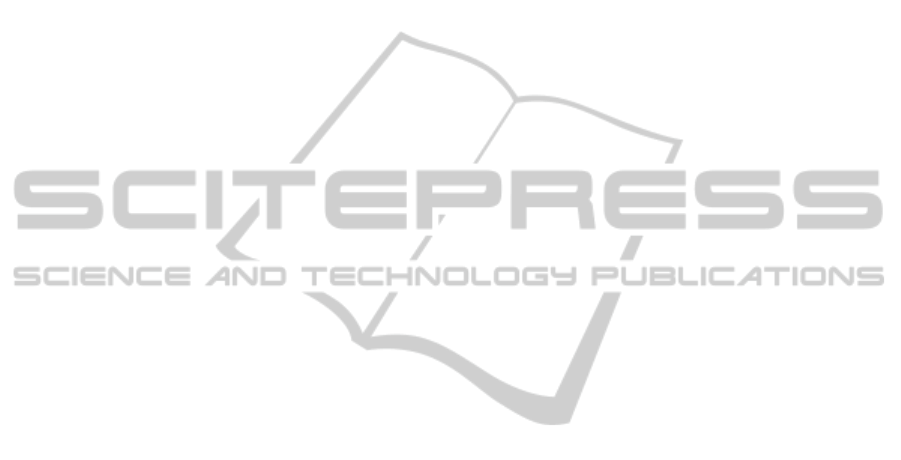
(2012b). Pedestrian detection at 100 frames per sec-
ond. In Proceedings of CVPR, pages 2903–2910.
Benenson, R., Mathias, M., Tuytelaars, T., and Van Gool, L.
(2013). Seeking the strongest rigid detector. In Proc.
of CVPR, pages 3666–3673, Portland, Oregon.
Benenson, R., Omran, M., Hosang, J., and Schiele, B.
(2014). Ten years of pedestrian detection, what have
we learned? In ECCV, CVRSUAD workshop.
Benezeth, Y., Jodoin, P.-M., Emile, B., Laurent, H., and
Rosenberger, C. (2008). Review and evaluation of
commonly-implemented background subtraction al-
gorithms. In Pattern Recognition, 2008. ICPR 2008.
19th International Conference on, pages 1–4. IEEE.
Benfold, B. and Reid, I. (2011). Stable multi-target tracking
in real-time surveillance video. In CVPR, pages 3457–
3464.
Breitenstein, M. D., Reichlin, F., Leibe, B., Koller-Meier,
E., and Van Gool, L. (2011). Online multiper-
son tracking-by-detection from a single, uncalibrated
camera. IEEE PAMI, 33(9):1820–1833.
CAVIAR project (2005). The CAVIAR project: Context
aware vision using image-based active recognition.
http://homepages.inf.ed.ac.uk/rbf/CAVIAR/.
Cho, H., Rybski, P., Bar-Hillel, A., and Zhang, W. (2012).
Real-time pedestrian detection with deformable part
models. In IEEE Intelligent Vehicles Symposium,
pages 1035–1042.
Dalal, N. and Triggs, B. (2005). Histograms of oriented gra-
dients for human detection. In Proceedings of CVPR,
volume 2, pages 886–893.
Doll´ar, P., Appel, R., Belongie, S., and Perona, P. (2014).
Fast feature pyramids for object detection.
Doll´ar, P., Belongie, S., and Perona, P. (2010). The fastest
pedestrian detector in the west. In Proceedings of
BMVC, pages 68.1–68.11.
Doll´ar, P., Tu, Z., Perona, P., and Belongie, S. (2009a). Inte-
gral channel features. In Proc. of BMVC, pages 91.1–
91.11.
Doll´ar, P., Wojek, C., Schiele, B., and Perona, P. (2009b).
Pedestrian detection: A benchmark. In Proceedings
of CVPR, pages 304–311.
Doll´ar, P., Wojek, C., Schiele, B., and Perona, P. (2012).
Pedestrian detection: An evaluation of the state of the
art. In IEEE PAMI, 34:743–761.
Felzenszwalb, P., Girschick, R., and McAllester, D. (2010).
Cascade object detection with deformable part mod-
els. In Proceedings of CVPR, pages 2241–2248.
Felzenszwalb, P., McAllester, D., and Ramanan, D. (2008).
A discriminatively trained, multiscale, deformable
part model. In Proceedings of CVPR.
Girshick, R., Donahue, J., Darrell, T., and Malik, J. (2014a).
Rich feature hierarchies for accurate object detection
and semantic segmentation. In Computer Vision and
Pattern Recognition.
Girshick, R., Felzenszwalb, P., and McAllester, D. (2012).
Discriminatively trained deformable part models, re-
lease 5. IEEE Transactions on Pattern Analysis and
Machine Intelligence.
Girshick, R. B., Iandola, F. N., Darrell, T., and Malik, J.
(2014b). Deformable part models are convolutional
neural networks. CoRR, abs/1409.5403.
Girshick, R. B. and Malik, J. (2013). Training deformable
part models with decorrelated features. In IEEE Inter-
national Conference on Computer Vision, ICCV 2013,
Sydney, Australia, December 1-8, 2013.
Kalman, R. (1960). A new approach to linear filtering
and prediction problems. In Transaction of the ASME
Journal of Basic Engineering, volume 82.
Krizhevsky, A., Sutskever, I., and Hinton, G. E. (2012). Im-
agenet classification with deep convolutional neural
networks. In Advances in Neural Information Pro-
cessing Systems 25, pages 1097–1105. Curran Asso-
ciates, Inc.
Leykin, A. and Hammoud, R. (2010). Pedestrian tracking
by fusion of thermal-visible surveillance videos. Ma-
chine Vision and Applications, 21(4):587–595.
Orts-Escolano, S., Garcia-Rodriguez, J., Morell, V., Ca-
zorla, M., Azorin, J., and Garcia-Chamizo, J. M.
(2014). Parallel computational intelligence-based
multi-camera surveillance system. Journal of Sensor
and Actuator Networks, 3(2):95–112.
Parks, D. H. and Fels, S. S. (2008). Evaluation of back-
ground subtraction algorithms with post-processing.
In Advanced Video and Signal Based Surveillance,
2008. AVSS’08. IEEE Fifth International Conference
on, pages 192–199. IEEE.
Pedersoli, M., Gonzalez, J., Hu, X., and Roca, X. (2013).
Toward real-time pedestrian detection based on a de-
formable template model. In IEEE ITS.
Rogez, G., Orrite, C., Guerrero, J. J., and Torr, P. H. S.
(2014a). Exploiting projective geometry for view-
invariant monocular human motion analysis in man-
made environments. Computer Vision and Image Un-
derstanding, 120:126–140.
Rogez, G., Rihan, J., Guerrero, J. J., and Orrite, C. (2014b).
Monocular 3D gait tracking in surveillance scenes.
IEEE Transactions on Systems Man and Cybernetics
Part B (Cybernetics), 44(6):894–909.
Russakovsky, O., Deng, J., Su, H., Krause, J., Satheesh, S.,
Ma, S., Huang, Z., Karpathy, A., Khosla, A., Bern-
stein, M., Berg, A. C., and Fei-Fei, L. (2014). Ima-
genet large scale visual recognition challenge.
Singh, V. K., Wu, B., and Nevatia, R. (2008). Pedestrian
tracking by associating tracklets using detection resid-
uals. In Motion and video Computing, 2008. WMVC
2008. IEEE Workshop on, pages 1–8. IEEE.
Van Beeck, K., Tuytelaars, T., and Goedem´e, T. (2012). A
warping window approach to real-time vision-based
pedestrian detection in a truck’s blind spot zone. In
Proceedings of ICINCO.
Zivkovic, Z. and van der Heijden, F. (2006). Efficient adap-
tive density estimation per image pixel for the task of
background subtraction. Pattern recognition letters,
27(7):773–780.
VISAPP2015-InternationalConferenceonComputerVisionTheoryandApplications
334