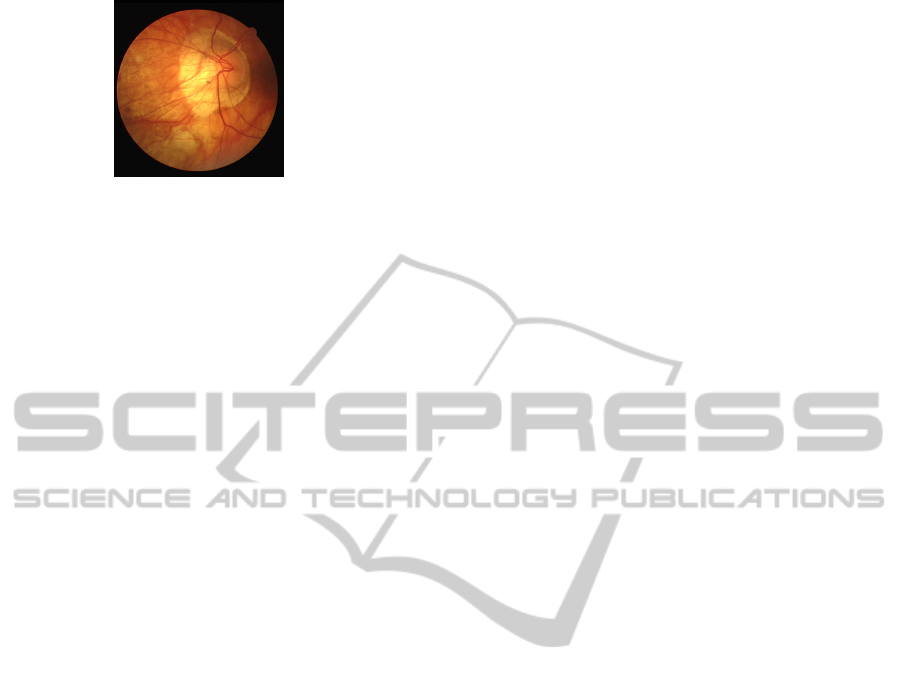
Figure 6: This picture shows an abnormal optic disc that
could not yet be properly segmented by the algorithm.
these algorithms in segmentation of retinal images
segmentation, as well the very good accuracy average
obtained, when comparing with other studies devel-
oped on the same database.
The optic disc and the vessels are fundamental for
the understanding and analysis of ocular fundus im-
ages. Our objective was to define two algorithms that
will be used as a first stage in image registration to
identify false positives in pathology detection and for
automatic classification of detected pathologies.
ACKNOWLEDGEMENTS
The authors thank Fundo Mackenzie de Pesquisa
(Mackpesquisa) from the Universidade Presbiteriana
Mackenzie for the financial support for this research.
REFERENCES
Addison, P. S. (2005). Wavelet transforms and the ECG: a
review. Physiological measurement, 26(5):R155–99.
Burrus, C. S., Gopinath, R. A., and Guo, H. (1998). In-
troduction to Wavelets and Wavelet Transforms: A
Primer.
Florack, L. M., ter Haar Romeny, B. M., Koenderink, J. J.,
and Viergever, M. a. (1992). Scale and the differen-
tial structure of images. Image and Vision Computing,
10(6):376–388.
Frangi, A. F., Niessen, W. J., Vincken, K. L., and Viergever,
M. A. (1998). Multiscale vessel enhancement filtering
1 Introduction 2 Method. 1496:130–137.
Fraz, M. M., Remagnino, P., Hoppe, a., Uyyanonvara, B.,
Rudnicka, a. R., Owen, C. G., and Barman, S. a.
(2012). Blood vessel segmentation methodologies in
retinal images–a survey. Computer methods and pro-
grams in biomedicine, 108(1):407–33.
Haralick, R. M. and Sternberg, S. R. (1987). Image Analy-
sis Morphology. (4):532–550.
Kande, G. B., Subbaiah, P. V., and Savithri, T. S. (2010).
Unsupervised Fuzzy Based Vessel Segmentation In
Pathological Digital Fundus Images. J. Med. Syst.,
34(5):849–858.
Koenderink, J. J. (1984). Biological Cybernetics The Struc-
ture of Images. 370:363–370.
Lupas, C. A., Tegolo, D., and Trucco, E. (2010). FABC
: Retinal Vessel Segmentation Using AdaBoost.
14(5):1267–1274.
Mallat, S. G. (1989). Theory for multiresolution signal de-
composition: the wavelet representation. IEEE Trans-
actions on Pattern Analysis and Machine Intelligence,
11:674–693.
Mar
´
ın, D., Aquino, A., Geg
´
undez-arias, M. E., and Bravo,
J. M. (2010). Pr E oo f Pr E oo f. pages 1–13.
Niemeijer, M., Staal, J., Ginneken, B. V., Loog, M., and
Abr, M. D. Comparative study of retinal vessel
segmentation methods on a new publicly available
database.
Ricci, E. and Perfetti, R. (2007). Retinal Blood Vessel Seg-
mentation Using Line Operators and Support Vector
Classification. Medical Imaging, IEEE Transactions
on, 26(10):1357–1365.
Rossant, F., Badellino, M., Chavillon, A., Bloch, I., and
Paques, M. (2011). A Morphological Approach for
Vessel Segmentation in Eye Fundus Images, with
Quantitative Evaluation. Journal of Medical Imaging
and Health Informatics, 1(1):42–49.
Shapiro, L. and Stockman, G. (2001). Computer Vision,
volume 2004.
Sinthanayothin, C., Boyce, J. F., Cook, H. L., and
Williamson, T. H. (1999). Automated localisation of
the optic disc, fovea, and retinal blood vessels from
digital colour fundus images. British Journal of Oph-
thalmology, 83(8):902–910.
Staal, J., Member, A., Abr
`
amoff, M. D., Niemeijer, M.,
Viergever, M. A., Ginneken, B. V., and Detection,
A. R. (2004). Ridge-Based Vessel Segmentation in
Color Images of the Retina. 23(4):501–509.
Witkin, A. P. (1983). Scale-space Filtering. In Proceedings
of the Eighth International Joint Conference on Arti-
ficial Intelligence - Volume 2, IJCAI’83, pages 1019–
1022, San Francisco, CA, USA. Morgan Kaufmann
Publishers Inc.
Zana, F. and Klein, J. C. (2001). Segmentation of vessel-
like patterns using mathematical morphology and cur-
vature evaluation. IEEE transactions on image pro-
cessing : a publication of the IEEE Signal Processing
Society, 10(7):1010–9.
VISAPP2015-InternationalConferenceonComputerVisionTheoryandApplications
622