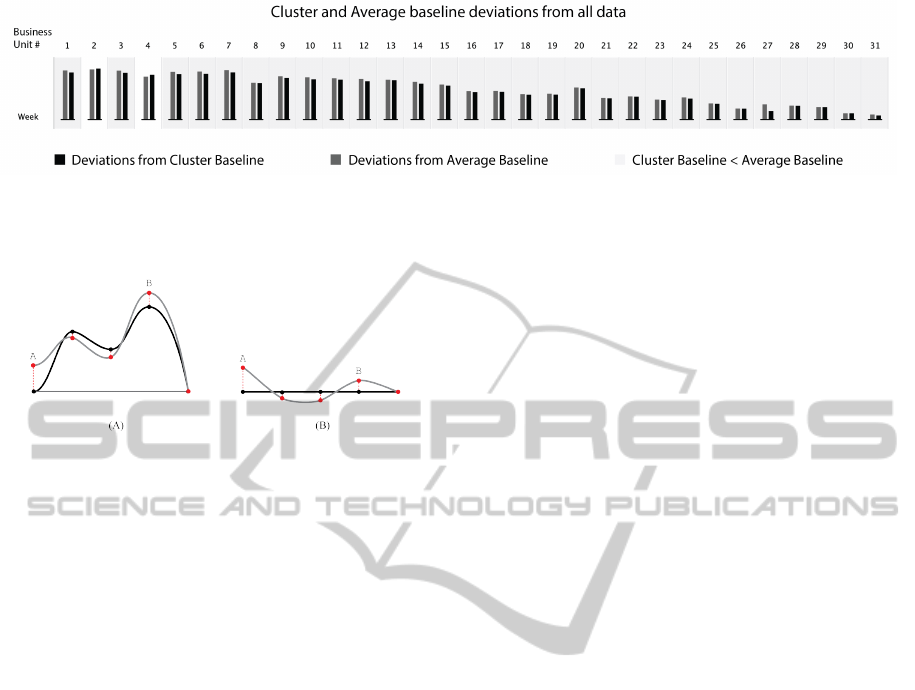
Figure 11: In this graph it is represented the average deviation from the week-based baselines created through the simply
average and the clustering methods with all dataset. Each column represents one of the 31 Business Units. When the cluster
baseline’s deviation is lower then the average baseline the corresponding area is filled with light grey.
Figure 12: On the left schematic we can see the baseline,
in black, and the consumption line, in grey. Here a set of
points are marked and the distance between them are the
deviations of the consumption values to the baseline. On
the right schematic, these deviations are translated to the
new visualization approach.
holidays. We can also see a drop of consumptions
between the days 24 and 29, which matches with the
Christmas period. With this visualization model we
managed to eliminate the periodic repetition, and em-
phasize moments of greater or lesser importance. Be-
sides the deviations are really clear and we can easily
understand what is above or bellow the baseline, it’s
difficult to compare the values.
To get a general overview of the deviations from
the baselines for the whole dataset we developed a
calendar view that improves the comparison among
deviations as well as better highlight the temporal mo-
ments when certain deviation pattern occurs. Since
this calendar view displays the overall consumption
in a day, we generated new week-based baselines
through clustering, where the consumptions are ag-
gregated by day.
In this calendar view, each month is positioned
from left to right, and the days of the week are po-
sitioned from top to bottom, from Monday to Sunday,
respectively. Each day of the month is placed on the
corresponding row, so, all week days in the visualiza-
tion are horizontally aligned. Each day is represented
by a rectangle (Figure 14). The top and bottom edges
of the rectangles represent, respectively, the lower and
higher consumption value of the represented Business
Unit or Department in all dataset. The baseline is
a black horizontal line positioned over the rectangle.
Since we are using a week-based baseline, for each
row of the visualization (from Monday to Sunday) the
line will be positioned at different positions, accord-
ingly to the baseline’s value for the corresponding day
of the week. From each baseline, we draw a rectan-
gle, with a height corresponding to the deviation in
consumption for the respective day, coloring it red, if
it is positive, and Persian green one, if it is negative.
With this method, we can represent all deviations in
a calendar view, emphasizing temporal patterns in the
deviations. With this visualization we can have two
levels of information: (i) a general overview of all
days where it is possible to see the highest deviations
among the different days, and (ii) a more local view to
compare how much the consumption of one day have
deviated from the baseline.
An example of this method can be seen on Fig-
ure 15, where we represent the consumption values
of the Business Unit Drinks for the 730 days, by us-
ing a week-based baseline created with the cluster-
ing method. We can say that the consumption in this
Business Unit does not have many atypical days. In
the two years we can see the same behavior: from
July to September and in December the sales tend to
be higher than the usual, probably due the summer va-
cations and Christmas. The calendar views were gen-
erated for each Department and Business Unit, but it
is our intent to generate more specific views for cat-
egories in the product hierarchy. With this last vi-
sualization model we can have a qualitative analysis
about the consumptions through time and understand
behaviors that tend to repeat through months and even
through years. It is easy to understand when the con-
sumption is a higher or lower value, and how the de-
viations tend to evolve.
5 RESULTS AND CONCLUSION
Big Data intensifies the ability to make decisions
within organizations, to discover new sales opportu-
nities and to improve the understanding of profitabil-
IVAPP2015-InternationalConferenceonInformationVisualizationTheoryandApplications
244