognition rates R for dSIFT, GFD and FHD.
GFD dSIFT FHD
Peale 28.6 43.4 47.5
Motorbikes 65.7 50.9 70.9
with these two methods, compared to the best results
obtained with FHD descriptors. The results obtained
with both GFDs and dSIFTs are slightly lower than
the ones obtained with the proposed color FHD de-
scriptors, showing that FHDs are more suited to rec-
ognize complex structured objects where spatial orga-
nization is a discriminative feature.
4 DISCUSSION
This article has introduced a novel approach for ob-
ject recognition in color images. The main original-
ity of this approach lies in the extension of an object
descriptor using both shape and relative spatial infor-
mation about the subparts of the object to be recog-
nized. These subparts are obtained by considering a
content-based decomposition relying on the combina-
tion of an image segmentation algorithm and a clus-
tering algorithm. This decomposition strategy makes
it possible to deal with the problem raised by the tex-
tural properties of the objects in color images. Exper-
imental validations on two datasets have shown that
describing objects with such shape and spatial rela-
tions features between structural image layers yields
interesting recognition results.
Our work presents some limitations. From a
methodological point of view, the image decomposi-
tion approach could be affected by the clustering step,
which is based on color information. For instance, if
different subparts of an object share the same color,
it will lead to inconsistent image decompositions. In
addition, the number of resulting clusters has to be set
a priori and can be not adapted to the described ob-
ject. From an applicative point of view, the datasets
used were relatively small and present high intra-class
variance making it difficult to accurately evaluate the
behavior of our method.
In the future, we plan to enhance the FHD de-
scriptor by considering a multi-layer strategy where
the number of layers extracted per image may be au-
tomatically adapted depending on the image content.
We also plan to involve this framework into biomedi-
cal object recognition tasks where the object of inter-
est can be mixed up with the background.
ACKNOWLEDGEMENTS
This work was sponsored by the French National Re-
search Agency project SPIRIT #11-JCJC-008-01.
REFERENCES
Andreopoulos, A. and Tsotsos, J. K. (2013). 50 Years of
object recognition: Directions forward. Comput. Vis.
Image Und., 117(8):827–891.
Bloch, I. (2005). Fuzzy spatial relationships for image pro-
cessing and interpretation: A review. Image Vision
Comput., 23(2):89–110.
Bloch, I. and Ralescu, A. L. (2003). Directional relative po-
sition between objects in image processing: A com-
parison between fuzzy approaches. Pattern Recogn.,
36(7):1563–1582.
Bosch, A., Zisserman, A., and Muoz, X. (2006). Scene clas-
sification via pLSA. In Proc. of ECCV 2006, volume
3954 of LNCS, pages 517–530.
Comaniciu, D. and Meer, P. (2002). Mean shift: A robust
approach toward feature space analysis. IEEE Trans.
Pattern Anal. Mach. Intell., 24(5):603–619.
Delaye, A. and Anquetil, E. (2011). Fuzzy relative posi-
tioning templates for symbol recognition. In Proc. of
IEEE ICDAR 2011, pages 1220–1224.
Egenhofer, M. J. (1989). A formal definition of binary topo-
logical relationships. In Foundations of Data Organi-
zation and Algorithms, volume 367 of LNCS, pages
457–472.
Garnier, M., Hurtut, T., and Wendling, L. (2012). Object
description based on spatial relations between level-
sets. In Proc. of IEEE DICTA 2012, pages 1–7.
Inglada, J. and Michel, J. (2009). Qualitative spatial reason-
ing for high-resolution remote sensing image analysis.
IEEE Trans. Geosci. Remote Sens., 47(2):599–612.
Matsakis, P. and Wendling, L. (1999). A new way to repre-
sent the relative position between areal objects. IEEE
Trans. Pattern Anal. Mach. Intell., 21(7):634–643.
Morales-Gonz
´
alez, A. and Garc
´
ıa-Reyes, E. (2013). Sim-
ple object recognition based on spatial relations and
visual features represented using irregular pyramids.
Multimed. Tools Appl., 63(3):875–897.
Santosh, K., Lamiroy, B., and Wendling, L. (2012). Symbol
recognition using spatial relations. Pattern Recogn.
Lett., 33(3):331–341.
Zhang, D. and Lu, G. (2002). Shape-based image retrieval
using Generic Fourier Descriptor. Signal Process. Im-
age, 17(10):825–848.
VISAPP2015-InternationalConferenceonComputerVisionTheoryandApplications
434