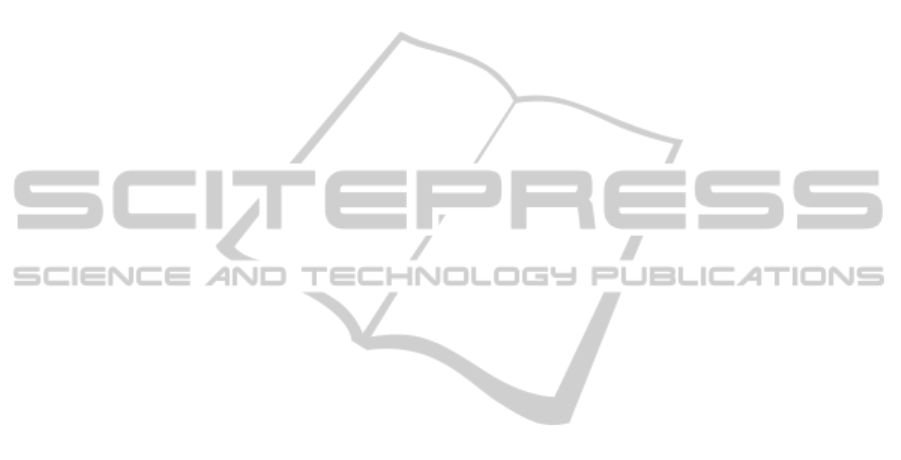
5 CONCLUSION
We have improved the system to detect and recog-
nize engineered nanoparticles we proposed in (Kock-
entiedt et al., 2012). We have added an appropriate
filter to the image segmentation and reviewed its pa-
rameters. Moreover, we have added two types of clas-
sification features: Haralick features and estimated
electron counts. We have shown that they add consid-
erable value to the system by testing how often these
features have been selected for the training of the clas-
sifier. In addition, we have introduced an algorithm
to automatically select the best classification parame-
ters and features. This way, even inexperienced users
can train the system to recognize new particle types
without setting any parameters. The improved system
achieves much better results than the original one for
two engineered nanoparticle types and comparable re-
sults for a third type.
In the future, we want to further improve the us-
ability of the system and reduce the amount of manual
work. Firstly, we want to reduce the number of sam-
ples that have to be manually classified by automat-
ically selecting the best candidates to be classified.
This approach is called active learning.
Secondly, we want to allow the system to pre-
dict the classification performance to be expected if
more training samples are added. This way, the user
can make an informed decision if it is worth spend-
ing time to make more SEM images to generate more
training samples. If the classification performance is
unlikely to be significantly improved, the user can
save time and money which would otherwise have
been spent. Early results of this are reported in (Kock-
entiedt et al., 2014).
ACKNOWLEDGEMENTS
We kindly thank U. Gernert from ZELMI for the
cooperation in SEM analysis. This work was sup-
ported by funding from the Deutsche Forschungsge-
meinschaft (DFG INST 131/631-1).
REFERENCES
Buades, A., Coll, B., and Morel, J.-M. (2005). A non-local
algorithm for image denoising. In IEEE Computer
Society Conference on Computer Vision and Pattern
Recognition, volume 2, pages 60–65. IEEE.
Flores, A. B., Robles, L. A., Arias, M. O., and Ascencio,
J. A. (2003). Small metal nanoparticle recognition us-
ing digital image analysis and high resolution electron
microscopy. Micron, 34(2):109–118.
Hall, M., Frank, E., Holmes, G., Pfahringer, B., Reutemann,
P., and Witten, I. H. (2009). The WEKA data min-
ing software: An update. ACM SIGKDD Explorations
Newsletter, 11(1):10–18.
Jain, A. K., Duin, R. P., and Mao, J. (2000). Statistical
pattern recognition: A review. IEEE Transactions on
Pattern Analysis and Machine Intelligence, 22(1):4–
37.
Kockentiedt, S., T
¨
onnies, K., and Gierke, E. (2014). Pre-
dicting the influence of additional training data on
classification performance for imbalanced data. In
Jiang, X., Hornegger, J., and Koch, R., editors, Pro-
ceedings of the 36th German Conference on Pattern
Recognition, GCPR 2014, volume 8753 of Lecture
Notes in Computer Science, pages 377–387. Springer
International Publishing.
Kockentiedt, S., T
¨
onnies, K., Gierke, E., Dziurowitz, N.,
Thim, C., and Plitzko, S. (2012). Automatic detection
and recognition of engineered nanoparticles in SEM
images. In VMV 2012: Vision, Modeling & Visualiza-
tion, pages 23–30. Eurographics Association.
Kockentiedt, S., T
¨
onnies, K., Gierke, E., Dziurowitz, N.,
Thim, C., and Plitzko, S. (2013). Poisson shot noise
parameter estimation from a single scanning electron
microscopy image. In Egiazarian, K. O., Agaian,
S. S., and Gotchev, A. P., editors, Proc. SPIE 8655,
Image Processing: Algorithms and Systems XI, vol-
ume 8655, pages 86550N–86550N–13.
Kubat, M., Holte, R. C., and Matwin, S. (1998). Machine
learning for the detection of oil spills in satellite radar
images. Machine Learning, 30(2-3):195–215.
Laghari, M. S. (2003). Recognition of texture types of
wear particles. Neural Computing & Applications,
12(1):18–25.
Langford, M., Taylor, G., and Flenley, J. (1990). Comput-
erized identification of pollen grains by texture anal-
ysis. Review of Palaeobotany and Palynology, 64(1-
4):197–203.
Oleshko, V. P., Kindratenko, V. V., Gijbels, R. H., Van Es-
pen, P. J. M., and Jacob, W. A. (1996). Study of
quasi-fractal many-particle-systems and percolation
networks by zero-loss spectroscopic imaging, electron
energy-loss spectroscopy and digital image analysis.
Mikrochimica Acta Supplement, 13:443–451.
Oster, T. (2010). Erkennung von Nanofaseragglomer-
aten in REM-Bildern auf Basis von Texturinformatio-
nen. Bachelor thesis, Otto-von-Guericke University
of Magdeburg.
Ostrowski, A. D., Martin, T., Conti, J., Hurt, I., and Herr
Harthorn, B. (2009). Nanotoxicology: Characteriz-
ing the scientific literature, 2000-2007. Journal of
Nanoparticle Research, 11(2):251–257.
Rodriguez-Damian, M., Cernadas, E., Formella, A., and
Fernandez-Delgado, M. (2006). Automatic detection
and classification of grains of pollen based on shape
and texture. IEEE Transactions on Systems, Man
and Cybernetics, Part C (Applications and Reviews),
36(4):531–542.
Rosin, P. L. (2001). Unimodal thresholding. Pattern Recog-
nition, 34(11):2083–2096.
ImprovedAutomaticRecognitionofEngineeredNanoparticlesinScanningElectronMicroscopyImages
343