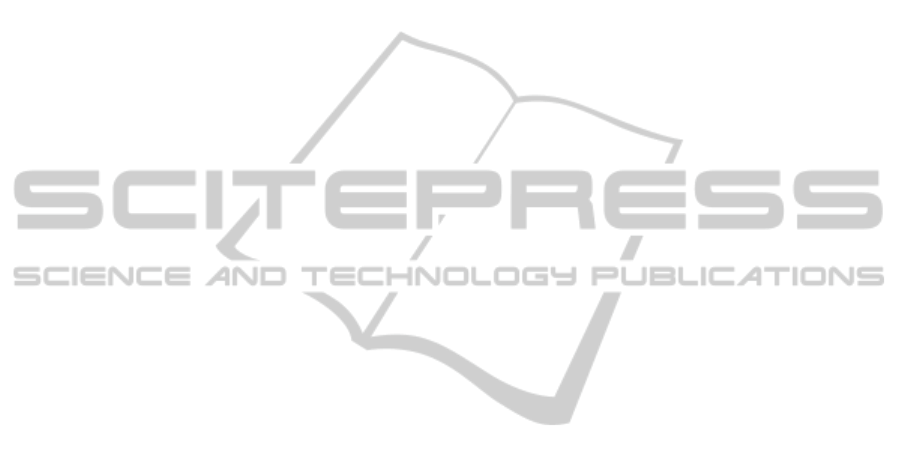
sults for translatory motion estimation compared with
synthetic ground truth. However, the aperture prob-
lem occurred in the slanted bar case in which the mo-
tion was estimated orthogonal to the contrast. Nev-
ertheless, a proper motion estimation was achieved at
the bar ends. The aperture problem can be overcome
via the feedback of a larger receptivefield (MT) (Bay-
erl and Neumann, 2004) or using IOC mechanism for
translatory motion.
The error value increased in rotational motion
cases due to the limited number of estimated direc-
tions compared with the ground truth. This drawback
could be overcome by increasing the estimated direc-
tions of our model. The spatial low resolution of the
DVS sensor has unfavorable impact on several loca-
tions of the complex image case due to the spatial
aliasing problem which leads to spurious estimations.
The size of the temporal sampling interval can af-
fect the motion estimation results in which smaller
temporal window size gives better estimation. This
smaller window can capture more motion details
since it accumulates the occurred events immediately
and transcribes their motions instantaneously. As a
consequence, the subtle information can be main-
tained. The speed sensitivity of our model was evalu-
ated in a rotational motion and bouncing ball cases,
where the speed of different locations were calcu-
lated. In general, the results reveal that our model is
sensitive to different speeds. However, further inves-
tigation should be carried out to verify the estimated
speed value comparing with the actual speed.
Balancing the activations of the individual cells is
achieved by the normalization process. This process
operates in the spatial and directional domain. Con-
sequently, the overall cells activities is adjusted in a
local region.
Our results were compared with (Tschechne et al.,
2014b) by estimating the mean angular error for both
models. The comparison reveals that our approach
leads to estimate the motion with smaller error than
the proposed model in (Tschechne et al., 2014b).
Our model can be extended using a variant tempo-
ral sampling window during one motion scenario, in
which the window size changes dynamically relative
to the speed of the motion. This will enable the model
to be autonomously adaptive for fast and slow speed
motion.
ACKNOWLEDGEMENTS
LIAK has been supported by grants from the Min-
istry of Higher Education and Scientific Research
(MoHESR) Iraq and from the German Academic
Exchange Service (DAAD). HN. acknowledges sup-
port from DFG in the Collaborative Research Cen-
ter SFB/TR ( A companion technology for cognitive
technical systems). The authors would like to thank
S. Tschechne and R. Sailer for providing the compar-
ison materials. We thank M. Schels for his help in
recording biological motion.
REFERENCES
Adelson, E. and Bergen, J. (1985). Spatiotemporal energy
models for the perception of motion. Journal of the
Optical Society of America, 2(2):90–105.
Adelson, E. and Movshon, J. (1982). Phenome-
nal coherence of moving visual pattern. Nature,
300(5892):523–525.
Bayerl, P. and Neumann, H. (2004). Disambiguating vi-
sual motion through contextual feedback modulation.
Neural Computation, 16(10):2041–2066.
Benosman, R., Leng, S., Clercq, C., Bartolozzi, C., and M.,
S. (2012). Asynchronous framless event-based opti-
clal flow. Neural Networks, 27:32–37.
Bouecke, J., Tlapale, E., Kornprobst, P., and Neumann, H.
(2010). Neural mechanisms of motion detection, in-
tegration, and segregation: from biology to artificial
image processing systems. EURASIP Journal on Ad-
vances in Signal Processing.
Brosch, T. and Neumann, H. (2014). Computing with a
canonical neural circuits model with pool normaliza-
tion and modulating feedback. Neural Computation
(in press).
Brox, T., Bruhn, A., Papenberg, N., and Weickert, J. (2004).
High accuracy optical flow estimation based on a the-
ory for warping. In Proc.8th European Conference on
Computer Vision, Springer LNCS 3024, T. Pajdle and
J. Matas(Eds), (prague, nRepublic), pages 25–36.
Caplovitz, G., Hsieh, P., and Tse, P. (2006). Mechanisms
underlying the perceived angular velocity of a rigidly
rotating object. Vision Reseach., 46(18):2877–2893.
Carandini, M. and Heeger, D. J. (2012). Normalization as
a canonical neural computation. Nature Reviews Neu-
roscience, 13:51–62.
Challinor, K. L. and Mather, G. (2010). A motion-
energy model predicts the direction discrimination
and mae duration of two-stroke apparent motion at
high and low retinal illuminance. Vision Research,
50(12):1109–1116.
Dayan, P. and Abbot, L. F. (2001). Theoretical neuro-
science. MIT Press,Cambridge, Mass, USA,.
De Valois, R., Cottarisb, N. P., Mahonb, L. E., Elfara, S. D.,
and Wilsona, J. A. (2000). Spatial and temporal re-
ceptive fields of geniculate and cortical cells and di-
rectional selectivity. Vision Research, 40(27):3685–
3702.
Delbruck, T. and Lichtsteiner, P. (2008). Fast sensory motor
control based on event-based hybrid neuromorphic-
procedural system. IEEE International Symposiom on
circuit and system, pages 845 – 848.
Bio-inspiredModelforMotionEstimationusinganAddress-eventRepresentation
345