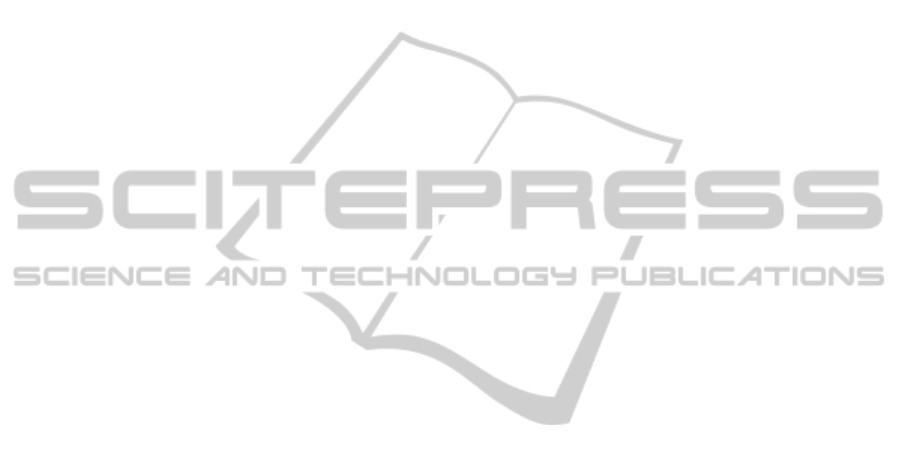
content. This is due to our method taking into account
region uniformity and consistency between consecu-
tive frames, which we included in the new criteria.
REFERENCES
Borsotti, M., Campadelli, P., and Schettini, R. (1998).
Quantitative evaluation of color image segmentation
results. Pattern Recognition Letters, 19(8):741 – 747.
Chabrier, S., Emile, B., Rosenberger, C., and Laurent, H.
(2006). Unsupervised performance evaluation of im-
age segmentation. EURASIP Journal on Applied Sig-
nal Processing, 2006:217–217.
Charron, C. and Hicks, Y. (2010). An evolving mog for
online image sequence segmentation. In ICIP, pages
2189–2192.
Chen, A. Y. C. and Corso, J. J. (2010). Propagating multi-
class pixel labels throughout video frames. In Proc. of
Western New York Image Processing Workshop.
Chen, H.-C. and Wang, S.-J. (2004). The use of visible
color difference in the quantitative evaluation of color
image segmentation. Proc. IEEE Int. Conf. on Acous-
tics, Speech, and Signal Processing (ICASSP), 3:iii–
593–6 vol.3.
Correia, P. and Pereira, F. (2003). Objective evaluation of
video segmentation quality. IEEE Trans. on Image
Processing, 12(2):186–200.
Dey, V., Zhang, Y., and Zhong, M. (2010). A review on im-
age segmentation techniques with remote sensing per-
spective. In Proc. of the Int. Society for Photogram-
metry and Remote Sensing Symposium (ISPRS), vol-
ume 38, pages 5–7.
Erdem, C¸ . E., Sankur, B., and Tekalp, A. M. (2004).
Performance measures for video object segmentation
and tracking. IEEE Trans. on Image Processing,
13(7):937–951.
Galasso, F., Nagaraja, N. S., C
´
ardenas, T. J., Brox, T.,
and Schiele, B. (2013). A unified video segmenta-
tion benchmark: Annotation, metrics and analysis. In
IEEE Int. Conf. on Computer Vision (ICCV).
Gelasca, E. D. and Ebrahimi, T. (2006). On evaluating met-
rics for video segmentation algorithms. In Second Int.
Workshop on Video Processing and Quality Metrics
for Consumer Electronics (VPQM ).
Greenspan, H., Dvir, G., and Rubner, Y. (2004). Context-
dependent segmentation and matching in image
databases. Computer Vision and Image Understand-
ing, 93(1):86–109.
Grundmann, M., Kwatra, V., Han, M., and Essa, I. (2010).
Efficient hierarchical graph-based video segmenta-
tion. In IEEE Conf. on Computer Vision and Pattern
Recognition (CVPR), pages 2141–2148.
Haralick, R. M. and Shapiro, L. G. (1985). Image segmen-
tation techniques. Computer vision, graphics, and im-
age processing, 29(1):100–132.
Kaloskampis, I. and Hicks, Y. (2014). Estimating adap-
tive coefficients of evolving gmms for online video
segmentation. In 6th Int. Symp. on Communications,
Control and Signal Processing (ISCCSP), pages 513–
516.
Levine, M. D. and Nazif, A. M. (1985). Dynamic measure-
ment of computer generated image segmentations.
IEEE Trans. on Pattern Analysis and Machine Intel-
ligence, PAMI-7(2):155–164.
Liu, J. and Yang, Y.-H. (1994). Multiresolution color image
segmentation. IEEE Trans. on Pattern Analysis and
Machine Intelligence, 16(7):689–700.
Martin, D., Fowlkes, C., Tal, D., and Malik, J. (2001). A
database of human segmented natural images and its
application to evaluating segmentation algorithms and
measuring ecological statistics. In Proc. Eighth IEEE
Int. Conf. on Computer Vision (ICCV), volume 2,
pages 416–423.
Meil
˘
a, M. (2003). Comparing clusterings by the variation of
information. In Learning theory and kernel machines,
volume 2777, pages 173–187.
Morris, O., Lee, M. d. J., and Constantinides, A. (1986).
Graph theory for image analysis: an approach based
on the shortest spanning tree. Communications, Radar
and Signal Processing, IEE Proc. F, 133(2):146–152.
Rosenberger, C. and Chehdi, K. (2000). Genetic fusion:
application to multi-components image segmentation.
In Proc. IEEE Int. Conf. on Acoustics, Speech, and
Signal Processing (ICASSP), volume 6, pages 2223–
2226.
Unnikrishnan, R., Pantofaru, C., and Hebert, M. (2005). A
measure for objective evaluation of image segmenta-
tion algorithms. In IEEE Computer Society Conf. on
Computer Vision and Pattern Recognition-Workshops
(CVPR) Workshops, pages 34–34.
Unnikrishnan, R., Pantofaru, C., and Hebert, M. (2007). To-
ward objective evaluation of image segmentation al-
gorithms. IEEE Trans. on Pattern Analysis and Ma-
chine Intelligence, 29(6):929–944.
Xu, C. and Corso, J. J. (2012). Evaluation of super-voxel
methods for early video processing. In IEEE Conf.
on Computer Vision and Pattern Recognition (CVPR),
pages 1202–1209.
Zhang, H., Fritts, J. E., and Goldman, S. A. (2004). An
entropy-based objective evaluation method for image
segmentation. In Electronic Imaging, pages 38–49.
Zhang, H., Fritts, J. E., and Goldman, S. A. (2008). Image
segmentation evaluation: A survey of unsupervised
methods. Computer Vision and Image Understanding,
110(2):260–280.
VISAPP2015-InternationalConferenceonComputerVisionTheoryandApplications
530