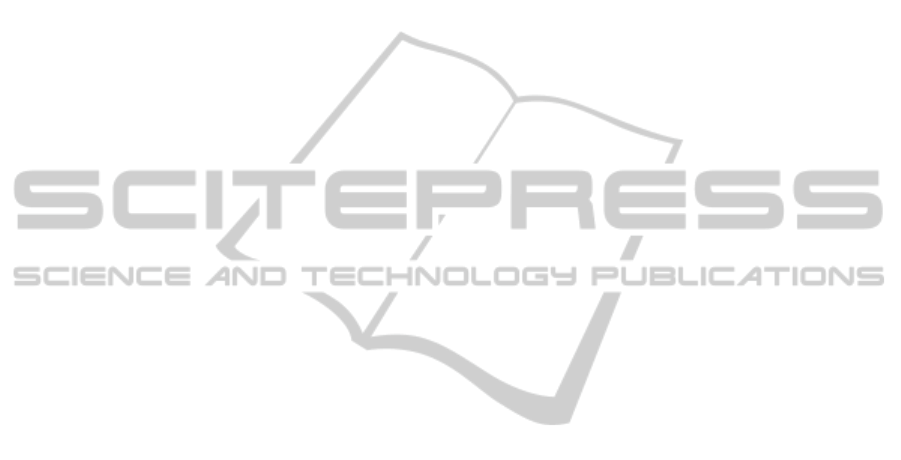
we will explore extension of our system for use with
different types of networks.
REFERENCES
Alper, B., Bach, B., Riche, N. H., Isenberg, T., and Fekete,
J.-D. (2013). Weighted graph comparison techniques
for brain connectivity analysis. In Mackay, W. E.,
Brewster, S. A., and Bødker, S., editors, CHI, pages
483–492. ACM.
B
¨
ottger, J., Schurade, R., Jakobsen, E., Schaefer, A., and
Margulies, D. S. (2014). Connexel visualization: a
software implementation of glyphs and edge-bundling
for dense connectivity data using braingl. Front Neu-
rosci, 8(15).
BSA (2014). Brainspan Atlas. See
http://www.brainspan.org/.
Bullmore, E. and Sporns, O. (2009). Complex brain net-
works: graph theoretical analysis of structural and
functional systems. Nat. Rev. Neurosci., 10(3):186–
198.
Chang, H.-M., Chiang, A.-S., Didimo, W., Lin, C.-Y., Li-
otta, G., and Montecchiani, F. (2013). On the robust-
ness of the drosophila neural network. In Network Sci-
ence Workshop (NSW), 2013 IEEE 2nd, pages 168–
171.
D3 (2014). Data Driven Documents. See http://d3js.org/.
Destrieux, C., Fischl, B., Dale, A., and Halgren, E. (2010).
Automatic parcellation of human cortical gyri and
sulci using standard anatomical nomenclature. Neu-
roImage, 53(1):1 – 15.
Greicius, M. D., Srivastava, G., Reiss, A. L., and Menon, V.
(2004). Default-mode network activity distinguishes
alzheimer’s disease from healthy aging: Evidence
from functional mri. Proceedings of the National
Academy of Sciences of the United States of America,
101(13):4637–4642.
Hagmann, P., Cammoun, L., Gigandet, X., Meuli, R.,
Honey, C. J., Wedeen, V. J., and Sporns, O. (2008).
Mapping the structural core of human cerebral cortex.
PLoS Biol, 6(7).
HBP (2014). The Human Brain Project. See
https://www.humanbrainproject.eu/.
Lee, H., Kang, H., Chung, M. K., Kim, B. N., and Lee, D. S.
(2012). Persistent brain network homology from the
perspective of dendrogram. IEEE Trans Med Imaging,
31(12):2267–2277.
Margulies, D. S., B
¨
ottger, J., Watanabe, A., and Gor-
golewski, K. J. (2013). Visualizing the human con-
nectome. NeuroImage, 80:445–461.
Nowke, C., Schmidt, M., van Albada, S., Eppler, J., Bakker,
R., Diesrnann, M., Hentschel, B., and Kuhlen, T.
(2013). Visnest – interactive analysis of neural ac-
tivity data. In Biological Data Visualization (BioVis),
2013 IEEE Symposium on, pages 65–72.
Onoda, K. and Yamaguchi, S. (2013). Small-worldness and
modularity of the resting-state functional brain net-
work decrease with aging. Neurosci. Lett., 556:104–
108.
Smith, S., Vidaurre, D., Beckmann, C., Glasser, M., Jenkin-
son, M., Miller, K., Nichols, T., Robinson, E., Salimi-
Khorshidi, G., Woolrich, M., Barch, D., Ugurbil, K.,
and DC, V. (2013). Functional connectomics from
resting-state fmri. Trends in Cognitive Sciences, 17.
Sorger, J., Buhler, K., Schulze, F., Liu, T., and Dickson, B.
(2013). neuromap – interactive graph-visualization of
the fruit fly’s neural circuit. In Biological Data Visu-
alization (BioVis), 2013 IEEE Symposium on, pages
73–80.
van den Heuvel, M. P. and Pol, H. E. H. (2010). Explor-
ing the brain network: A review on resting-state fmri
functional connectivity. European Neuropsychophar-
macology, 20(8):519 – 534.
Van Essen, D. C., Smith, S. M., Barch, D. M., Behrens,
T. E., Yacoub, E., and Ugurbil, K. (2013). The wu-
minn human connectome project: An overview. Neu-
roImage, 80(0):62 – 79. Mapping the Connectome.
Wang, J., Zuo, X., and He, Y. (2010). Graph-based network
analysis of resting-state functional mri. Frontiers in
Systems Neuroscience, 4(16).
Webcola (2014). WebCoLa. See
http://marvl.infotech.monash.edu/webcola/.
WebGL (2013). WebGL. See
https://www.khronos.org/webgl/.
XTK (2014). The X Toolkit. See
https://github.com/xtk/X#publications.
IVAPP2015-InternationalConferenceonInformationVisualizationTheoryandApplications
138