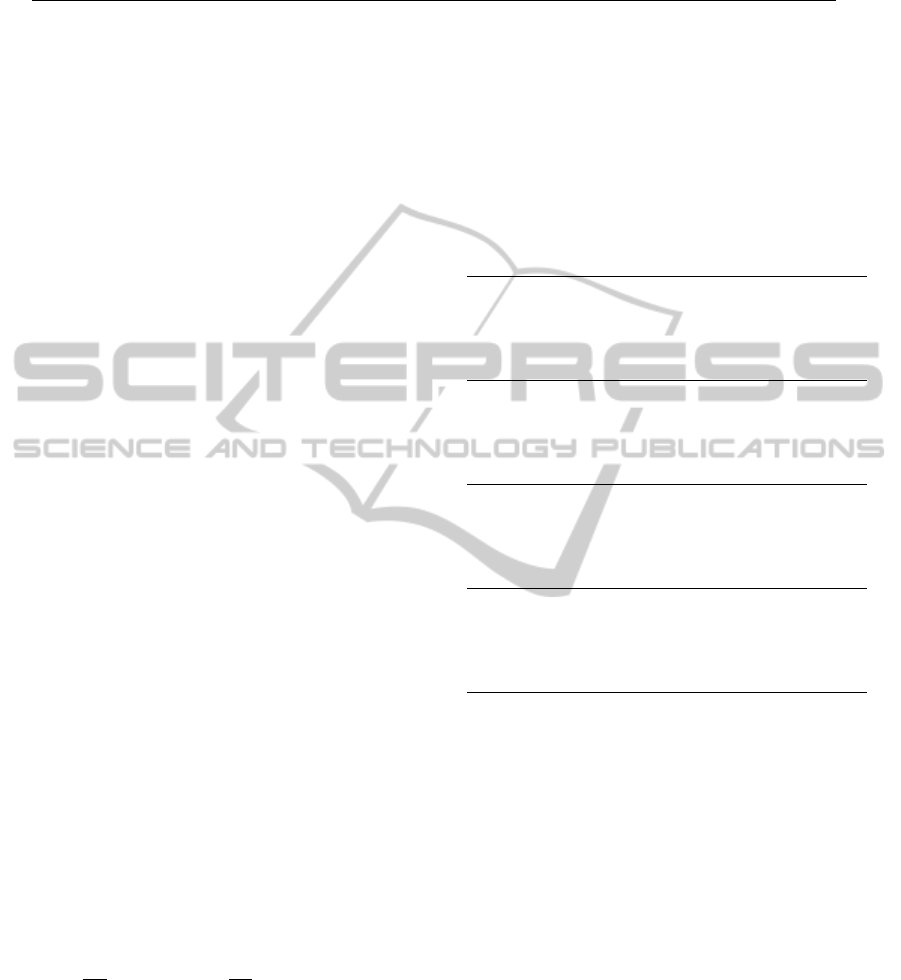
Table 1: Salient information of the 5 considered mosaic images.
Mosaic image Size |T
I
| |I| µ
|T |
ρ
|T |
[%] ρ
∅
[%] µ
I
T
µ
O
T
Church 793 × 1031 855 0.82 × 10
6
543.5 59.5 43.2 39.2 806.9
Museum 1951 × 2386 135 4.66 × 10
6
21451.8 40.1 37.8 13.8 201.0
Bird 942 × 704 717 0.66 × 10
6
530.0 58.0 42.7 54.3 618.2
Flower 1097 × 1872 546 2.05 × 10
6
2646.0 41.8 29.6 42.6 315.5
University 438 × 340 134 0.15 × 10
6
666.1 70.3 40.1 35.1 962.3
University. This is a portion of a mosaic forming the
floor in the main building of our campus, which
dates back to the 1938. This mosaic is, in several
points, quite deteriorated due to pounding.
Table 1 shows the values of the hardness estima-
tors indexes (leftmost 4 columns) for the 5 mosaic im-
ages, along with width and height of the image, the
number |T
I
| of tiles in the corresponding ground truth,
the size of the image |I|, and the average size of a tile
µ
|T |
.
3.3 Results and Discussion
We applied each method to each mosaic image, with
several values for the main method parameter (total-
ing 603 method applications) and obtained the corre-
sponding segmentations: with respect to the main pa-
rameter value, we sampled more densely those inter-
vals in which the performance appeared to be better.
We then computed the performance metrics presented
in Section 2.
With the aim to harmonize the results and to easily
compare the performances of different algorithm, we
chose to scale the main parameter of each method (as
briefly identified and described above) by the effec-
tive number of tiles in each mosaic, when appropriate.
Indeed, the parameters of TP, ERSS and SL methods
are the desired numbers of superpixels, hence scaling
the parameters allows for performance comparison of
every method applied on the full set of available mo-
saics. Instead, considering the other two algorithms
(TOS and SLIC), the parameters are related to dif-
ferent mosaic information and the scaling by the ef-
fective tiles number would be useless. More in de-
tail, let p be the main parameter: we set p := α for
TOS, p :=
l
w
l
h
|T
I
|
for SL, p :=
K
|T
I
|
for TP and ERSS,
and p := w for SLIC. For SL, we experimented, for
each mosaic image, with l
w
, l
h
values consistent with
the width/height ratio of the image.
The results are shown graphically in Figure 2 and
are summarized in Table 2. Figure 2 consists of 20
charts, one row per method: the first column (left)
shows the F-measure vs. the main parameter, the sec-
ond column (center) shows the count error vs. the
main parameter, and the third column (right) shows
Table 2: Performance metrics of the segmentation methods
obtained on the mosaic images (MI) using the main param-
eter value (third column) which led to the best F-measure.
For each mosaic image, the best value for the count error
and the best value for the F-measure are highlighted.
MI Method p Count Prec Rec Fm
Church
TOS 4.00 0.54 56.4 71.9 63.2
SL 1.08 0.08 35.3 71.1 47.2
TP 2.39 1.54 63.5 63.8 63.7
ERSS 2.83 1.83 51.2 39.3 44.4
SLIC 12.83 0.25 47.5 83.8 60.6
Museum
TOS 6.00 0.14 64.4 87.3 74.1
SL 1.07 0.07 52.3 91.7 66.6
TP 1.73 0.84 60.8 60.3 60.6
ERSS 2.16 1.18 48.0 39.5 43.3
SLIC 5.00 7.03 73.9 80.6 77.1
Bird
TOS 4.00 0.03 52.8 81.7 64.2
SL 1.31 0.31 40.4 68.6 50.9
TP 2.14 1.28 62.9 68.4 65.5
ERSS 2.94 1.94 55.3 39.9 46.3
SLIC 12.00 0.32 50.6 84.8 63.4
Flower
TOS 4.00 0.06 49.4 67.8 57.2
SL 0.99 0.01 37.1 63.1 46.7
TP 1.72 0.83 67.4 63.5 65.4
ERSS 2.75 1.75 60.2 35.3 44.5
SLIC 6.00 1.74 63.4 70.4 66.8
University
TOS 4.00 0.90 63.2 78.5 70.1
SL 1.04 0.04 44.0 88.6 58.8
TP 1.90 1.78 67.0 68.9 67.9
ERSS 2.99 1.99 52.6 40.9 46.0
SLIC 8.00 0.33 54.5 87.9 67.3
F-measure vs. count error. Charts of the first two
columns show one line per mosaic image. Table 2
shows the performance metrics obtained by the meth-
ods on the mosaic images, using only the main pa-
rameter value which led to the best F-measure.
It can be seen that the best (largest) values in terms
of F-measure are obtained with TOS and SLIC meth-
ods. In particular, these methods achieve 74.1% and
77.1% F-measure for the Museum image—best val-
ues for ERSS, TP and SL are respectively 46.3%,
67.9% and 66.6% on the Bird, University and Mu-
seum images. This finding is confirmed by Table 2,
which highlights, for each mosaic, the best values ob-
tained for the count error and the F-measure: concern-
ing the latter, SLIC performs best on 2 images, TP on
2 and TOS on the last image; TOS is the second best
VISAPP2015-InternationalConferenceonComputerVisionTheoryandApplications
134