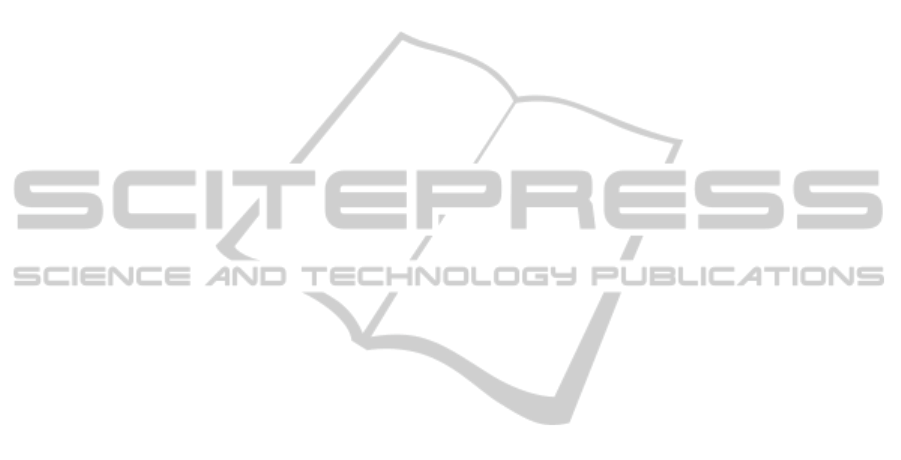
and grasses, waving flags and flashing lights as
foreground objects. Second, in the moving vehicle
detection foreground objects are extracted simply
using the background subtraction method. This
method has suffered from illumination changes,
especially shadows, as well as slowly moving
traffics. Third, the morphological process ignores
isolate road regions. This leads to defective
preliminary road segments. Finally, there is no
strategy for dealing with the problem of over-
estimate due to perspective projection of vehicles
moving along the roadside. The above drawbacks
associated with the previous method have been
compensated for in the current method. We have
applied both the previous and current methods to
all the 13 experimental videos. However, the
previous method only worked on six of them (i.e.,
videos 1, 7, 10, 11, 12, and 13). This is probably
because of the associated drawbacks mentioned
above. Table 4 shows the experimental results, in
which except for video 11, the current method has
outperformed the previous one for the rest videos,
especially videos 1 and 12.
5
CONCLUDING REMARKS
AND FUTURE WORK
In this paper, an automatic road segmentation
method was presented. The proposed method is
dedicated to static traffic scenes. Previous
researches have paid more attention on either
dynamic or restricted static scenes. As a matter of
fact, a large number of traffic applications have to
do with static traffic scenes and various conditions
of environment, weather, illumination, viewpoint,
and road type can make the road segmentation of
static traffic scenes challenging too. A number of
video sequences of traffic scenes under different
conditions have been used in our experiments. The
error rates of road segmentation of all experimental
videos were within 25%. In terms of potential
applications, the performance of the proposed road
segmentation method can be acceptable. We will
keep improving the performance of the current
method and develop potential applications in our
future work
REFERENCES
Ndoye, M., Totten V. F., Krogmeier, J. V., and Bullock,
D. M., 2011. Sensing and signal processing for
vehicle re-identification and travel time estimation.
IEEE Trans. on Intelligent Transportation Systems,
12(1), pp. 119-131.
Perez, J., Milanes, V., and Onieva, E., 2011. Cascade
architecture for lateral control in autonomous
vehicles. IEEE Trans. on Intelligent Transportation
Systems, 12(1), pp. 73-82.
Skog, I., and P. Händel, 2009. In-car positioning and
navigation technologies — A Survey. IEEE Trans.
on Intelligent Transportation Systems, 10(3), pp. 4–
21.
Alvarez, J. M., Lopez, A., and Baldrich, R., 2008.
Illuminant-invariant model-based road
segmentation. Proc. of IEEE Intelligent Vehicles
Symp., Eindhoven University of Technology
Eindhoven, The Netherlands.
Chen, Y. Y., & Chen, S. W., 2010. A restricted bus-lane
monitoring system. Proc. of the 23rd IPPR Conf. on
CVGIP, Kaohsiung, Taiwan.
Chung, Y. C., Wang, J. M., Chang, C. L., and Chen, S.
W., 2004. Road segmentation with fuzzy and
shadowed sets. Proc. of Asian Conf. on Computer
Vision, Korea.
Ha, D. M.,
Lee, J. M., and Kim, Y. D., 2004. Neural-
edge-based vehicle detection and traffic parameter
extraction. Image and Vision Computing, 22(11),
pp.899-907.
Alvarez, J. M. and Lopez, A. M., 2011. Road detection
based on illumination invariance. IEEE Trans. on
Intelligent Transportation Systems, 12(1), pp. 184-
193.
Courbon, J., Mezouar, Y., and Martinet, P., 2009.
Autonomous navigation of vehicles from a visual
memory using a generic camera model. IEEE Trans.
on Intelligent Transportation Systems, 10(3), pp.
392-402.
Obradovic, D., Lenz, H., and Shupfner, M., 2008.
Fusion of sensor data in siemens car navigation
system,” IEEE Trans. on Vehicular Technology,
Vol. 56, No. 1, pp. 43-50, 2008.
Danescu, R. and Nedevschi, S., 1994. Probabilistic lane
tracking in difficult road scenarios using
stereovision. IEEE Trans. on Intelligent
Transportation Systems, 10(2), pp. 272-282.
Santos, M., Linder, M., Schnitman, L., Nunes, U., and
Oliveria, L., 2013. Learning to segment roads for
traffic analysis in urban images. IEEE Intelligent
Vehicles Symposium, pp. 527-532, Gold Coast, QLD.
Tan, C., Hong, T., Chang, T., and Shneier, M., 2006.
Color model-based real-time learning for road
following. Proc. of the IEEE Conf. on Intelligent
Transportation Systems, Toronto, Canada.
Sha, Y., Zhang, G. Y., and Yang, Y., 2007. A road
detection algorithm by boosting using feature
combination. Proc. of IEEE Intelligent Vehicles
Symposium, Istanbul, Turkey.
Wang, J. M., Chung, Y. C., Lin, S. C., Chang, S. L., and
Chen, S. W., 2004. Vision-based traffic
measurement system. Proc. of IEEE Int’l. Conf. on
Pattern Recognition, Cambridge, United Kingdom.
VISAPP2015-InternationalConferenceonComputerVisionTheoryandApplications
476