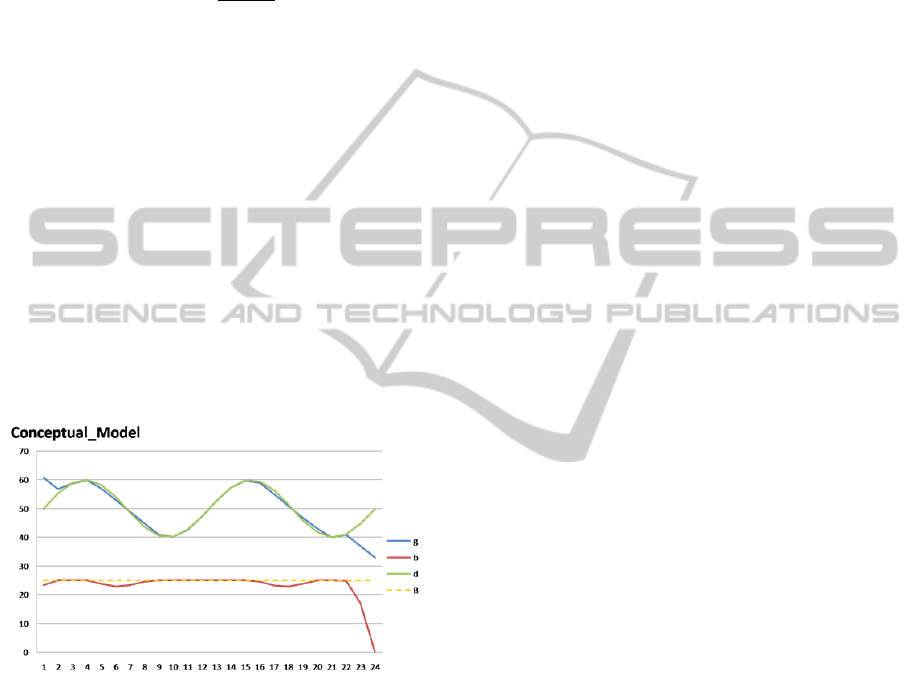
single-generator case under the assumption of an
electrical “per unit” DC model.
The considered planning horizon is
T24
hours
and each time step
t
has a duration of
1
hour.
According to the original model, the demand (in GJ
units) profile in the planning horizon is represented
by:
5010sin
(7)
The energy storage system is characterized by a
battery capacity
B25
GJ, and an initial level of
charge
b
0
12.5
GJ.
The additive components to the cost function
Z
−to
be minimized− are:
0.5
(8)
(9)
As proposed by Chandy et al. (2010), in our “basic”
case study some simplifications are included: i) the
last component, related to the final level of charge of
the batteries, is obmitted; ii) the cost coefficient is
constant in the planning horizon and fixed to
γ
t
1
for all
t
(invariant case) While the value of the
coefficient a was set to
2
.
Figure 2: Conceptual Model Results for the basic case
study. The
x
-axis reports the time slots,
y
-axis indicates
power levels.
As depicted in Figure 2, according to Chandy et al.
(2010), the optimal generation
g
t
is linear when the
batteries charge and discharge, and follows the
demand when the storage system is at the maximum
level of charge. In the particular illustrative problem
setting, the storage system is hardly used at all and
appears quite oversized. In fact, it is mainly due to
the relative values of generation and storage costs
and their invariance in the planning horizon.
The energy storage system clearly needs a better
modeling to deal with the level of charge at the end
of the period. The decision-makers needs to consider
in the planning activities also the preference and
limitations on the generation-side and the possible
uncertainties on the demand-side.
3.3 OPF: Enhanced Models
Starting from the Conceptual Model, and on the
basis of the requirements defined in the application
context, we are working, together with the decision-
makers, on several modeling extensions mainly
devoted to address the following issues, which
compose our current research agenda:
1. Limitations on the flows to/from the storage
system in each time slot;
2. Generated flow possibly assumes negative values
(i.e., the Distribution System Operator (DSO)
should receive energy from the node);
3. Demand predictions possibly assume negative
values (i.e., customers should produce energy);
4. (Upper/Lower) Bounds on the amount of
generated energy (possibly negative), e.g.,
a) constant bounds;
b) time-dependent (yet known) bounds;
c) preferences (penalty based) on the level of
power generation;
5. Uncertainties affecting the demand forecasts
d
t
;
6. Storage system inefficiencies with respect to
holding, discharging and recharging phases;
7. Possible different energy sources;
8. More specific constraints related to the discharge
and recharge phases of specific classes of energy
storage systems;
9. Extension to a multi-generator and multi-bus
context.
In particular, in this work we address the modeling
issues related to points 4 and 5.
3.3.1 Uncertain Demand Forecasts
In general, uncertainties affecting the demand
forecasts
d
t
are described as prediction intervals and
error distributions (Box et al., 2008, Pflug and
Römisch, 2007, Narayanaswamy et al., 2012,
Conejo et al., 2010). On the basis of a demand
forecast, the planner receives, for each time slot, the
predicted value (i.e.,
d
t
), the prediction interval, and
the distribution of the values inside that interval.
On the basis of the forecasting values distribution
within the prediction interval (e.g., from
Autoregressive Integrated Moving Average
ICORES2015-InternationalConferenceonOperationsResearchandEnterpriseSystems
226