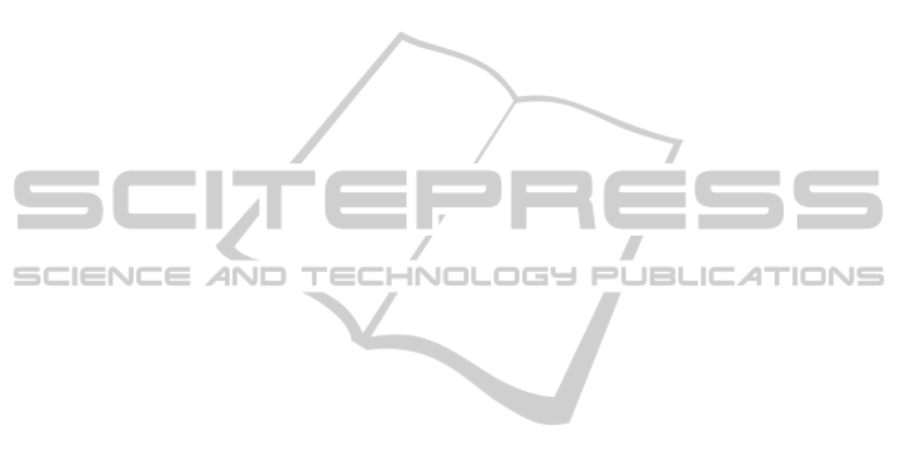
REFERENCES
Ali, S. and Shah, M. (2010). Human action recognition in
videos using kinematic features and multiple instance
learning. Pattern Analysis and Machine Intelligence,
IEEE Transactions on, 32(2):288–303.
Cuturi, M., Vert, J., Birkenes, O., and Matsui, T. (2007).
A kernel for time series based on global alignments.
In Acoustics, Speech and Signal Processing, 2007.
ICASSP 2007. IEEE International Conference on, vol-
ume 2, pages II–413–II–416.
Gong, D. and Medioni, G. (2011). Dynamic manifold warp-
ing for view invariant action recognition. In Computer
Vision (ICCV), 2011 IEEE International Conference
on, pages 571–578.
Gong, D., Medioni, G., and Zhao, X. (2014). Structured
time series analysis for human action segmentation
and recognition. Pattern Analysis and Machine In-
telligence, IEEE Transactions on, 36(7):1414–1427.
Gong, D., Medioni, G., Zhu, S., and Zhao, X. (2012). Ker-
nelized temporal cut for online temporal segmentation
and recognition. In Computer Vision–ECCV 2012,
pages 229–243. Springer.
Junejo, I., Dexter, E., Laptev, I., and Perez, P. (2011).
View-independent action recognition from temporal
self-similarities. Pattern Analysis and Machine Intel-
ligence, IEEE Transactions on, 33(1):172–185.
Lawrence, N. D. (2004). Gaussian process latent variable
models for visualisation of high dimensional data.
Advances in neural information processing systems,
16:329–336.
Lawrence, N. D. and Quinonero-Candela, J. (2006). Local
distance preservation in the gp-lvm through back con-
straints. In Proceedings of the 23rd international con-
ference on Machine learning, pages 513–520. ACM.
Li, R., Chellappa, R., and Zhou, S. K. (2013). Recognizing
interactive group activities using temporal interaction
matrices and their riemannian statistics. International
journal of computer vision, 101(2):305–328.
Li, W., Zhang, Z., and Liu, Z. (2010). Action recognition
based on a bag of 3d points. In Computer Vision and
Pattern Recognition Workshops (CVPRW), 2010 IEEE
Computer Society Conference on, pages 9–14.
Liao, W.-K. and Medioni, G. (2008). 3d face tracking and
expression inference from a 2d sequence using mani-
fold learning. In Computer Vision and Pattern Recog-
nition, 2008. CVPR 2008. IEEE Conference on, pages
1–8.
Lv, F. and Nevatia, R. (2006). Recognition and segmenta-
tion of 3-d human action using hmm and multi-class
adaboost. In Computer Vision–ECCV 2006, pages
359–372. Springer.
Lv, F. and Nevatia, R. (2007). Single view human action
recognition using key pose matching and viterbi path
searching. In Computer Vision and Pattern Recogni-
tion, 2007. CVPR ’07. IEEE Conference on, pages 1–
8.
Mordohai, P. and Medioni, G. (2010). Dimensionality esti-
mation, manifold learning and function approximation
using tensor voting. The Journal of Machine Learning
Research, 11:411–450.
Ning, H., Xu, W., Gong, Y., and Huang, T. (2008). La-
tent pose estimator for continuous action recogni-
tion. In Computer Vision–ECCV 2008, pages 419–
433. Springer.
Noma, H. S. K.-i. (2002). Dynamic time-alignment kernel
in support vector machine. Advances in neural infor-
mation processing systems, 14:921.
Ntouskos, V., Papadakis, P., Pirri, F., et al. (2013). Discrim-
inative sequence back-constrained gp-lvm for mocap
based action recognition. In International Conference
on Pattern Recognition Applications and Methods.
Rakotomamonjy, A., Bach, F., Canu, S., Grandvalet, Y.,
et al. (2008). Simplemkl. Journal of Machine Learn-
ing Research, 9:2491–2521.
Roweis, S. T. and Saul, L. K. (2000). Nonlinear dimension-
ality reduction by locally linear embedding. Science,
290(5500):2323–2326.
Ryoo, M. and Aggarwal, J. (2011). Stochastic represen-
tation and recognition of high-level group activities.
International journal of computer Vision, 93(2):183–
200.
Tenenbaum, J. B., De Silva, V., and Langford, J. C. (2000).
A global geometric framework for nonlinear dimen-
sionality reduction. Science, 290(5500):2319–2323.
Vemulapalli, R., Pillai, J., and Chellappa, R. (2013). Ker-
nel learning for extrinsic classification of manifold
features. In Computer Vision and Pattern Recogni-
tion (CVPR), 2013 IEEE Conference on, pages 1782–
1789.
Wang, J., Fleet, D., and Hertzmann, A. (2008). Gaussian
process dynamical models for human motion. Pat-
tern Analysis and Machine Intelligence, IEEE Trans-
actions on, 30(2):283–298.
Weinland, D.,
¨
Ozuysal, M., and Fua, P. (2010). Making
action recognition robust to occlusions and viewpoint
changes. In Computer Vision–ECCV 2010, pages
635–648. Springer.
Zhang, X. and Fan, G. (2011). Joint gait-pose manifold for
video-based human motion estimation. In Computer
Vision and Pattern Recognition Workshops (CVPRW),
2011 IEEE Computer Society Conference on, pages
47–54.
CollaborativeActivitiesUnderstandingfrom3DData
23