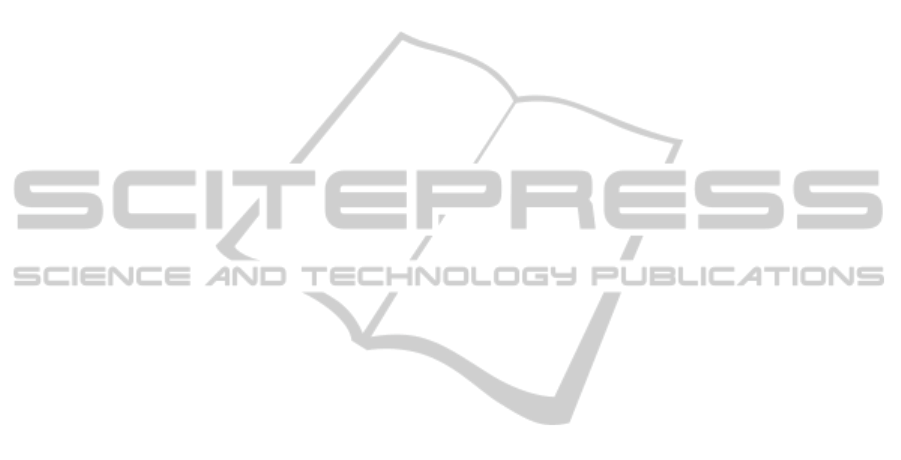
could make frequent small orders. How could a man-
ager be certain that choosing an optimal (s, S) policy
was superior to these alternatives? It turns out that
where demand is uncertain the optimal (s, S) policy
is optimal over any alternative inventory policy that
could be adopted (Scarf, 1960) although the proof was
not available until several years after the paper from
Arrow et.al.
4.2 Perishable Inventory
Some commodities have a fixed life span. We might
immediately think of such things as milk, eggs, bread
and so on but there are other examples: photographic
material, chemical weapons in a store, some short half
life radioactive items. If demand were known with
certainty for these then it would be easy to order in
such a way that the commodity never perishes. The
combination of demand uncertainty and a perishable
(fixed-lifetime) commodity is a more challenging one
than one where the demand is uncertain but the com-
modity has an infinite lifetime.
This forces us to confront not just the optimal or-
der polices in the face of uncertain demand but the
optimal issuing policies as well. The two ends of the
continuum for these are first in first out (FIFO) and
last in first out (LIFO). It seems fairly intuitive that a
FIFO policy is optimal for such inventories and early
proofs for some simple cases were provided in 1958
(Derman and Klein, 1958) while other cases were ad-
dressed later (Pierskalla and Roach, 1972).
The optimal ordering policies for perishable in-
ventories with uncertain demand were investigated
by both Steven Nahmias (Nahmias, 1975) and Brant
Fries (Fries, 1975). These researchers independently
derived the optimal inventory policy for this case. For
this case the inventory manager need only determine
the optimal quantity S of the commodity to hold. As
soon as the inventory falls below this value it is op-
timal to order replacement from the supplier. This is
known as the (S − 1, S) inventory policy.
4.3 Blood Inventories
Blood inventories are a subclass of perishable inven-
tory. These differ as, in addition to demand, the sup-
ply of blood and blood products is also stochastic.
The earliest relevant work on blood inventories ap-
plied known industrial inventory models to the prob-
lem (Millard, 1959). Two measures of blood in-
ventory performance were suggested: probability of
shortage and expected outdates. These measures are
still vitally important today. A limitation of this early
research is that it assumed that variation in both de-
mand and supply of blood is attributed to a Poisson
process. Other early work also tends to continue the
use of the a Poisson process for the supply of blood
(Elston and Pickrel, 1963; Prastacos, 1984), how-
ever differing assumptions have been applied to the
demand for blood. These have variously been neg-
ative binomial (Elston and Pickrel, 1963), modified
log normal (Prastacos, 1984) and batch Poisson (Goh
et al., 1993). More recently independent Poisson, or
related, processes have been used to model both sup-
ply and demand (Blake and Hardy, 2014; Abouee-
Mehrizi et al., 2014; Abbasi and Seidmann, 2014).
It is natural to ask how well these assumptions
match data collected from real blood banks. In their
paper, Blake and Hardy give us data from two Cana-
dian blood banks. A comparison is made between the
observed mean and standard deviation of empirical
aggregate inventory data and results obtained by sim-
ulation. While the means are approximately equal, it
is clear that the standard deviations do not match. Site
A, for example, has a mean of 8,197.54 and a standard
deviation of 1,204.15. The simulation for this site is
approximately equal in the mean but the standard de-
viation is 433.10. The real standard deviation is 2.8
times larger. Their assumption of a zero inflated Pois-
son process for supply and demand gives a simulation
which underestimates the true variance of the aggre-
gate inventory.
In a separate example it was shown (Atkinson
et al., 2012) that a simulation of blood bank dona-
tions and hospital transfusions required a coefficient
of variation of 1.32 to minimise the sum of squared
deviations between the empirical and simulated data.
This would not be the case if the donations and trans-
fusions resulted from a Poisson process.
Consideration of the appropriate donation and
transfusion distributions is important as shortages and
outdates occur in the tails of these distributions rather
than at the mean. Underestimation of the standard de-
viation would tend to underestimate the occurrence of
these events.
5 METHODOLOGY
The approach used in this research will set up a math-
ematical framework for modelling of blood inven-
tories. This will include learnings from a system
dynamics approach to modelling blood inventory in
which it is shown that the system itself contains feed-
back loops and delays which can cause volatility in
the donation rate and inventory levels without having
to assume exogenous sources of variation.
ICORES2015-DoctoralConsortium
4