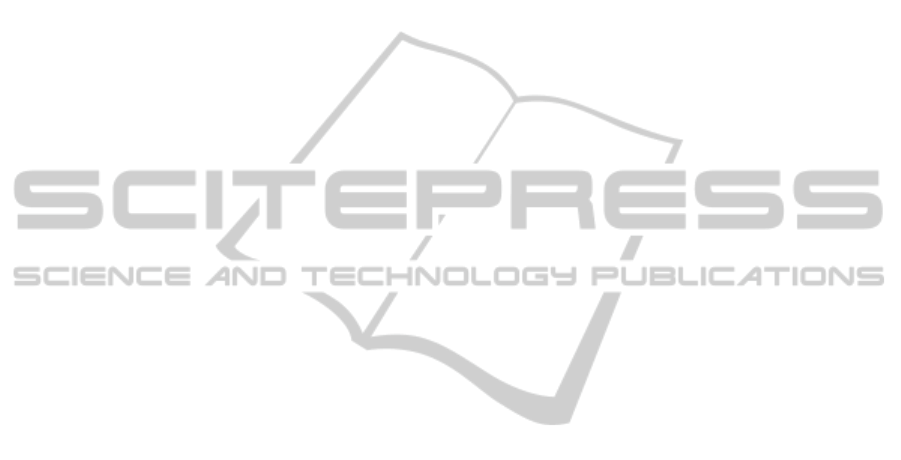
are computed in each region and a substantial model
of portrait aesthetic estimation is proposed. Com-
parison between different methods of aesthetic scores
and categories prediction has been made, and per-
formance of 4 recent works is significantly outper-
formed. The proposed feature selection process en-
hanced the overall prediction accuracy and the most
discriminant features and regions have been summa-
rized. Improvements are still to be done to deal effi-
ciently with rotated or occluded faces, and the frame-
work can be generalized to other kind of images by
replacing the face detection process by any adapted
segmentation algorithm.
In the future, results may be enhanced by the ad-
dition of high-level features. More precisely, it would
be interesting to consider attributes such as gender,
age, facial expression, eyes and mouth closeness, etc.
These attributes are closer to human perception of fa-
cial aesthetics than low-level statistics and can help to
perform more specific evaluation, to match with con-
sumer applications and to handle faces with glasses,
hats, make-up or facial hair.
REFERENCES
Aydin, T., Smolic, A., and Gross, M. (2014). Automated
Aesthetic Analysis of Photographic Images. IEEE
Transactions on Visualization and Computer Graph-
ics.
Chang, C. and Lin, C. (2011). LIBSVM: a library for sup-
port vector machines. ACM Transactions on Intelli-
gent Systems and Technologies, pages 1–39.
Crete, F., Dolmiere, T., Ladret, P., and Nicolas, M. (2007).
The blur effect: perception and estimation with a new
no-reference perceptual blur metric. In SPIE Elec-
tronic Image Symposium.
Datta, R., Joshi, D., Li, J., and Wang, J. (2006). Studying
aesthetics in photographic images using a computa-
tional approach. Computer VisionECCV 2006.
Desnoyer, M. and Wettergreen, D. (2010). Aesthetic Im-
age Classification for Autonomous Agents. 20th In-
ternational Conference on Pattern Recognition, pages
3452–3455.
Dhar, S., Ordonez, V., and Berg, T. (2011). High level de-
scribable attributes for predicting aesthetics and inter-
estingness. Computer Vision and Pattern Recognition,
pages 1657–1664.
Faria, J., Bagley, S., R
¨
uger, S., and Breckon, T. (2013).
Challenges of finding aesthetically pleasing images.
In Image Analysis for Multimedia Interactive Services
(WIAMIS), volume 2, pages 4–7.
Hasler, D. and Suesstrunk, S. (2003). Measuring colorful-
ness in natural images. Electronic Imaging. Interna-
tional Society for Optics and Photonics., pages 87–95.
He, K., Sun, J., and Tang, X. (2010). Single Image Haze Re-
moval Using Dark Channel Prior. IEEE transactions
on pattern analysis and machine intelligence.
Jiang, W., Loui, A. C., and Cerosaletti, C. D. (2010). Auto-
matic aesthetic value assessment in photographic im-
ages. IEEE International Conference on Multimedia
and Expo, pages 920–925.
Ke, Y., Tang, X., and Jing, F. (2006). The design of high-
level features for photo quality assessment. In Com-
puter Vision and Pattern Recognition, volume 1, pages
419–426.
Khan, S. and Vogel, D. (2012). Evaluating visual aesthetics
in photographic portraiture. Computational Aesthetics
in Graphics, Visualization and Imaging, pages 1–8.
Li, C., Loui, A., and Chen, T. (2010). Towards aesthetics:
a photo quality assessment and photo selection sys-
tem. In Proceedings of the international conference
on Multimedia, pages 10–13.
Lienhard, A., Reinhard, M., Caplier, A., and Ladret, P.
(2014). Photo Rating of Facial Pictures based on Im-
age Segmentation. In Proceedings of the 9th Int. Conf.
on computer Vision Theory and Applications, pages
329–336, Lisbonne, Portugal.
Luo, Y. and Tang, X. (2008). Photo and video quality
evaluation: Focusing on the subject. Computer Vi-
sionECCV 2008, pages 386–399.
Males, M., Hedi, A., and Grgic, M. (2013). Aesthetic qual-
ity assessment of headshots. In 55th International
Symposium ELMAR, number September, pages 25–
27.
Marchesotti, L. and Perronnin, F. (2012).
´
Evaluation au-
tomatique de la qualit
´
e esth
´
etique des photographies
`
a l’aide de descripteurs d’images g
´
en
´
eriques. In Re-
connaissance des Formes et Intelligence Artificielle
(RFIA).
Murray, N., Marchesotti, L., and Perronnin, F. (2012).
AVA: A large-scale database for aesthetic visual anal-
ysis. Computer Vision and Pattern Recognition, pages
2408–2415.
Poga
ˇ
cnik, D., Ravnik, R., Bovcon, N., and Solina, F.
(2012). Evaluating photo aesthetics using machine
learning. In Data Mining and Data Warehouses, pages
4–7.
Robnik-
ˇ
Sikonja, M. and Kononenko, I. (2003). Theoretical
and empirical analysis of ReliefF and RReliefF. Ma-
chine learning, 53:23–69.
Tang, X., Luo, W., and Wang, X. (2013). Content-Based
Photo Quality Assessment. IEEE Transactions on
Multimedia, 15(8):1930–1943.
Tong, Y., Konik, H., Cheikh, F. A., and Tremeau, A.
(2010). Full reference image quality assessment based
on saliency map analysis. Journal of Imaging Science
and Technology, 54(3):1–21.
Viola, P. and Jones, M. (2001). Rapid object detection
using a boosted cascade of simple features. In Pro-
ceedings of the IEEE Computer Society Conference on
Computer Vision and Pattern Recognition., volume 1,
pages I–511–I–518. IEEE Comput. Soc.
Willis, J. and Todorov, A. (2006). Making Up Your Mind
After a 100-Ms Exposure to a Face. Psychological
science, 17(7):592–598.
Wong, L.-k. and Low, K.-l. (2009). Saliency-enhanced im-
age aesthetics class prediction. In 16th IEEE Interna-
tional Conference on Image Processing, pages 997–
1000. Ieee.
VISAPP2015-InternationalConferenceonComputerVisionTheoryandApplications
552