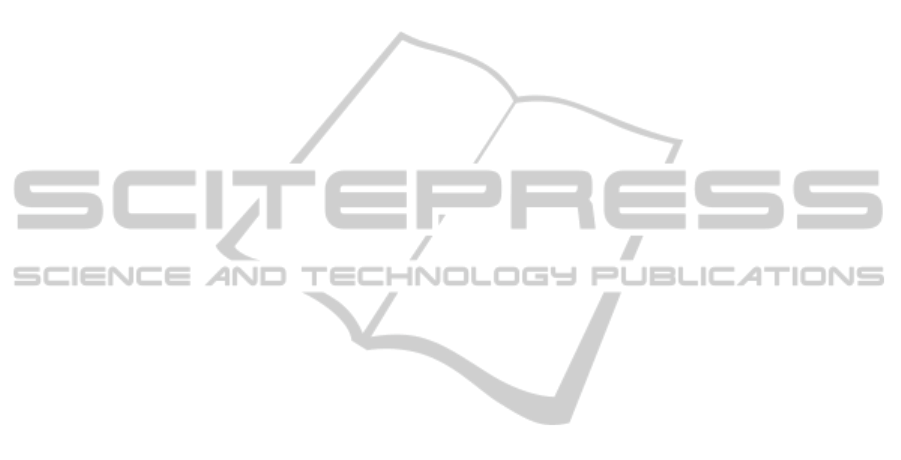
movements in individuals with Parkinson's disease
Exp. Brain Res., 197 (3) (2009), pp. 223–233.
Clarke, R. Human identification in information systems:
Management challenges and public information issues.
Available in http://www.anu.edu.au/people/
Roger.Clarke/ DV/HumanID.html, Dec. 1994 –
Accessed 22/10/2014.
Caples, S. M., A. S. Gami, V. K. Somers Obstructive sleep
apnea. Ann. Intern. Med., 142 (3) (2005), pp. 187–
197.
Drotár, Peter, Jiří Mekyska, Irena Rektorová, Lucia
Masarová, Zdenek Smékal, Marcos Faundez-Zanuy.
Analysis of in-air movement in handwriting: A novel
marker for Parkinson's disease. Comput Methods
Programs Biomed. 2014 Sep 17. pii: S0169-
2607(14)00320-4. doi: 10.1016/j.cmpb.2014.08.007.
Eurostat (2012) Statistics Explained Archive, Vol. 2
Social Statistics (http://epp.eurostat.ec.europa.eu/
cache/ITY_OFFPUB/KS-FM-13-002/EN/KS-FM-13-
002-EN.PDF/) – Accessed 22/10/2014.
El Sayed M., Alsebai A., Salaheldin A., El Gayar N.,
ElHelw M. Ambient and wearable sensing for gait
classification in pervasive healthcare environments.
Proceedings of the 12th IEEE International
Conference on e-Health Networking Applications and
Services (Healthcom); Lyon, France. 1–3 July 2010;
pp. 240–245.
Faundez-Zanuy, M., E. Sesa-Nogueras, J. Roure-Alcobé,
J. Garré-Olmo, K. Lopez-de-Ipiña, J. Solé-Casals,
“Online Drawings for Dementia Diagnose: In-Air and
Pressure Information Analysis”, XIII Mediterranean
Conference on Medical and Biological Engineering
and Computing 2013 IFMBE Proceedings Volume 41,
2014, pp 567-570.
Foley, R. G., A Lamar Miller. The effects of marijuana
and alcohol usage on handwriting, Forensic Sci.Int. 14
(1979) 159-164.
Forbes, K. E., M. F. Shanks, A Venneri. The evolution of
dysgraphia in Alzheimer’s disease, Brain Res.Bull. 63
(2004) 19-24.
Kushida, C. A., M. R. Littner, T. Morgenthaler, C. A.
Alessi, D. Bailey, J. Coleman, L. Friedman, M.
Hirshkowitz, S. Kapen, M. Kramer, T. Lee-Chiong,
D.L. Loube, J. Owens, J.P. Pancer, M. Wise. Practice
parameters for the indications for polysomnography
and related procedures: an update for 2005.
López-de-Ipiña, K., J. B. Alonso, C. M. Travieso, J. Solé-
Casals, H. Egiraun, A. Ezeiza, N.Barroso, M.
Faundez-Zanuy, M. Ecay-Torres, P. Martinez-Lage,
U. Martinez-de-Lizardui, “On the selection of non-
invasive methods based on speech analysis oriented to
automatic Alzheimer Disease diagnosis”, Sensors, vol.
5, pp. 6730-6745, 2013. DOI: 10.3390/s130506730.
López-de-Ipiña, K., J. B. Alonso, J. Solé-Casals, N.
Barroso, P. Henriquez, M. Faundez-Zanuy, C. M.
Travieso, M. Ecay-Torres, P. Martínez-Lage, H.
Eguiraun, “On Automatic Diagnosis of Alzheimer’s
Disease Based on Spontaneous Speech Analysis and
Emotional Temperature”, Cognitive Computation,
2013. 10.1007/s12559-013-9229-9.
López-de-Ipiña, K., J. Solé-Casals, , H. Eguiraun, J.B.
Alonso, C.M. Travieso, A. Ezeiza, N. Barroso, M.
Ecay-Torres, P. Martinez-Lage, B. Beitia. Feature
selection for spontaneous speech analysis to aid in
Alzheimer’s disease diagnosis: A fractal dimension
approach. Computer Speech & Language Available
online 27 August 2014. DOI: 10.1016/
j.csl.2014.08.002.
Mortaza N1, Abu Osman NA, Mehdikhani M. Are the
spatio-temporal parameters of gait capable of
distinguishing a faller from a non-faller elderly? Eur J
Phys Rehabil Med. 2014 Apr 24.
Neils-Strunjas, J., K Groves-Wright, P Mashima, S
Harnish. Dysgraphia in Alzheimer's disease: a review
for clinical and research purposes, J.Speech
Lang.Hear.Res. 49 (2006) 1313-1330.
Patel, Shyamal, Hyung Park, Paolo Bonato, Leighton
Chan and Mary Rodgers. A review of wearable
sensors and systems with application in rehabilitation
Journal of NeuroEngineering and Rehabilitation 2012,
9:21 doi:10.1186/1743-0003-9-21.
Phillips, J. G., RP Ogeil, F Müller. Alcohol consumption
and handwriting: A kinematic analysis, Human
Movement Science. 28 (2009) 619-632.
Rosenblum, S., S Parush, P Weiss. The in Air
Phenomenon: Temporal and Spatial Correlates of the
Handwriting Process, Perceptual & Motor Skills. 96
(2003) 933.
Sasse, M. Angela, and Kat Krol. "Usable biometrics for an
ageing population." (2013): 303-320.
Solé-Casals, J., C. Munteanu, O.Capdevila Martín, F.
Barbé, C. Queipo, J.Amilibia, J. Durán-Cantolla.
Detection of severe obstructive sleep apnea through
voice analysis. Applied Soft Computing, Volume 23,
October 2014, Pages 346–354 DOI:
10.1016/j.asoc.2014.06.017.
Stone E., Skubic M., Rantz M., Abbott C., Miller S.
Average in-home gait speed: Investigation of a new
metric for mobility and fall risk assessment of elders.
Gait & Posture. 2014 Sep 6. DOI: 10.1016/
j.gaitpost.2014.08.019.
Tao, W., Tao Liu, Rencheng Zheng,Hutian Feng. Gait
Analysis Using Wearable Sensors. Sensors (Basel).
2012; 12(2): 2255–2283. doi: 10.3390/s120202255.
Tucha, O., S Walitza, L Mecklinger, D Stasik, T Sontag,
KW Lange. The effect of caffeine on handwriting
movements in skilled writers, Human Movement
Science. 25 (2006) 523-535.
Tucha, O., L. Mecklinger, J. Thome, A. Reiter, G.L.
Alders, H. Sartor, M. Naumann, K.W. Lange
Kinematic analysis of dopaminergic effects on skilled
handwriting movements in Parkinson's disease J.
Neural Transm., 113 (5) (2006), pp. 609–623
http://dx.doi.org/10.1007/s00702-005-0346-9.
Turcot K, Aissaoui R, Boivin K, Pelletier M, Hagemeister
N, de Guise J. A. New accelerometric method to
discriminate between asymptomatic subjects and
patients with medial knee osteoarthritis during 3-d
gait. IEEE Trans Biomed Eng. 2008 Apr; 55(4):1415-
22.
BIOSIGNALS2015-InternationalConferenceonBio-inspiredSystemsandSignalProcessing
370