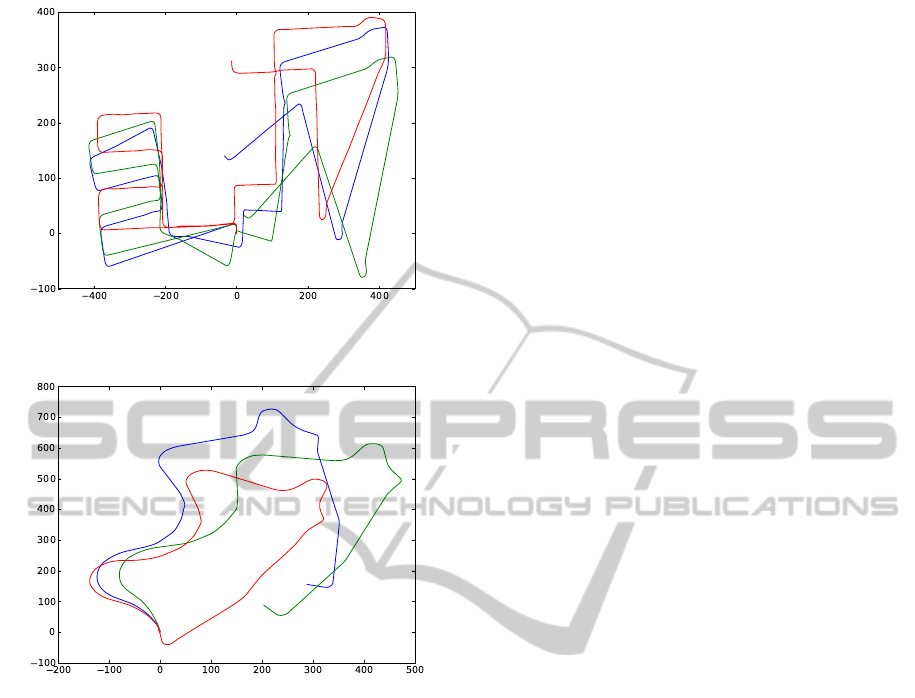
(a) Train sequence (Seq.8)
(b) Test sequence (Seq.9)
Figure 6: Original ground truth path is displayed in Red,
discretised ground truth in Green and the estimated one in
Blue.
based landmark detection scheme which will help in
automatic loop closure. In the current form the ap-
proach cannot be compared to the state-of-the-art ap-
proaches for visual odometry in terms of precision.
We believe our work is a step towards building a com-
mon architecture for many vision tasks like object
classification, depth estimation, activity analysis and
visual odometry.
ACKNOWLEDGEMENTS
This work was supported in part by the German Fed-
eral Ministry of Education and Research (BMBF) in
projects 01GQ0841 (BFNT Frankfurt), by an NSERC
Discovery grant and by a Google faculty research
award.
REFERENCES
Badino, H., Yamamoto, A., and Kanade, T. (2013). Visual
odometry by multi-frame feature integration. In Com-
puter Vision Workshops (ICCVW), 2013 IEEE Inter-
national Conference on, pages 222–229. IEEE.
Bergstra, J., Breuleux, O., Bastien, F., Lamblin, P., Pascanu,
R., Desjardins, G., Turian, J., Warde-Farley, D., and
Bengio, Y. (2010). Theano: a CPU and GPU math
expression compiler. In SciPy.
Chandraker, M., Reddy, D., Wang, Y., and Ramamoorthi, R.
(2013). What object motion reveals about shape with
unknown brdf and lighting. In Computer Vision and
Pattern Recognition (CVPR), 2013 IEEE Conference
on, pages 2523–2530. IEEE.
Eigen, D., Puhrsch, C., and Fergus, R. (2014). Depth map
prediction from a single image using a multi-scale
deep network. In Advances in neural information pro-
cessing systems.
Geiger, A., Lenz, P., and Urtasun, R. (2012). Are we ready
for autonomous driving? the kitti vision benchmark
suite. In Conference on Computer Vision and Pattern
Recognition (CVPR).
Kitt, B., Geiger, A., and Lategahn, H. (2010). Visual odom-
etry based on stereo image sequences with ransac-
based outlier rejection scheme. In Intelligent Vehicles
Symposium (IV), 2010 IEEE, pages 486–492. IEEE.
Konda, K. R. and Memisevic, R. (2013). Unsupervised
learning of depth and motion. CoRR, abs/1312.3429.
Konda, K. R., Memisevic, R., and Michalski, V. (2014).
Learning to encode motion using spatio-temporal syn-
chrony. In Proceedings of ICLR.
Krizhevsky, A., Sutskever, I., and Hinton, G. E. (2012). Im-
agenet classification with deep convolutional neural
networks. In Advances in neural information process-
ing systems, pages 1097–1105.
Memisevic, R. (2011). Gradient-based learning of higher-
order image features. In Computer Vision (ICCV),
2011 IEEE International Conference on, pages 1591–
1598. IEEE.
Memisevic, R., Konda, K. R., and Krueger, D. (2014). Zero-
bias autoencoders and the benefits of co-adapting fea-
tures. CoRR, abs/1402.3337.
Nist
´
er, D., Naroditsky, O., and Bergen, J. (2004). Visual
odometry. In Computer Vision and Pattern Recogni-
tion, 2004. CVPR 2004. Proceedings of the 2004 IEEE
Computer Society Conference on, volume 1, pages I–
652. IEEE.
VISAPP2015-InternationalConferenceonComputerVisionTheoryandApplications
490