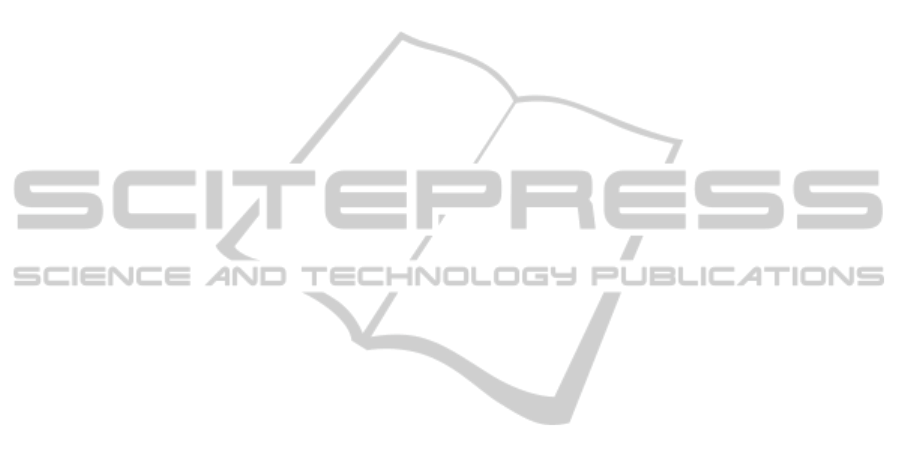
Starting with the static case, the corresponding
MSE performance of the initialization step and the
second deblurring step of UP-SR are reported in Fig-
ure 2 in solid and dashed lines, respectively. In the
simple case where r = 1, the SR resolution problem is
merely a denoising one where the ground truth is es-
timated from 9 noisy measurements. In other words,
the objective is not to increase resolution, and hence
there is no blur due to upsampling. Indeed, as seen in
Figure 2, the solid red line overlaps with the dashed-
dotted black line which corresponds to the theoretical
variance for the odd case obtained using (20). A non-
zero bias is found for r = 2 and r = 4 where the cor-
responding blue and green solid lines are above the
theoretical variance. This suggests a correlation be-
tween motion and upsampling blur as expressed by
the vector u
i
in (15). We note an increased bias for
a larger SR factor r. This is justified by a larger blur
effect due to the dense upsampling and to motion. Fi-
nally, the dashed lines in Figure 2 confirm the perfor-
mance enhancement after applying the optimization
in (10); thus, ensuring an effective deblurring. We
used an exhaustive search to find the best parameters
for Γ. These quantitative results can be appreciated
visually in Figure 4 where the noise level is fixed at
σ = 30 mm. The effective resolution enhancement,
with a SR factor of r = 4, and denoising power of
UP-SR for a static depth scene is seen in 3D in Fig-
ure 4 (i). The average RMSE in 3D is shown in Fig-
ure 4 (l).
In the dynamic case, a similar behaviour has been
observed with some differences related to the local
motion estimation and data type. We can see that even
for the simple case with r = 1, a non-zero bias from
the theoretical variance is found for both the initial
and optimized results, represented by the solid and
dashed red lines in Figure 3, respectively. This bias
is mainly due to the error caused by self-occlusions
and errors in registration. In the case of resolution en-
hancement with SR factors r = 2 and r = 4, we can
see that the non-zero bias in Figure 3 follows the same
behaviour as the one for the static case. The difference
is a smaller shift from the theoretical variance, espe-
cially for low noise levels as can be seen in the corre-
sponding blue and green solid lines. This is directly
related to the data type. Whereas in the dynamic case
we used a simple CAD object (Figure 1 (a)), in the
static case we used a scanned object containing more
geometric details (Figure 1 (b)). Therefore, the down-
sampling process has more effect on the static object
and leads to a larger loss in details, hence a larger bias.
5 CONCLUSION
We have proposed to adapt the affine bias model pro-
posed by (Chatterjee and Milanfar, 2009) to approxi-
mate the bias of a depth multi–frame super–resolution
algorithm using a patch–based representation. Speci-
cally, the Upsampling for Precise Super–Resolution
(UP–SR) algorithm has been considered. With an ad-
ditional step to handle the effect of downsampling,
this derived statistical analysis may be applied to any
multi–frame super–resolution algorithm. The appli-
cation to UP–SR has the advantage that it does not
need to handle downsampling separately because it
directly transfers the super–resolution problem to a
denoising one. We provided a theoretical perfor-
mance analysis of UP–SR in terms of mean square
error, including the variance and the bias terms. We
validated these results experimentally using a syn-
thetic simulation setup. This analysis gave insights
on the effect of the different parameters: noise level,
the number of observed low resolution frames, and
the super–resolution factor. In summary, the perfor-
mance of UP–SR or any multi-frame super–resolution
algorithm increases with the increase of the number of
observations. In the case of dynamic scenes, this per-
formance decreases due to local motions and errors
of registration. In the case of UP–SR, there is an ad-
ditional error due to the upsampling effect. It can be
reduced thanks to the final deblurring phase.
ACKNOWLEDGEMENT
This work was supported by the National Research
Fund (FNR), Luxembourg, under the CORE project
C11/BM/1204105/FAVE.
REFERENCES
3d MLI (2014). http://www.iee.lu/technologies.
Beaulieu, N. and Jiang, S. (2010). Ml estimation of signal
amplitude in laplace noise,. In Global Telecommuni-
cations Conference. IEEE.
Chatterjee, P. and Milanfar, P. (2009). Bias modeling for
image denoising,. In 43rd Asilomar Conference on
Signals, Systems and Computers.
Elad, M. and Feuer, A. (1999). Super-resolution reconstruc-
tion of continuous image sequences,. In Transaction
Pattern Analysis and Machine Intelligence. IEEE.
K. Al Ismaeil, D. Aouada, B. M. B. O. (2013a). Depth
super-resolution by enhanced shift & add,. In 15th In-
ternational Conference on Computer Analysis of Im-
ages and Patterns. Springer.
VISAPP2015-InternationalConferenceonComputerVisionTheoryandApplications
192