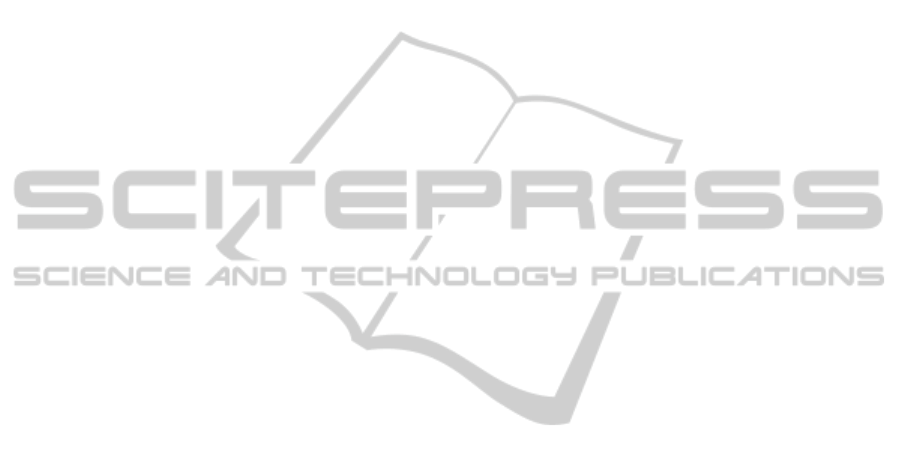
6 CONCLUSIONS
In this paper we addressed two usually unrelated re-
search fields: unsupervised segmentation evaluation
and automatic image annotation. Our proposal of in-
cluding semantic measures in the segmentation evalu-
ation process and the combination of several individ-
ual evaluators displayed better results than the most
relevant measures found in the literature. We also
showed that the measures that evaluate a segmenta-
tion more similar to humans, are not the best for se-
lecting partition levels to perform automatic recogni-
tion tasks. Therefore, in our opinion, more efforts
should be devoted to develop segmentation evaluation
measures that work better for automatic image anno-
tation, instead of focusing on the best segmentation
output for humans.
The final results of the annotation process showed
that selecting ”good” levels at the beginning provides
better annotation accuracy. As future work, we plan
to include saliency maps in the segmentation evalu-
ation process, trying to find partitions that preserve
distinctive objects or parts.
ACKNOWLEDGEMENTS
This work was supported in part by CONACYT
project 215546.
REFERENCES
Arbelaez, P., Hariharan, B., Gu, C., Gupta, S., Bourdev,
L. D., and Malik, J. (2012). Semantic segmentation
using regions and parts. In CVPR, pages 3378–3385.
IEEE.
Csurka, G., Larlus, D., and Perronnin, F. (2013). What is a
good evaluation measure for semantic segmentation?
In 24th British Machine Vision Conference (BMVC),
University of Bristol, United Kingdom.
Dogra, D. P., Majumdar, A. K., and Sural, S. (2012). Eval-
uation of segmentation techniques using region area
and boundary matching information. J. Vis. Comun.
Image Represent., 23(1):150–160.
Gould, S., Fulton, R., and Koller, D. (2009). Decomposing
a scene into geometric and semantically consistent re-
gions. In ICCV, pages 1–8. IEEE.
Haxhimusa, Y. and Kropatsch, W. G. (2004). Segmenta-
tion graph hierarchies. In Proceedings of Joint In-
ternational Workshops on Structural, Syntactic, and
Statistical Pattern Recognition S+SSPR 2004, volume
LNCS 3138, pages 343–351. Springer, Berlin Heidel-
berg, New York.
Huang, Q., Han, M., Wu, B., and Ioffe, S. (2011). A hierar-
chical conditional random field model for labeling and
segmenting images of street scenes. 2013 IEEE Con-
ference on Computer Vision and Pattern Recognition,
0:1953–1960.
Khan, J. F. and Bhuiyan, S. M. (2014). Weighted entropy
for segmentation evaluation. Optics and Laser Tech-
nology, 57(0):236 – 242. Optical Image Processing.
Morales-Gonz´alez, A. and Garc´ıa-Reyes, E. B. (2013).
Simple object recognition based on spatial relations
and visual features represented using irregular pyra-
mids. Multimedia Tools Appl., 63(3):875–897.
Morales-Gonz´alez, A., Reyes, E. B. G., and Sucar, L. E.
(2013). Improving image segmentation for boosting
image annotation with irregular pyramids. In CIARP
(1), volume 8258 of LNCS, pages 399–406. Springer.
Olson, C. R. (2001). Object-based vision and attention in
primates. Current Opinion in Neurob., 11:171–179.
Russakovsky, O., Deng, J., Krause, J., Berg, A., and Li, F.
(2014). Results of ILSVRC2013. http://www.image-
net.org/challenges/LSVRC/2013/results.php.
Russell, C., Ladicky, L., Kohli, P., and Torr, P. H. S. (2014).
Associative hierarchical random fields. IEEE Trans-
actions on Pattern Analysis and Machine Intelligence,
36(6):1–1.
Song, Y.-Z., Arbelaez, P., Hall, P. M., Li, C., and Balikai,
A. (2010). Finding semantic structures in image hi-
erarchies using laplacian graph energy. In ECCV (4),
volume 6314 of LNCS, pages 694–707. Springer.
van de Sande, K. E. A., Uijlings, J. R. R., Gevers, T., and
Smeulders, A. W. M. (2011). Segmentation as selec-
tive search for object recognition. In Proceedings of
ICCV ’11, pages 1879–1886. IEEE Computer Society.
Yang, P., Liu, W., Zhou, B. B., Chawla, S., and Zomaya,
A. Y. (2013). Ensemble-based wrapper methods for
feature selection and class imbalance learning. In
PAKDD (1), volume 7818 of LNCS, pages 544–555.
Springer.
Zankl, G., Haxhimusa, Y., and Ion, A. (2012). Interac-
tive labeling of image segmentation hierarchies. In
DAGM/OAGM Symposium, volume 7476 of LNCS,
pages 11–20. Springer.
Zhang, H., Fritts, J. E., and Goldman, S. A. (2008). Image
segmentation evaluation: A survey of unsupervised
methods. Comput. Vis. Image Underst., 110(2):260–
280.
Zhang, S. and Xie, M. (2013). Beyond sliding windows:
Object detection based on hierarchical segmentation
model. In International Conference on Communica-
tions, Circuits and Systems (ICCCAS), pages 263 –
266. IEEE.
UnsupervisedSegmentationEvaluationforImageAnnotation
155