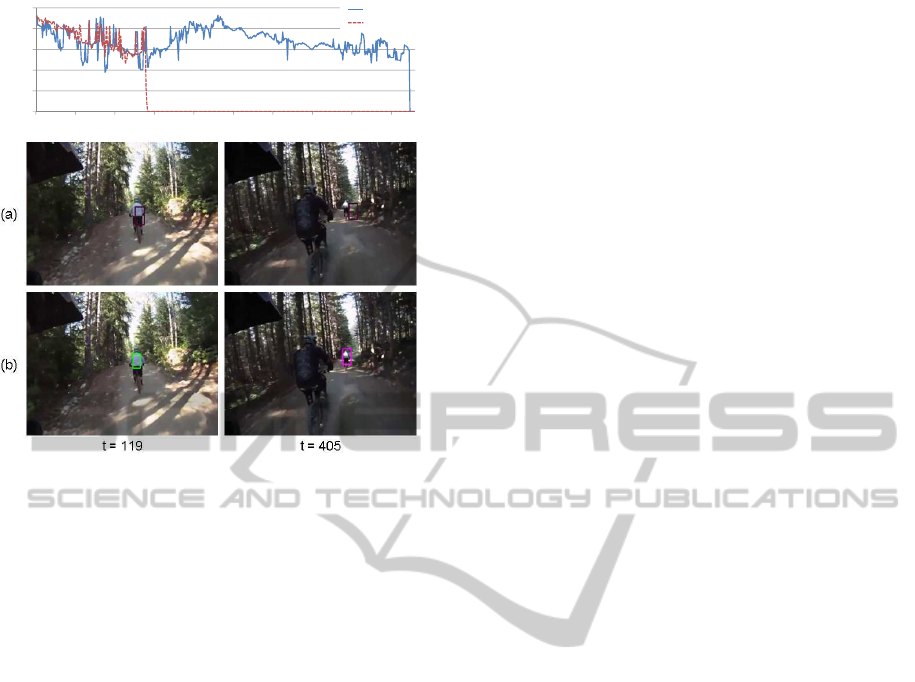
!"
!#
!$
!%
&
& '& & & &'& " & "'& ( & ('& # & #'&
!"#$%&
%'(&
)*+,-./01
232
Figure 4: Comparison of results on the “dh” video for (a)
Fusion of Features (FoF), (b) the proposed method (PM)
where (pink: Haar, blue: HOG, green: HOC Tracker).
6 CONCLUSION
In this paper, we presented a simple method to select
from a pool of trackers the most suitable one. It in-
tegrates a spatial and temporal coherence criterion, a
consistent confidence evaluation for tracker selection
and a selective update strategy. We used OAB-based
trackers with simple low-level features. Experimen-
tal results demonstrate that, even in very challenging
sequences, the proposed method improves the overall
robustness and outperforms classical tracker combi-
nation strategies.
Future work will concentrate on introducing new
and better performing trackers also introducing more
low-level features (e.g. motion) to complete the set of
trackers and achieve a best theoretical selection rate
of 100% (c.f. Table 1).
REFERENCES
Avidan, S. (2007). Ensemble tracking. IEEE Trans. on
PAMI, 29(2):261–271.
Badrinarayanan, V., Perez, P., Le Clerc, F., and Oisel, L.
(2007). Probabilistic color and adaptive multi-feature
tracking with dynamically switched priority between
cues. In Proc. of ICCV.
Bailer, C., Pagani, A., and Stricker, D. (2014). A superior
tracking approach: Building a strong tracker through
fusion. In Proc. of ECCV, pages 170–185.
Collins, R. T. and Liu, Y. (2005). On-line selection of dis-
criminative tracking features. IEEE Trans. on PAMI,
27(10):1631–1643.
Dalal, N. and Triggs, B. (2005). Histograms of oriented
gradients for human detection. In Proc. of CVPR.
Duffner, S., Odobez, J.-M., and Ricci, E. (2009). Dynamic
partitioned sampling for tracking with discriminative
features. In Proc. of BMVC, London, UK.
Freund, Y. and Schapire, R. (1997). A decision-theoretic
generalization of on-line learning and an application
to boosting. Journal of Computer and System Sci-
ences, 55(1):119–139.
Grabner, H. and Bischof, H. (2006). On-line boosting and
vision. In Proc. of CVPR, pages 260–267.
Grabner, H., Grabner, M., and Bischof, H. (2006). Real-
time tracking via on-line boosting. In Proc. of BMVC,
pages 47–56.
Hua, C., Wu, H., Chen, Q., Wada, T., and City, W. (2006).
A pixel-wise object tracking algorithm with target and
background sample. In Proc. of ICPR.
Kittler, J., Hatef, M., Duin, R. P. W., and Matas, J. (1998).
On combining classifiers. IEEE Trans. on PAMI,
20(3):226–239.
Kristan, M., Cehovin, L., Pflugfelder, R., Nebehay, G., Fer-
nandez, G., Matas, J., and et al. (2013). The Visual
Object Tracking VOT2013 challenge results. In Proc.
of ICCV (Workshops).
Kwon, J. and Lee, K. (2010). Visual tracking decomposi-
tion. In Proc. of CVPR, pages 1269–1276.
Kwon, J. and Lee, K. (2011). Tracking by sampling track-
ers. In Proc. of ICCV.
Leichter, I., Lindenbaum, M., and Rivlin, E. (2006). A gen-
eral framework for combining visual trackers – “black
boxes” approach. IJCV, 67(3):343–363.
Maggio, E., Smeraldi, F., and Cavallaro, A. (2007). Adap-
tive multifeature tracking in a particle filtering frame-
work. IEEE on Circuits and Systems for Video Tech-
nology, 17(10):1348–1359.
Moreno-Noguer, F., Sanfeliu, A., and Dimitris, S. (2008).
Dependent multiple cue integration for robust track-
ing. IEEE Trans. on PAMI, 30(4):670–685.
Nickel, K. and Stiefelhagen, R. (2008). Dynamic integra-
tion of generalized cues for person tracking. In Proc.
of ECCV, pages 514–526.
Perez, P., Vermaak, J., and Blake, A. (2004). Data fu-
sion for visual tracking with particles. Proc. of IEEE,
93(3):495–513.
Smeulder, W. M. A., Dung, M. C., Cucchiara, R., Calderara,
S., Deghghan, A., and Shah, M. (2014). Visual track-
ing: an experimental survey. IEEE Trans. on PAMI.
Stalder, S., Grabner, H., and Gool, L. V. (2009). Beyond
semi-supervised tracking: Tracking should be as sim-
ple as detection, but not simpler than recognition. In
ICCV (WS on On-line Comp. Vis.)), pages 1409–1416.
Stenger, B., Woodley, T., and Cipolla, R. (2009). Learning
to track with multiple observers. In Proc. of CVPR.
Triesch, J. and v. d. Malsburg, C. (2001). Democratic in-
tegration: Self-organized integration of adaptive cues.
Neural Computation, 13(9):2049–2074.
CoherentSelectionofIndependentTrackersforReal-timeObjectTracking
591