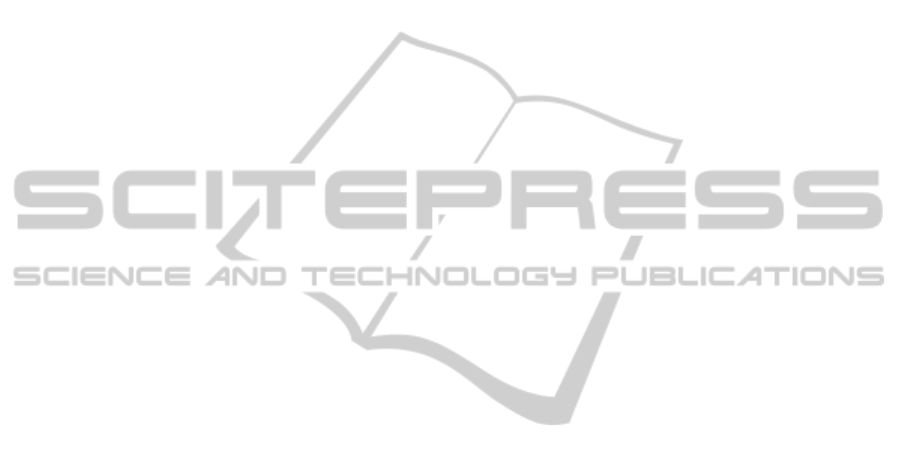
composed only by 24 subjects (15 allergic), the
author is nowadays carrying out a collection process
at allergy section of the Guadalajara University
Hospital (Spain). Subjects of this database are adults
and children exposed to allergy provocation test
involving drugs and food.
Nevertheless, only 2 of 11 subjects that come up
are allergic and not all of them could be detected due
to several circumstances.
Moreover, another application for the use of the
HRV signal is just starting: the detection of
hypoglycaemia in diabetic patients. At present, if a
diabetic patient believes he is in a state of
hypoglycaemia, he needs to do himself a blood
analysis or carry continuously a device that
measures the glucose level on the interstitial tissue.
This devices are carried inserted, and apart from
they affect the comfort of the patients, they are very
expensive. Besides, this devices have a big delay on
the level of glucose measurement on situations of
abrupt changes: immediately after a big meal or
during the realisation of physical activity.
It has been studied that, during a hypoglycaemia,
the body generates adrenaline (like during a stress
situation), which provokes a HR elevation (O.
Hamdy et al., 2014). In this case, what makes
difficult the detection of this situations are the daily
activities that also elevate the HR, like the
realization of physical activity. In this way, it is
planned to study the HR and the movement (by
using inertial sensors) of a series of diabetic subjects
to establish the differences between normal HR
elevations and those produced during normal
situations.
ACKNOWLEDGEMENTS
This work is supported in part by the Spanish
Ministry of Economy and Competitiveness (LORIS
Project, TIN2012-38080-C04-01) and by the
University of Alcalá trough the FPI program.
REFERENCES
G. M. Friesen, T. C. Jannett, M. a Jadallah, S. L. Yates, S.
R. Quint, and H. T. Nagle, “A comparison of the noise
sensitivity of nine QRS detection algorithms.,” IEEE
Trans. Biomed. Eng., vol. 37, no. 1, pp. 85–98, Jan.
1990.
M. W. Phyu, Y. Zheng, B. Zhao, L. Xin, and Y. S. Wang,
“A real-time ECG QRS detection ASIC based on
wavelet multiscale analysis,” in 2009 IEEE Asian
Solid-State Circuits Conference, 2009, pp. 293–296.
S.-W. Chen, H.-C. Chen, and H.-L. Chan, “A real-time
QRS detection method based on moving-averaging
incorporating with wavelet denoising.,” Comput.
Methods Programs Biomed., vol. 82, no. 3, pp. 187–
95, Jun. 2006.
C. I. Ieong, M. I. Vai, and P. U. Mak, “ECG QRS
Complex detection with programmable hardware.,”
Conf. Proc. IEEE Eng. Med. Biol. Soc., vol. 2008, pp.
2920–3, Jan. 2008.
A. Ghaffari, H. Golbayani, and M. Ghasemi, “A new
mathematical based QRS detector using continuous
wavelet transform,” Comput. Electr. Eng., vol. 34, no.
2, pp. 81–91, Mar. 2008.
C. Xiaomeng, “A NEW real-time ECG R-wave detection
algorithm,” in Proceedings of 2011 6th International
Forum on Strategic Technology, 2011, vol. 1, no. 2,
pp. 1252–1255.
R. Rodriguez, A. Mexicano, J. Bila, R. Ponce, S.
Cervantes, and A. Martinez, “Hilbert transform and
neural networks for identification and modeling of
ECG complex,” in Third International Conference on
Innovative Computing Technology (INTECH 2013),
2013, pp. 327–332.
S. Pal and M. Mitra, “Empirical mode decomposition
based ECG enhancement and QRS detection.,”
Comput. Biol. Med., vol. 42, no. 1, pp. 83–92, Jan.
2012.
S. Pal and M. Mitra, “QRS Complex detection using
Empirical Mode Decomposition based windowing
technique,” in 2010 International Conference on
Signal Processing and Communications (SPCOM),
2010, pp. 1–5.
M. K. Das, S. Ari, and S. Priyadharsini, “On an algorithm
for detection of QRS complexes in noisy
electrocardiogram signal,” in 2011 Annual IEEE India
Conference, 2011, no. ii, pp. 1–5.
B. Khiari, E. Ben Braiek, and M. Jemni, “R-wave
detection using EMD and bionic wavelet transform,”
in 2013 International Conference on Electrical
Engineering and Software Applications, 2013, pp. 1–
5.
S. Kouchaki, A. Dehghani, S. Omranian, and R. Boostani,
“ECG-based personal identification using empirical
mode decomposition and Hilbert transform,” in The
16th CSI International Symposium on Artificial
Intelligence and Signal Processing (AISP 2012), 2012,
no. Aisp, pp. 569–573.
M. Zhang and C. Zhang, “A novel ECG signal denoising
method based on Hilbert-Huang Transform,” in 2010
International Conference on Computer and
Communication Technologies in Agriculture
Engineering, 2010, pp. 284–287.
J. Moraes, M. M. Freitas, F. N. Vilani, and E. V. Costa,
“A QRS complex detection algorithm using
electrocardiogram leads,” in Computers in Cardiology,
2002, pp. 205–208.
C. Choi, Y. Kim, and K. Shin, “A PD control-based QRS
detection algorithm for wearable ECG applications.,”
BIOSTEC2015-DoctoralConsortium
34