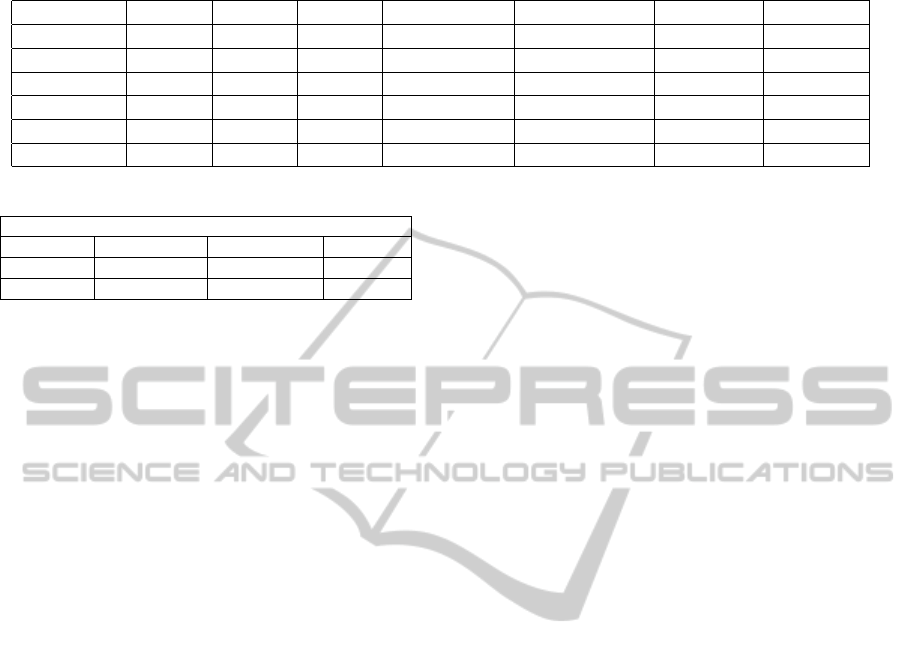
Table 2: Results table - Original and Modified Optical Flow.
Seg./Route Prec. Recall F1 Prec. (Mod.) Recall (Mod.) F1 (Mod.) Gain (F1)
S01/A-A 59.52% 48.10% 53.20% 71.43% 75.47% 73.39% 37.98%
S01/B-B 73.33% 33.00% 45.51% 86.95% 58.25% 69.77% 53.28%
S02/A-A 70.83% 50.07% 59.13% 82.81% 75.71% 79.10% 33.78%
S02/B-B 62.10% 28.97% 39.49% 70.67% 50.96% 59.21% 49.95%
S03/A-A 62.38% 48.50% 54.54% 74.51% 74.51% 74.51% 36.60%
S03/B-B 58.97% 27.06% 37.09% 67.24% 47.56% 55.71% 50.19%
Table 3: Results table - StMarc video.
St Marc
Route Groundtruth System result Accuracy
Route A-A 4 4 100.0%
Route B-B 4 4 100.0%
5 CONCLUSION
We presented a hybrid algorithm that combines opti-
cal flow to track points along video frames and fore-
ground detection to reacquire points and update track-
ing information during size variation of objects in
sequence. This mixed solution allows us to mini-
mize problems caused by changes in object size along
video frames.
It is clear that good results in optical flow using
Lucas-Kanade approach highly depends on quality of
initial object segmentation. If foreground detector
fails, all following approaches will also fail because it
will calculate pixel displacement in wrong positions.
By using a hybrid solution that combines optical flow
results with foreground extraction the error is mini-
mized and system accuracy is increased.
ACKNOWLEDGEMENTS
This work was supported by Fapemig, CNPq and
Capes.
REFERENCES
Buch, N., Velastin, S., and Orwell, J. (2011). A review
of computer vision techniques for the analysis of ur-
ban traffic. Intelligent Transportation Systems, IEEE
Transactions on, 12(3):920–939.
Chen, T.-H., Lin, Y.-F., and Chen, T.-Y. (2007). Intelli-
gent vehicle counting method based on blob analysis
in traffic surveillance. In Innovative Computing, In-
formation and Control, 2007. ICICIC ’07. Second In-
ternational Conference on, pages 238–238.
Fawzi M. Al-Naima, H. A. H. (2012). Vehicle traffic con-
gestion estimation based on rfid. volume 4:30.
Fleischer, P., Nelson, A., Sowah, R., and Bremang, A.
(2012). Design and development of gps/gsm based ve-
hicle tracking and alert system for commercial inter-
city buses. In Adaptive Science Technology (ICAST),
2012 IEEE 4th International Conference on, pages 1–
6.
Friedman, N. and Russell, S. (1997). Image segmentation
in video sequences: A probabilistic approach. In Pro-
ceedings of the Thirteenth Conference on Uncertainty
in Artificial Intelligence, UAI’97, pages 175–181, San
Francisco, CA, USA. Morgan Kaufmann Publishers
Inc.
Han, C. and Zhang, Q. (2008). Real-time detection of vehi-
cles for advanced traffic signal control. In Computer
and Electrical Engineering, 2008. ICCEE 2008. Inter-
national Conference on, pages 245–249.
Jinglei, Z. and Zhengguang, L. (2007). A vision-based road
surveillance system using improved background sub-
traction and region growing approach. In Software
Engineering, Artificial Intelligence, Networking, and
Parallel/Distributed Computing, 2007. SNPD 2007.
Eighth ACIS International Conference on, volume 3,
pages 819–822.
Jodoin, J.-P., Bilodeau, G.-A., and Saunier, N. (2014). Ur-
ban tracker: Multiple object tracking in urban mixed
traffic. In Applications of Computer Vision (WACV),
2014 IEEE Winter Conference on, pages 885–892.
Lee, S.-M. and Baik, H. (2006). Origin-destination (o-d)
trip table estimation using traffic movement counts
from vehicle tracking system at intersection. In IEEE
Industrial Electronics, IECON 2006 - 32nd Annual
Conference on, pages 3332–3337.
Pe
˜
nate S
´
anchez, A., Quesada-Arencibia, A., and
Travieso Gonz
´
alez, C. (2012). Real time vehi-
cle recognition: A novel method for road detection.
In Moreno-D
´
ıaz, R., Pichler, F., and Quesada-
Arencibia, A., editors, Computer Aided Systems
Theory – EUROCAST 2011, volume 6928 of Lecture
Notes in Computer Science, pages 359–364. Springer
Berlin Heidelberg.
Roberts, W., Watkins, L., Wu, D., and Li, J. (2008). Vehicle
tracking for urban surveillance. volume 6970, pages
69700U–69700U–8.
Shi, J. and Tomasi, C. (1994). Good features to track. In
Computer Vision and Pattern Recognition, 1994. Pro-
ceedings CVPR ’94., 1994 IEEE Computer Society
Conference on, pages 593–600.
Stauffer, C. and Grimson, W. E. L. (1999). Adaptive
background mixture models for real-time tracking.
In Computer Vision and Pattern Recognition, 1999.
VISAPP2015-InternationalConferenceonComputerVisionTheoryandApplications
606