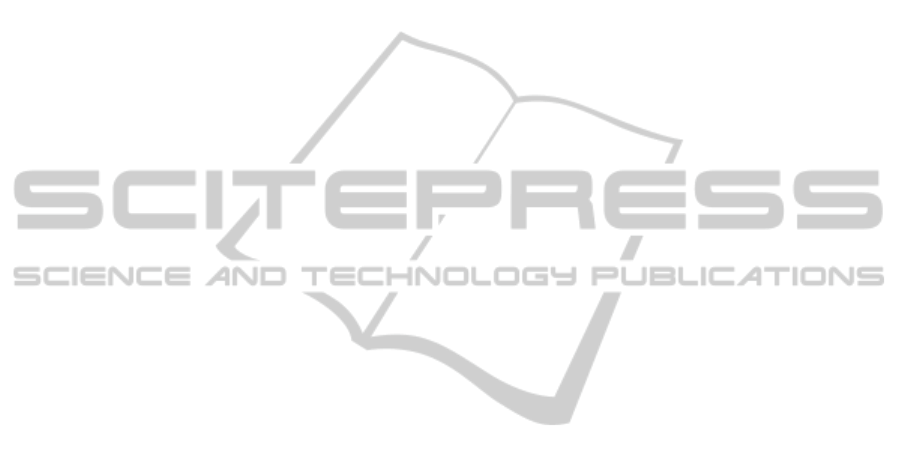
bell, A. (2011). BeWell: A smartphone application to
monitor, model and promote wellbeing. IEEE.
Lara, O. and Labrador, M. (2012). A mobile platform for
real-time human activity recognition. In 2012 IEEE
Consumer Communications and Networking Confer-
ence (CCNC), pages 667–671.
Lara, O. D. and Labrador, M. A. (2013). A survey on human
activity recognition using wearable sensors. Com-
munications Surveys & Tutorials, IEEE, 15(3):1192–
1209.
Lau, S. L., Konig, I., David, K., Parandian, B., Carius-
Dussel, C., and Schultz, M. (2010). Supporting patient
monitoring using activity recognition with a smart-
phone. In Wireless Communication Systems (ISWCS),
2010 7th International Symposium on, pages 810–
814. IEEE.
Liang, Y., Zhou, X., Yu, Z., and Guo, B. (2013). Energy-
efficient motion related activity recognition on mobile
devices for pervasive healthcare. Mobile Networks
and Applications, pages 1–15.
Liang, Y., Zhou, X., Yu, Z., Guo, B., and Yang, Y. (2012).
Energy efficient activity recognition based on low res-
olution accelerometer in smart phones. In Advances
in Grid and Pervasive Computing, number 7296 in
Lecture Notes in Computer Science, pages 122–136.
Springer Berlin Heidelberg.
Lu, H., Yang, J., Liu, Z., Lane, N. D., Choudhury, T., and
Campbell, A. T. (2010). The jigsaw continuous sens-
ing engine for mobile phone applications. In Pro-
ceedings of the 8th ACM Conference on Embedded
Networked Sensor Systems, SenSys ’10, pages 71–84,
New York, NY, USA. ACM.
Marin-Perianu, M., Lombriser, C., Amft, O., Havinga, P.,
and Tr¨oster, G. (2008). Distributed activity recogni-
tion with fuzzy-enabled wireless sensor networks. In
Distributed Computing in Sensor Systems, pages 296–
313. Springer.
Martin, H., Bernardos, A. M., Iglesias, J., and Casar, J. R.
(2013). Activity logging using lightweight classifica-
tion techniques in mobile devices. Personal and Ubiq-
uitous Computing, 17(4):675–695.
Miluzzo, E., Lane, N. D., Fodor, K., Peterson, R., Lu, H.,
Musolesi, M., Eisenman, S. B., Zheng, X., and Camp-
bell, A. T. (2008). Sensing meets mobile social net-
works: The design, implementation and evaluation of
the CenceMe application. In Proceedings of the 6th
ACM Conference on Embedded Network Sensor Sys-
tems, SenSys ’08, pages 337–350, New York, NY,
USA. ACM.
Ouchi, K. and Doi, M. (2012). Indoor-outdoor activ-
ity recognition by a smartphone. In Proceedings of
the 2012 ACM Conference on Ubiquitous Computing,
UbiComp ’12, pages 600–601, New York, NY, USA.
ACM.
Reddy, S., Mun, M., Burke, J., Estrin, D., Hansen, M., and
Srivastava, M. (2010). Using mobile phones to deter-
mine transportation modes. ACM Trans. Sen. Netw.,
6(2):1–27.
Ryder, J., Longstaff, B., Reddy, S., and Estrin, D. (2009).
Ambulation: A tool for monitoring mobility patterns
over time using mobile phones. In International Con-
ference on Computational Science and Engineering,
2009. CSE ’09, volume 4, pages 927–931.
Schindhelm, C. (2012). Activity recognition and step de-
tection with smartphones: Towards terminal based in-
door positioning system. In 2012 IEEE 23rd Inter-
national Symposium on Personal Indoor and Mobile
Radio Communications (PIMRC), pages 2454–2459.
Shoaib, M., Bosch, S., Incel, O. D., Scholten, H., and
Havinga, P. J. M. (2014). Fusion of smartphone mo-
tion sensors for physical activity recognition. Sensors,
14(6):10146–10176.
Shoaib, M., Scholten, H., and Havinga, P. (2013). To-
wards physical activity recognition using smartphone
sensors. In Ubiquitous Intelligence and Computing,
2013 IEEE 10th International Conference on and 10th
International Conference on Autonomic and Trusted
Computing (UIC/ATC), pages 80–87.
Siirtola, P. (2012). Recognizing human activities user-
independently on smartphones based on accelerome-
ter data. International Journal of Interactive Multime-
dia and Artificial Intelligence, 1(Special Issue on Dis-
tributedComputing and Artificial Intelligence):38–45.
Siirtola, P. and Roning, J. (2013). Ready-to-use activity
recognition for smartphones. In 2013 IEEE Sympo-
sium on Computational Intelligence and Data Mining
(CIDM), pages 59–64.
Stewart, V., Ferguson, S., Peng, J.-X., and Rafferty, K.
(2012). Practical automated activity recognition us-
ing standard smartphones. In Pervasive Computing
and Communications Workshops, IEEE International
Conference on, volume 0, pages 229–234, Los Alami-
tos, CA, USA. IEEE Computer Society.
Thiemjarus, S., Henpraserttae, A., and Marukatat, S.
(2013). A study on instance-based learning
with reduced training prototypes for device-context-
independent activity recognition on a mobile phone.
In 2013 IEEE International Conference on Body Sen-
sor Networks (BSN), pages 1–6.
Vo, Q. V., Hoang, M. T., and Choi, D. (2013). Personal-
ization in mobile activity recognition system using k-
medoids clustering algorithm. International Journal
of Distributed Sensor Networks, 2013.
Wang, Y., Lin, J., Annavaram, M., Jacobson, Q. A., Hong,
J., Krishnamachari, B., and Sadeh, N. (2009). A
framework of energy efficient mobile sensing for auto-
matic user state recognition. In Proceedings of the 7th
International Conference on Mobile Systems, Appli-
cations, and Services, MobiSys ’09, pages 179–192,
New York, NY, USA. ACM.
Yan, Z., Subbaraju, V., Chakraborty, D., Misra, A., and
Aberer, K. (2012). Energy-efficient continuous ac-
tivity recognition on mobile phones: An activity-
adaptive approach. In 2012 16th International Sympo-
sium on Wearable Computers (ISWC), pages 17–24.
Zhao, K., Du, J., Li, C., Zhang, C., Liu, H., and Xu, C.
(2013). Healthy: A diary system based on activity
recognition using smartphone. In 2013 IEEE 10th In-
ternational Conference on Mobile Ad-Hoc and Sensor
Systems (MASS), pages 290–294.
DefiningaRoadmapTowardsComparativeResearchinOnlineActivityRecognitiononMobilePhones
159