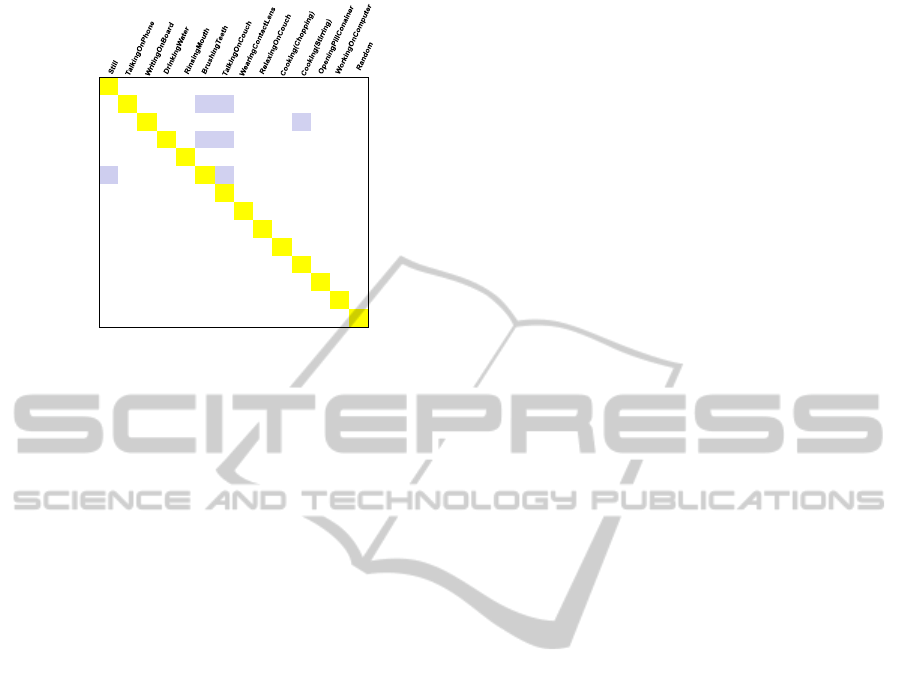
1.0
.25 .25 .50
.75 .25
.25 .25 .50
1.0
.25 .50 .25
1.0
1.0
1.0
1.0
1.0
1.0
1.0
1.0
Still
TalkingOnPhone
WritingOnBoard
DrinkingWater
RinsingMouth
BrushingTeeth
WearingContactLens
TalkingOnCouch
RelaxingOnCouch
Cooking(Chopping)
Cooking(Stirring)
OpeningPillContainer
WorkingOnComputer
Random
Figure 7: Confusion matrix for Cornell Activity Dataset.
7 CONCLUSION
In this paper, we presented a novel way of using the
bag-of-words model to represent an action sample
from noisy skeleton data. We have proposed a set
of novel joints based features and used these in the
proposed Bag-of-Joint-Features (BoJF) model. Fur-
ther, to take into account temporal differences within
and outside an action class, we have proposed the
Hierarchical Temporal-histogram (HT-hist) model.
We tested our approach on the MSR-Action3D and
Cornell activity datasets and obtained results that are
comparable with the other state-of-the-art methods.
The key advantage of this approach is that it provides
an efficient and simpler way of representing an
action sample. However, there are some challenges
to overcome. Actions involving interaction with
environment may not be well represented using just
the skeleton data. We may need data from other
channels and appropriate methods to represent such
actions. In future, we intend to use knowledge
from color and depth maps as well to improve the
recognition process.
REFERENCES
Bobick, A. F. and Davis, J. W. (2001). The recognition
of human movement using temporal templates. IEEE
Trans. Pattern Anal. Mach. Intell., 23(3):257–267.
Chang, C. C. and Lin, C. J. (2011). LIBSVM: A Library for
Support Vector Machines. ACM Trans. Intell. Syst.
Technol., 2(3).
Jin, S. Y. and Choi, H. J. Essential body-joint and atomic
action detection for human activity recognition using
longest common subsequence algorithm. In Computer
Vision - ACCV 2012 Workshops, volume 7729 of Lec-
ture Notes in Computer Science, pages 148–159.
Koppula, H., Gupta, R., and Saxena, A. (2013). Learning
human activities and object affordances from RGB-D
videos. IJRR, 32(8):951–970.
Laptev, I. (2005). On space-time interest points. Int. J.
Comput. Vision, 64(2-3):107–123.
Laptev, I., Marszalek, M., Schmid, C., and Rozenfeld,
B. (2008). Learning realistic human actions from
movies. In Proceedings of the 2008 Conference on
Computer Vision and Pattern Recognition, Los Alami-
tos, CA, USA.
Lazebnik, S., Schmid, C., and Ponce, J. (2006). Beyond
bags of features: Spatial pyramid matching for rec-
ognizing natural scene categories. In Proceedings of
the 2006 IEEE Computer Society Conference on Com-
puter Vision and Pattern Recognition, volume 2, pages
2169–2178.
Li, W., Zhang, Z., and Liu, Z. (2010). Action recogni-
tion based on a bag of 3D points. In Proceedings of
the 2010 IEEE Computer Society Conference on Com-
puter Vision and Pattern Recognition.
Lv, F. and Nevatia, R. (2006). Recognition and segmenta-
tion of 3-d human action using HMM and Multi-class
Adaboost. In Proceedings of the 9th European Con-
ference on Computer Vision, pages 359–372.
Ni, B., Wang, G., and Moulin, P. (2011). RGBD-HuDaAct:
A color-depth video database for human daily activity
recognition. In ICCV Workshops, pages 1147–1153.
IEEE.
Niebles, J. C., Wang, H., and Fei-Fei, L. (2008). Un-
supervised learning of human action categories us-
ing spatial-temporal words. Int. J. Comput. Vision,
79(3):299–318.
Poppe, R. (2010). A survey on vision-based human action
recognition. Image Vision Comput., 28(6):976–990.
Schuldt, C., Laptev, I., and Caputo, B. (2004). Recognizing
human actions: A local SVM approach. In Proceed-
ings of the 17th International Conference on Pattern
Recognition, (ICPR’04), volume 3, pages 32–36.
Sung, J., Ponce, C., Selman, B., and Saxena, A. (2011).
Human activity detection from RGBD images. In
Association for the Advancement of Artificial Intelli-
gence (AAAI) workshop on Pattern, Activity and Intent
Recognition.
Sung, J., Ponce, C., Selman, B., and Saxena, A. (2012). Un-
structured human activity detection from RGBD im-
ages. In International Conference on Robotics and
Automation (ICRA).
Swain, M. and Ballard, D. (1991). Color indexing. In IJCV,
7(1):1132.
Turaga, P. K., Chellappa, R., Subrahmanian, V. S., and
Udrea, O. (2008). Machine recognition of human ac-
tivities: A survey. IEEE Trans. Circuits Syst. Video
Techn., 18(11):1473–1488.
Wang, J., Liu, Z., Wu, Y., and Yuan, J. (2012). Mining
actionlet ensemble for action recognition with depth
cameras. In Proceedings of the 2012 IEEE Conference
on Computer Vision and Pattern Recognition, CVPR
’12, pages 1290–1297.
Bag-of-FeaturesbasedActivityClassificationusingBody-jointsData
321