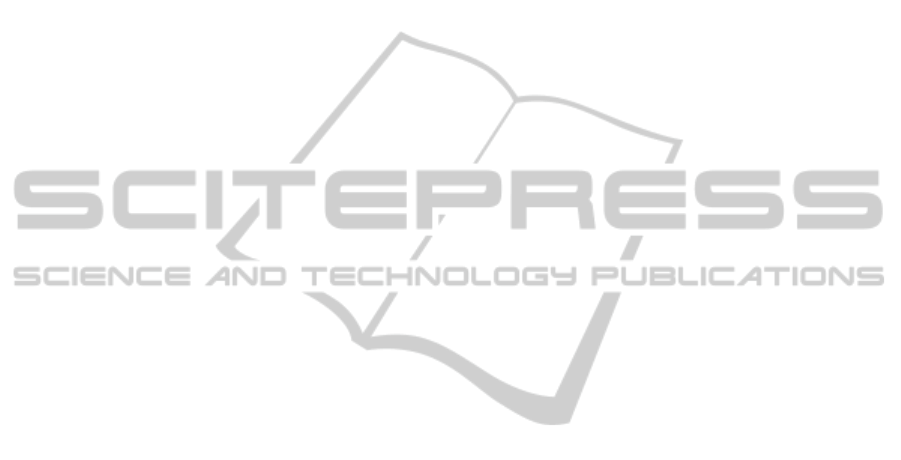
compression on the decomposed coefficients and
then after decompressing those coefficients, features
are extracted from them and used for classification.
REFERENCES
Abo-Zahhad, M. M., Abdel-Hamid, T. K., Mohamed, A.
M., 2014. Compression of ECG signals based on
DWT and exploiting the correlation between ECG
signal samples. Int'l J. of Comm., Network and System
Sciences, 7: 53-70.
Addison, P. S., 2002. The illustrated wavelet transform
handbook: introductory theory and applications in
science, engineering, medicine and finance. CRC.
Ahmed, S.M., Al-Zoubi, Q., Abo-Zahhad, M., 2007. A
hybrid ECG compression algorithm based on singular
value decomposition and discrete wavelet transform.
Journal of Medical Engineering and Technology,
31(1): 54-61.
Alvarado, A.S., Lakshminarayan, C., Principe, J.C., 2012.
Time-based compression and classification of heart-
beats. IEEE Transactions on Biomedical Engineering,
59(6): 1641-1648.
Automated ECG Interpretation, In Wikipedia. Retrieved:
November 4, 2014, from: http://en.wikipedia.org/wiki/
File:SinusRhythmLabels.svg.
Bortolan, G., Willems, J.L., 1993. Diagnostic ECG
classification based on neural networks, Journal of
Electrocardiology, 26: 75-79.
Bulusu, S.C., 2011. Detection of ECG transient ST-
segment episodes and machine learning based heart
beat classification, M.sc Thesis, The University of
Texas at Dallas, May 2010.
Cetin, A.E., Köymen, H., 2000. Compression of Digital
Biomedical Signals. The Biomedical Engineering
Handbook: Second Edition, Ed. J. D. Bronzino, Boca
Raton. CRC Press LLC.
Chan, H.L., Siao, Y.C., Chen, S.W., Yu, S.F., 2008.
Wavelet-based ECG compression by bit-field
preserving and running length encoding. Computer
methods and programs in biomedicine, 90(1): 1-8.
Duda, R.O., Hart, P.E., Stork, D.G., 2001. Pattern
Classification, 2
nd
Edition, John Wiley & Sons, Inc.
Hu, Y.H., Palreddy, S., Tompkins, W.J., 1997. A patient-
adaptable ECG beat classifier using a mixture of
experts approach, IEEE Transactions on Biomedical
Engineering, 44(9): 891-900.
Kamousi, B., 2011. Detection and Classification of
Cardiac Arrhythmias. PhD Thesis, University of
Minnesota, Minneapolis, MN, USA.
Khorrami, H., Moavenian, M., 2010. A comparative study
of DWT, CWT and DCT transformations in ECG
arrhythmias classification. Expert systems with
Applications, 37(8): 5751-5757.
Köhler, B., Hennig, C., Orglmeister, R., 2002. The
principles of QRS detection, IEEE Engineering in
Medicine and Biology, 21(1): 42-57.
Koski, A., Juhola, M., Meriste, M., 1995. Syntactic
recognition of ECG signals by attributed finite
automata, Pattern Recognition, 28(12): 1927-1940.
Manikandan, M.S., Dandapat, S., 2014. Wavelet-based
electrocardiogram signal compression methods and
their performances: A prospective review. Biomedical
Signal Processing and Control, Elsevier, 14: 73-107.
Marcovecchio, A.F., 2001. U.S. Patent No. 6,223,078.
Washington, DC: U.S. Patent and Trademark Office.
Massachusetts Institute of Technology. MIT-BIH ECG
database. Available: http://ecg.mit.edu/.
Miaou, S.G., Chen, S.T., Lin, C.L. 2002. An integration
design of compression and encryption for biomedical
signals. Journal of Medical and Biological
Engineering, 22(4): 183-192.
Pan, J., Tompkins, W.J., 1985, A real-time QRS detection
algorithm, Biomedical Engineering (BME), IEEE
Transactions, 32(3): 230-236.
Peng, C.C., 2011. A Memory-optimized architecture for
ECG signal processing, PhD Thesis, University of
Florida, Florida, United States.
Portet, F., Hernandez, A.I., Carrault, G., 2005. Evaluation
of real-time QRS detection algorithms in variable
contexts, Med. Biol. Eng. Comput., 43(3): 379-385.
Quickselect. In Wikipedia. Retrieved: November 4, 2014,
from: http://en.wikipedia.org/wiki/Quickselect.
Quotb, A., Bornat, Y., Renaud, S., 2011. Wavelet
transform for real-time detection of action potentials in
neural signals. Frontiers in Neuroengineering,
4(Article 7): 1-10.
Rangayyan, R.M., 2006. Biomedical signal analysis: a
case-study approach, Wiley-Interscience, 1
st
edition.
Reid, S., 2010. Model combination in multiclass
classification, PhD Thesis, University of Colorado at
Boulder, Colorado, United States.
Sayadi, O., Shamsollahi, M.B., 2008. ECG denoising and
compression using a modified extended Kalman filter
structure. IEEE Transactions on Biomedical
Engineering, 55(9): 2240-2248.
Senhadji, L., Carrault, G., Bellanger, J.J., Passariello, G.,
1995. Comparing wavelet transforms for recognizing
cardiac patterns, Engineering in Medicine and Biology
Magazine, IEEE, 14(2): 167-173.
Silipo, R., Marchesi, C., 1998. Artificial neural networks
for automatic ECG analysis, IEEE T Transactions on
Signal Processing, 46(5).
Tompkins, W.J., 1995. Biomedical Digital Signal
Processing, Prentice-Hall, Upper Saddle River, NJ.
Trahanias, P., Skordalakis, E., 1990. Syntactic pattern
recognition of the ECG, IEEE Transactions on Pattern
Analysis and Machine Intelligence, 12(7): 648,657.
Tsipouras, M.G., Fotiadis, D.I., Sideris, D., 2005. An
arrhythmia classification system based on the RR-
interval signal, Artificial Intelligence in Medicine,
33(3): 237-250.
Tumer, M.B., Belfore, L.A., Ropella, K.M., 2003. A
syntactic methodology for automatic diagnosis by
analysis of continuous time measurements using
hierarchical signal representations, IEEE Transactions
on Systems, Man, and Cybernetics, Part B:
Cybernetics, ,33(6):951-965.
CardiacArrhythmiasClassificationandCompressionusingaHybridTechnique
23