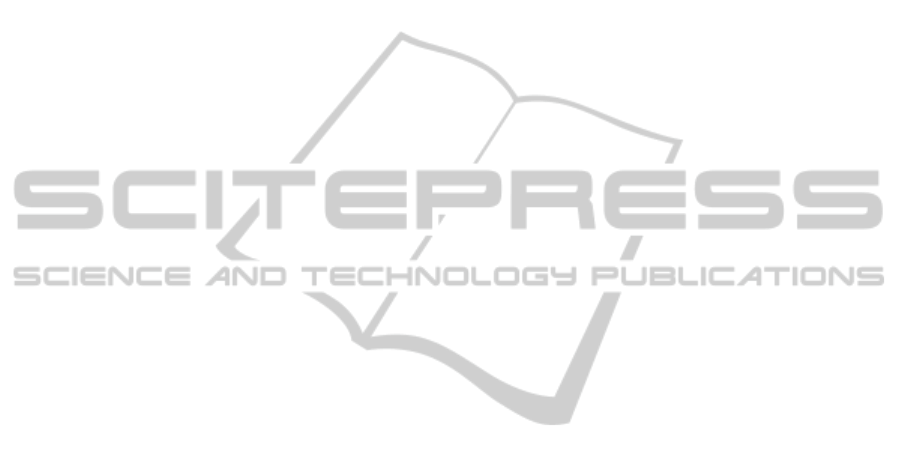
6 CONCLUSION
The proposed plan enable us to detect most HVS
episodes at its onset from the local field potentials
recorded from awake, freely moving rats and to trig-
ger the delivery of stimulations with arbitrary wave-
forms onto particular brain regions upon detecting
HVSs automatically. More generally, it could help to
improve the existing biometrics methods through bet-
ter event detection and apparently it is well adapted
for multidimensional signal. Previous results show
that the proposed filter detects about 90% of the
events and commits few errors, ensuring that most
HVS episodes identified are in agreement with real-
ity.
This corpus of methods relies on a running time
window whose window length (0.5−1s) is too long
to efficiently stop individual HVSs episodes (1−4 s).
Under this concern we will investigate in the future
the feasibility of using dynamical models to predict
the occurrence of a HVS before its onset and study if
β-waves can be induced by artificially evoked HVSs
REFERENCES
Clancy, E. and Farry, K. (2000). Adaptive whitening of
the electromyogram to improve amplitude estimation.
IEEE Trans Biomed Eng., 47(6).
Cover, T. and Thomas, J. (1991). Elements of Information
Theory. John Wiley and Sons.
DeFreitas, J., Beck, T., and Stock, M. (2012). Compar-
ison of methods for removing electromagnetic noise
from electromyographic signals. Physiological Mea-
surement, 33(2):147.
Denzler, J. and Brown, C. (2002). Information theoretic
sensor data selection for active object recognition and
state estimation. IEEE trans. on Pat. Anal. and Mach.
Intel., 24(2):145–156.
Johnson, B. A., Abramovich, Y. I., and Scharf, L. L. (2011).
Detectionestimation of multi-rank gaussian sources
using expected likelihood. Digital Signal Processing,
21(5):568 – 575.
Miyano, H., Masuda, T., and Sadoyama, T. (1980). A note
on the time constant in low-pass filtering of rectified
surface emg. IEEE Trans Biomed Eng., 27(5):274–8.
Perumal, R. and Chen, H. (2014). Performance analysis in
a wavelet-based algorithm for automatic detection of
high-voltage spindles in parkinson’s disease rat mod-
els. In 9th Asian-Pacific Conference on Medical and
Biological Engineering, Tainan, Taiwan.
Radek, R. J., Curzon, P., and Decker, M. W. (1994). Charac-
terization of high voltage spindles and spatial memory
in young, mature and aged rats. Brain Research Bul-
letin, 33:183188.
Sitnikova, E., Hramov, A. E., Koronovsky, A. A., and van
Luijtelaar, G. (2009). Sleep spindles and spike-wave
discharges in eeg: their generic features, similarities
and distinctions disclosed with fourier transform and
continuous wavelet analysis. J. Neurosci. Methods,
180:304316.
Vigneron, V. and Jutten, C. (2004). Independent Component
Analysis and Blind Signal Separation, volume LNCS
3195 of Lectures Notes in Computer Science, chap-
ter Fisher Information in Source Separation Problems,
pages 168–176. Springer.
Vigneron, V., Syed, T., Montagne, C., Barlovatz-Me imon,
G., and Lelandais, S. (2010). Template matching and
test detection. Application to cell l ocalization in cells
imagery. Pattern Recognition Letters, 31(14):2214–
2224.
Vrins, F., Jutten, C., and Verleysen, M. (2004). Fifth Inter-
national Conference, ICA 2004, volume LNCS 3195,
proceedings Sensor array and electrode selection for
non invasive fetal electrocardiogram extraction by In-
dependent Component Ananlysis. Springer-Verlag,
Granada, Spain.
Yao, K., Hudson, R., D., C., and Lorenzelli, F. (1998). Blind
beamforming on a randomly distributed sensor array
system. IEEE Journal on selected areas in communi-
cation, 16(8):1555–1566.
BIOSIGNALS2015-InternationalConferenceonBio-inspiredSystemsandSignalProcessing
378