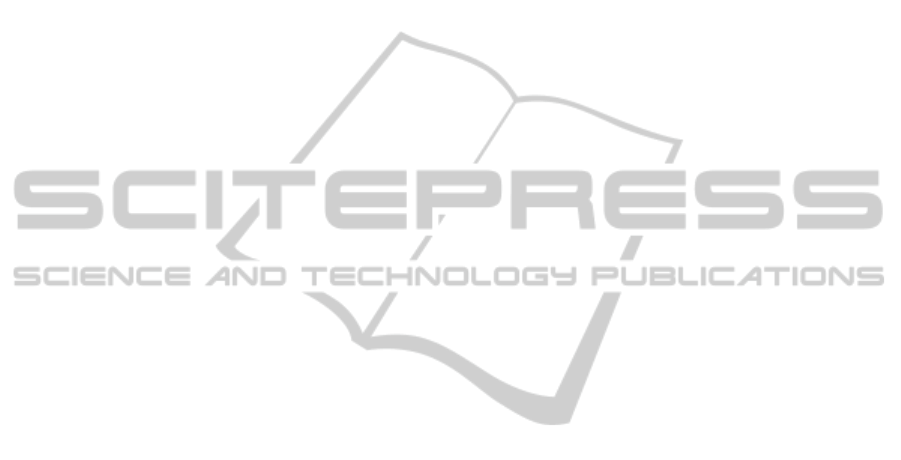
relevant situational awareness of topics and allows
them for serendipitous findings. These findings can
be easily added to existing or form new topic chan-
nels and therefore support the refinement of topics.
Future work includes an evaluation and a user
study of the visualization and to further extend the an-
alytical functionality. A possible idea would be to ad-
ditionally include an event detection mechanism and
automatically feed the resulting terms into the visual-
ization to create a large landscape of events and top-
ics.
A further improvement would be to extend the
system with a zooming feature to provide a more de-
tailed view to the users. This allows data to be dis-
played at different levels of granularity in order to get
deeper insights into the interesting points or periods in
time. For the topic channel definition, further options,
such as the source of the tweet (e.g., mobile phone or
web), the geographic region of the tweet or the type
of the tweet (e.g., retweet or direct message), could
be derived from the meta-data of tweets.
For a more powerful search and to improve the re-
sults, more full-text options, such as fuzzy search or
the exclusion of negative terms could be added. The
importance of the exclusion of negative terms can be
derived from the city observation case study, in which
the results shifted from tweets about the original topic
(reactions to the sport event Marathon) to a differ-
ent topic (reactions to the explosions) and therefore
it would be helpful to separate the topics from each
other.
REFERENCES
Abadi, D. J., Ahmad, Y., Balazinska, M., C¸ etintemel, U.,
Cherniack, M., Hwang, J., Lindner, W., Maskey, A.,
Rasin, A., Ryvkina, E., Tatbul, N., Xing, Y., and
Zdonik, S. B. (2005). The Design of the Borealis
Stream Processing Engine. In Proc. Intl. Conf. on In-
novative Data Systems Research (CIDR), pages 277–
289.
Abadi, D. J., Carney, D., C¸ etintemel, U., Cherniack, M.,
Convey, C., Lee, S., Stonebraker, M., Tatbul, N., and
Zdonik, S. (2003). Aurora: A New Model and Ar-
chitecture for Data Stream Management. The VLDB
Journal, 12(2):120–139.
Alvanaki, F., Michel, S., Ramamritham, K., and Weikum,
G. (2012). See what’s enblogue: real-time emer-
gent topic identification in social media. In Proceed-
ings of the 15th International Conference on Extend-
ing Database Technology, EDBT ’12, pages 336–347,
New York, NY, USA. ACM.
Arasu, A., Babu, S., and Widom, J. (2006). The CQL Con-
tinuous Query Language: Semantic Foundations and
Query Execution. The VLDB Journal, 15(2):121–142.
Bontcheva, K. and Rout, D. (2012). Making sense of so-
cial media streams through semantics: a survey. In
Semantic Web journal.
Bosch, H., Thom, D., Heimerl, F., Puttmann, E., Koch, S.,
Kr
¨
uger, R., W
¨
orner, M., and Ertl, T. (2013). Scat-
terblogs2: Real-time monitoring of microblog mes-
sages through user-guided filtering. IEEE Trans. Vis.
Comput. Graph., 19(12):2022–2031.
Culotta, A. (2010). Towards detecting influenza epidemics
by analyzing twitter messages. In Proceedings of the
First Workshop on Social Media Analytics, SOMA
’10, pages 115–122, New York, NY, USA. ACM.
Dork, M., Gruen, D., Williamson, C., and Carpendale, S.
(2010). A visual backchannel for large-scale events.
IEEE Transactions on Visualization and Computer
Graphics, 16(6):1129–1138.
Havre, S., Hetzler, E., Whitney, P., and Nowell, L. (2002).
Themeriver: Visualizing thematic changes in large
document collections. Visualization and Computer
Graphics, IEEE Transactions on, 8(1):9–20.
Java, A., Song, X., Finin, T., and Tseng, B. (2007). Why we
twitter: understanding microblogging usage and com-
munities. In Proceedings of the 9th WebKDD and 1st
SNA-KDD 2007 workshop on Web mining and social
network analysis, pages 56–65. ACM.
Krstaji
´
c, M., Bertini, E., and Keim, D. A. (2011). Cloud-
lines: Compact display of event episodes in multi-
ple time-series. IEEE Trans. Vis. Comput. Graph.,
17(12):2432–2439.
Kwak, H., Lee, C., Park, H., and Moon, S. (2010). What
is Twitter, a social network or a news media? In
Proceedings of the 19th international conference on
World wide web, WWW ’10, pages 591–600. ACM.
Lee, S., Lee, S., Kim, K., and Park, J. (2012). Bursty event
detection from text streams for disaster management.
In Proceedings of the 21st international conference
companion on World Wide Web, WWW ’12 Compan-
ion, pages 679–682, New York, NY, USA. ACM.
Li, J., Maier, D., Tufte, K., Papadimos, V., and Tucker, P. A.
(2005). No Pane, No Gain: Efficient Evaluation of
Sliding-Window Aggregates over Data Streams. SIG-
MOD Record, 34(1):39–44.
Li, J., Tufte, K., Shkapenyuk, V., Papadimos, V., Johnson,
T., and Maier, D. (2008). Out-of-Order Processing: A
New Architecture for High-Performance Stream Sys-
tems. PVLDB, 1(1):274–288.
MacEachren, A., Jaiswal, A., Robinson, A., Pezanowski,
S., Savelyev, A., Mitra, P., Zhang, X., and Blanford, J.
(2011). Senseplace2: Geotwitter analytics support for
situational awareness. In Visual Analytics Science and
Technology (VAST), 2011 IEEE Conference on, pages
181–190.
Maier, D., Grossniklaus, M., Moorthy, S., and Tufte, K.
(2012). Capturing Episodes: May the Frame Be With
You. In Proc. Intl. Conf. on Distributed Event-Based
Systems (DEBS), pages 1–11.
Marcus, A., Bernstein, M. S., Badar, O., Karger, D. R.,
Madden, S., and Miller, R. C. (2011). Twitinfo: ag-
gregating and visualizing microblogs for event explo-
ration. In Proceedings of the 2011 annual conference
IVAPP2015-InternationalConferenceonInformationVisualizationTheoryandApplications
38