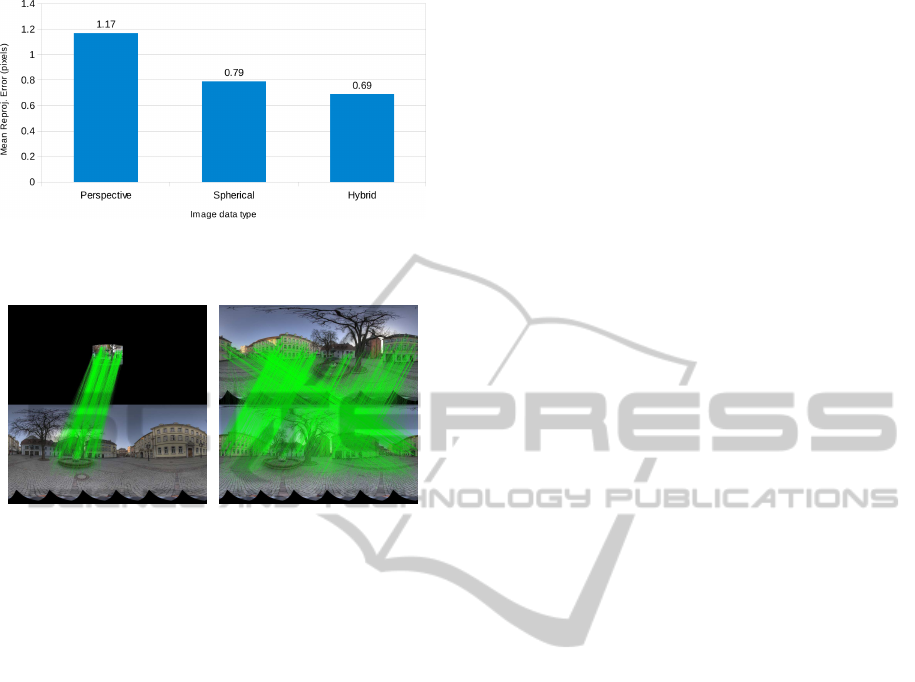
Figure 9: Global mean reprojection error for the hybrid St.
Martin Square experiment. Note how it decreases when per-
spective and spherical images are used together.
(a) (b)
Figure 10: (a) Symmetric matches between a warped per-
spective image and a spherical image. Matches are drawn
on their corresponding pixel maps to ease visualization. (b)
Symmetric matches between two full spherical images.
tween algorithms developed for perspective,spherical
and catadioptric images. Through extensive quantita-
tive evaluation on synthetic and real image sequences,
we showed that our approach delivers high quality
camera pose as well as scene geometry estimations
when compared to state of the art approaches opti-
mized for specific camera types.
Future work aims at integrating the optimization
of intrinsic parameters to increase the accuracy of
perspective cameras pose estimation. Additionally,
we plan to validate our framework on larger, hybrid
image datasets, supported by groundtruth data. Fi-
nally, SPHERA will be the underlying SfM mecha-
nism in our upcoming dense multi-view reconstruc-
tion approach.
ACKNOWLEDGEMENTS
This work was funded by the project DENSITY
(01IW12001). The authors would like to thank
Richard Schulz for the creation of the synthetic
dataset.
REFERENCES
Agarwal, S., Mierle, K., and Others (2010). Ceres solver.
https://code.google.com/p/ceres-solver/.
Agarwal, S., Snavely, N., Simon, I., Seitz, S. M., and
Szeliski, R. (2009). Building rome in a day. In ICCV,
pages 72–79, Kyoto, Japan.
Aly, M. and Bouguet, J.-Y. (2012). Street view goes in-
doors: Automatic pose estimation from uncalibrated
unordered spherical panoramas. In WACV, pages 1–8.
IEEE.
Anguelov, D., Dulong, C., Filip, D., Frueh, C., Lafon,
S., Lyon, R., Ogale, A., Vincent, L., and Weaver, J.
(2010). Google street view: Capturing the world at
street level. Computer, 43.
Bagnato, L., Frossard, P., and Vandergheynst, P. (2011). A
Variational Framework for Structure from Motion in-
Omnidirectional Image Sequences. Journal of Math-
ematical Imaging and Vision, 41(3):182–193.
Blender (2014). http://www.blender.org/. Retrieved Octo-
ber 19, 2014.
Chang, P. and Hebert, M. (2000). Omni-directional struc-
ture from motion. In OMNIVIS, Washington, DC,
USA. IEEE Computer Society.
Civetta (2009). http://www.weiss-ag.org/solutions/civetta/.
Retrieved October 16, 2014.
Furukawa, Y., Curless, B., Seitz, S. M., and Szeliski, R.
(2010). Towards internet-scale multi-view stereo. In
CVPR.
Furukawa, Y. and Ponce, J. (2008). Accurate, dense, and
robust multi-view stereopsis. IEEE Transactions on
Pattern Analysis and Machine Intelligence, 01(01):1–
14.
Gava, C. C., Hengen, J.-M., Taetz, B., and Stricker, D.
(2013). Keypoint detection and matching on high res-
olution spherical images. In ISVC, pages 363–372,
Rethymnon, Crete, Greece.
Geyer, C. and Daniilidis, K. (2001). Catadioptric projective
geometry. Int. Journal of Computer Vision, 43:223–
243.
Hartley, R. I. and Zisserman, A. (2004). Multiple View Ge-
ometry in Computer Vision. Cambridge University
Press, ISBN: 0521540518, second edition.
Huynh, D. Q. (2009). Metrics for 3d rotations: Comparison
and analysis. Journal of Mathematical Imaging and
Vision, 35(2):155–164.
Kangni, F. and Laganiere, R. (2007). Orientation and pose
recovery from spherical panoramas. ICCV, 0:1–8.
LizardQ (2014). http://www.lizardq.com. Retrieved Octo-
ber 16, 2014.
Lowe, D. G. (2004). Distinctive image features from scale-
invariant keypoints. Int. Journal of Computer Vision,
60:91–110.
Ma, Y., Soatto, S., Kosecka, J., and Sastry, S. (2003). An in-
vitation to 3D vision, from images to models. Springer
Verlag.
Micusik, B. and Pajdla, T. (2006). Structure from motion
with wide circular field of view cameras. IEEE Trans.
Pattern Anal. Mach. Intell., 28(7):1135–1149.
VISAPP2015-InternationalConferenceonComputerVisionTheoryandApplications
292