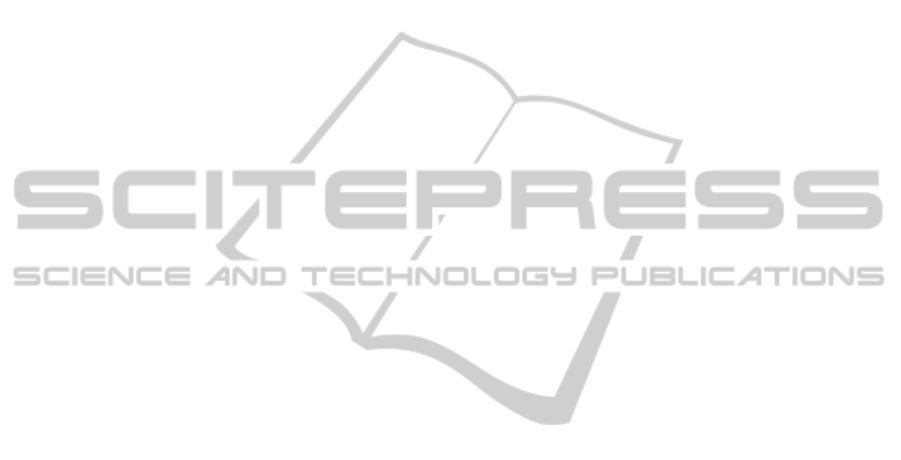
proposes a procedure to add any sort of contextual in-
formation that can be further generalized to include
other data apart from the object used during an ac-
tion. Additionally, the present approach shows that
the best results are obtained when kernels from spa-
cial, temporal, and tool informationare combined into
a multichannel SVM kernel. In this respect, the high-
est recognition rates are 71.57% using a combination
of trajectories, HOG and object. In the near future we
plan to add more contextual information –scene– in
order to improve the results.
ACKNOWLEDGEMENTS
This research has been partially supported by the
Industrial Doctorate program of the Government of
Catalonia, and by the European Community through
the FP7 framework program by funding the Vinbot
project (N 605630) conducted by Ateknea Solutions
Catalonia.
REFERENCES
Bilinski, P. and Corvee, E. (2013). Relative Dense Track-
lets for Human Action Recognition. 10th IEEE Inter-
national Conference on Automatic Face and Gesture
Recognition.
Chang, C.-C. and Lin, C.-J. (2011). LIBSVM:
A library for support vector machines. ACM
Transactions on Intelligent Systems and Tech-
nology, 2:27:1–27:27. Software available at
http://www.csie.ntu.edu.tw/ cjlin/libsvm.
Dalal, N. and Triggs, B. (2005). Histograms of oriented
gradients for human detection. In Proceedings of the
2005 IEEE Computer Society Conference on Com-
puter Vision and Pattern Recognition (CVPR’05) -
Volume 1 - Volume 01, CVPR ’05, pages 886–893,
Washington, DC, USA. IEEE Computer Society.
Dalal, N., Triggs, B., and Schmid, C. (2006). Human de-
tection using oriented histograms of flow and appear-
ance. In Proceedings of the 9th European Conference
on Computer Vision - Volume Part II, ECCV’06, pages
428–441, Berlin, Heidelberg. Springer-Verlag.
Dollar, P., Rabaud, V., Cottrell, G., and Belongie, S. (2005).
Behavior recognition via sparse spatio-temporal fea-
tures. In Proceedings of the 14th International Con-
ference on Computer Communications and Networks,
ICCCN ’05, pages 65–72, Washington, DC, USA.
IEEE Computer Society.
Hartley, R. I. and Zisserman, A. (2004). Multiple View Ge-
ometry in Computer Vision. Cambridge University
Press, ISBN: 0521540518, second edition.
Ikizler-Cinbis, N. and Sclaroff, S. (2010). Object, scene and
actions: Combining multiple features for human ac-
tion recognition. In Proceedings of the 11th European
Conference on Computer Vision: Part I, ECCV’10,
pages 494–507, Berlin, Heidelberg. Springer-Verlag.
Jiang, Y., Dai, Q., Xue, X., Liu, W., and Ngo, C. (2012).
Trajectory-based modeling of human actions withmo-
tion reference points. In European Conference on
Computer Vision (ECCV).
Kl¨aser, A., Marszałek, M., and Schmid, C. (2008). A spatio-
temporal descriptor based on 3d-gradients. In British
Machine Vision Conference, pages 995–1004.
Kuehne, H., Jhuang, H., Garrote, E., Poggio, T., and Serre,
T. (2011). HMDB: a large video database for human
motion recognition. In Proceedings of the Interna-
tional Conference on Computer Vision (ICCV).
Laptev, I. (2005). On space-time interest points. Int. J.
Comput. Vision, 64(2-3):107–123.
Lucas, B. D. and Kanade, T. (1981). An iterative image
registration technique with an application to stereo vi-
sion. In Proceedings of the 7th International Joint
Conference on Artificial Intelligence - Volume 2, IJ-
CAI’81, pages 674–679, San Francisco, CA, USA.
Morgan Kaufmann Publishers Inc.
Poppe, R. (2010). A survey on vision-based human action
recognition. Image Vision Comput., 28(6):976–990.
Reddy, K. K. and Shah, M. (2013). Recognizing 50 human
action categories of web videos. Mach. Vision Appl.,
24(5):971–981.
Schuldt, C., Laptev, I., and Caputo, B. (2004). Recognizing
human actions: A local svm approach. In Proceedings
of the Pattern Recognition, 17th International Confer-
ence on (ICPR’04) Volume 3 - Volume 03, ICPR ’04,
pages 32–36, Washington, DC, USA. IEEE Computer
Society.
Scovanner, P., Ali, S., and Shah, M. (2007). A 3-
dimensional sift descriptor and its application to ac-
tion recognition. In Proceedings of the 15th Inter-
national Conference on Multimedia, MULTIMEDIA
’07, pages 357–360, New York, NY, USA. ACM.
Snoek, C. G. M., Worring, M., and Smeulders, A. W. M.
(2005). Early versus late fusion in semantic video
analysis. In Proceedings of the 13th Annual ACM
International Conference on Multimedia, MULTIME-
DIA ’05, pages 399–402, New York, NY, USA. ACM.
Solmaz, B., Modiri, S. A., and Shah, M. (2012). Classifying
web videos using a global video descriptor. Machine
Vision and Applications.
Wang, H., Kl¨aser, A., Schmid, C., and Liu, C. (2013).
Dense trajectories and motion boundary descriptors
for action recognition. International Journal of Com-
puter Vision.
Wang, H., Kl¨aser, A., Schmid, C., and Liu, C.-L. (2011).
Action Recognition by Dense Trajectories. In IEEE
Conf. on Computer Vision & Pattern Recognition,
pages 3169–3176, Colorado Springs, United States.
Wang, H. and Schmid, C. (2013). Action Recognition with
Improved Trajectories. In ICCV 2013 - IEEE Interna-
tional Conference on Computer Vision, pages 3551–
3558, Sydney, Australie. IEEE.
Weinland, D., Ronfard, R., and Boyer, E. (2011). A sur-
vey of vision-based methods for action representation,
UsingActionObjectsContextualInformationforaMultichannelSVMinanActionRecognitionApproachbasedonBagof
Visual Words
85