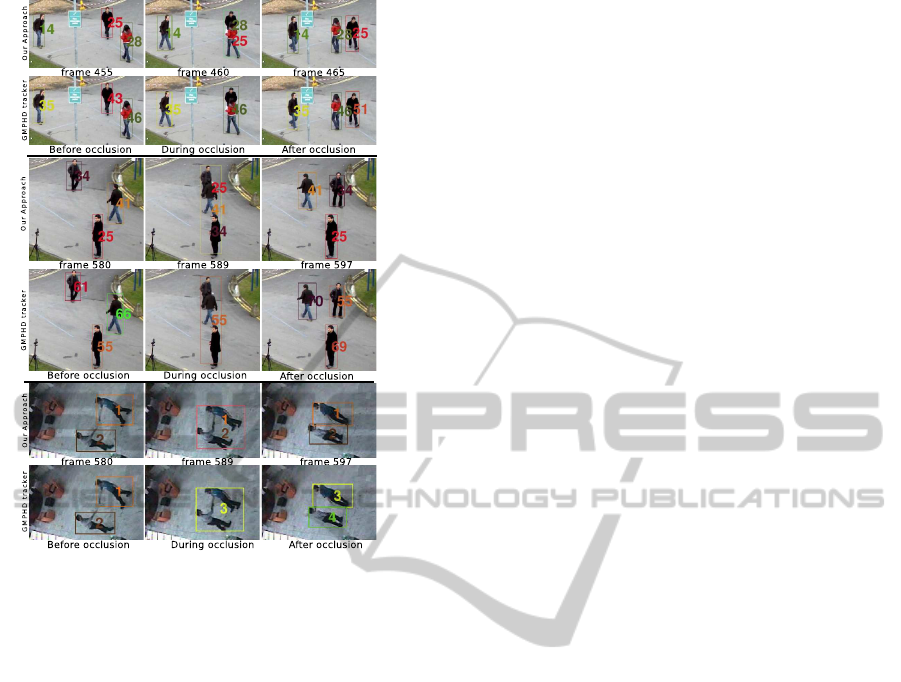
Figure 6: Examples of tracking.
results on PETS2009 and CAVIAR datasets show that
our approach offers a high improvement in estimat-
ing the number of objects and in ensuring a consistent
targets labeling over time, compared the original GM-
PHD. Furthermore, our approach exceeds 60 fps on a
PC. This makes it a good candidate for an embedded
implementation.
The future work consists in implementing our
method on an embedded processor with low comput-
ing resources connected to an image sensor in order
to form a smart camera. The idea is to extend our
approach to a network of smart cameras.
REFERENCES
Blackman, S. (2004). Multiple hypothesis tracking for mul-
tiple target tracking. Aerospace and Electronic Sys-
tems Magazine, IEEE, 19(1):5–18.
Blackman, S. and Popoli, R. (1999). Design and Analysis of
Modern Tracking Systems. Artech House, Norwood,
MA.
caviar(2004). http://homepages.inf.ed.ac.uk/rbf/caviardata1.
Clark, D., Panta, K., and Vo, B.-N. (2006). The gm-phd
filter multiple target tracker. In Information Fusion,
2006 9th International Conference on, pages 1–8.
Dziri, A., Duranton, M., and Chapuis, R. (2014). Low com-
plexity multi-target tracking for embedded systems.
In Information Fusion (FUSION), 2014 17th Interna-
tional Conference on, pages 1–8.
Edman, V., Maria, A., Granstr¨om, K., and Gustafsson, F.
(2013). Pedestrian group tracking using the gm-phd
filter. In Proceedings of the 21st European Signal Pro-
cessing Conference :.
Eiselein, V., Arp, D., Patzold, M., and Sikora, T. (2012).
Real-time multi-human tracking using a probability
hypothesis density filter and multiple detectors. In Ad-
vanced Video and Signal-Based Surveillance (AVSS),
2012 IEEE Ninth International Conference on, pages
325–330.
Geronimo, D., Lopez, A., Sappa, A., and Graf, T. (2010).
Survey of pedestrian detection for advanced driver as-
sistance systems. Pattern Analysis and Machine Intel-
ligence, IEEE Transactions on, 32(7):1239–1258.
Hoseinezhad, R., Vo, B.-N., and Suter, D. (2009). Fast
single-view people tracking. In Cognitive Systems
with Interactive Sensors (COGIS2009).
Lamard, L., Chapuis, R., and Boyer, J.-P. (2012). Dealing
with occlusions with multi targets tracking algorithms
for the real road context. In Intelligent Vehicles Sym-
posium (IV), 2012 IEEE, pages 371–376.
Ma, N., Bailey, D., and Johnston, C. (2008). Optimised sin-
gle pass connected components analysis. In ICECE
Technology, 2008. FPT 2008. International Confer-
ence on, pages 185–192.
Mahler, R. (2003). Multitarget bayes filtering via first-order
multitarget moments. Aerospace and Electronic Sys-
tems, IEEE Transactions on, 39(4):1152–1178.
Panta, K., Clark, D., and Vo, B.-N. (2009). Data association
and track management for the gaussian mixture prob-
ability hypothesis density filter. Aerospace and Elec-
tronic Systems, IEEE Transactions on, 45(3):1003–
1016.
pets2009 (2009). http://cs.binghamton.edu/ mrl-
data/pets2009.html.
Roller, D., Daniilidis, K., and Nagel, H. (1993). Model-
based object tracking in monocular image sequences
of road traffic scenes. International Journal of Com-
puter Vision, 10(3):257–281.
Vezzani, R., Baltieri, D., and Cucchiara, R. (2013). People
reidentification in surveillance and forensics: A sur-
vey. ACM Comput. Surv., 46(2):29:1–29:37.
Vijverberg, J. A., Koeleman, C. J., and With, P. H. N. d.
(2009). Tracking rectangular targets in surveillance
videos with the gm-phd filter. In Proceedings of
the 30-th Symposium on Information Theory in the
Benelux, pages 177–184.
Vo, B.-N. and Ma, W.-K. (2006). The gaussian mixture
probability hypothesis density filter. Signal Process-
ing, IEEE Transactions on, 54(11):4091–4104.
Wang, L., Hu, W., and Tan, T. (2003). Recent develop-
ments in human motion analysis. Pattern Recognition,
36(3):585 – 601.
Wang, Y.-D., Wu, J.-K., Kassim, A., and Huang, W.-M.
(2006). Tracking a variable number of human groups
in video using probability hypothesis density. In Pat-
tern Recognition, 2006. ICPR 2006. 18th Interna-
tional Conference on, volume 3, pages 1127–1130.
Wu, J. and Hu, S. (2010). Phd filter for multi-target visual
tracking with trajectory recognition. In Information
Fusion (FUSION), 2010 13th Conference on, pages
1–6.
Yilmaz, A., Javed, O., and Shah, M. (2006). Object track-
ing: A survey. ACM Comput. Surv., 38(4).
LowComplexityMulti-objectTrackingSystemDealingwithOcclusions
201