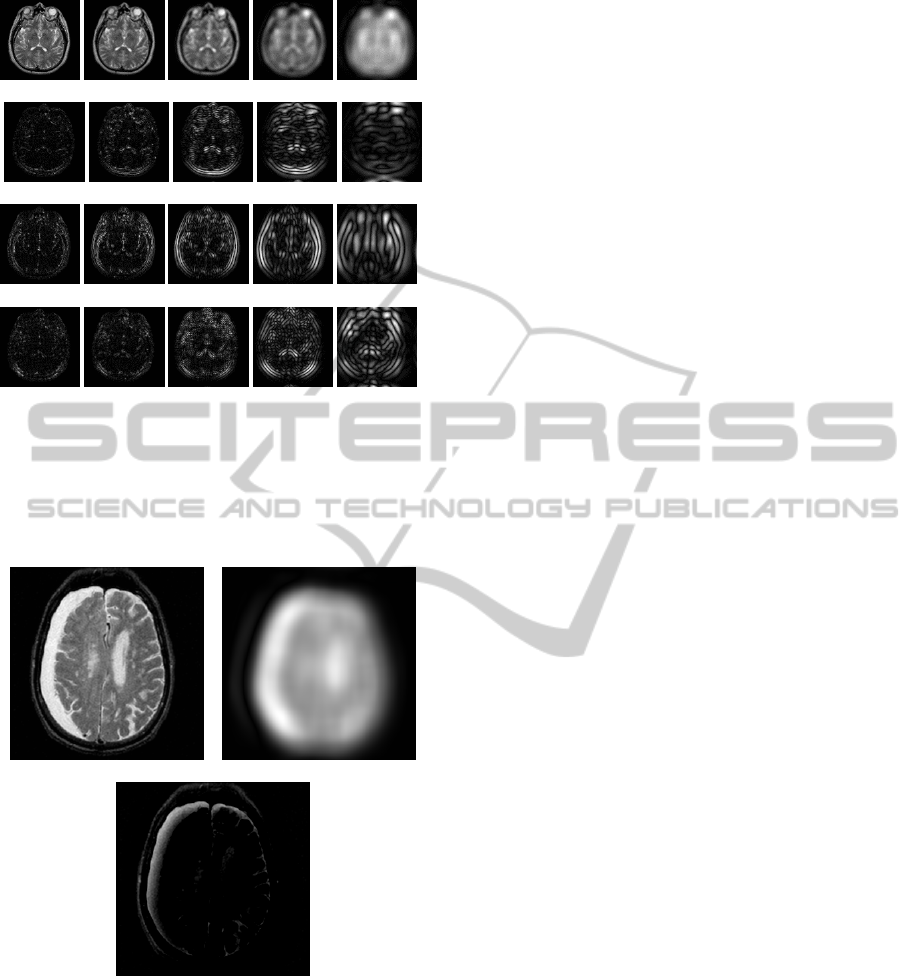
Figure 3: UWT outputs (5 levels using the Daubechies 2
(db2) wavelet): application to Figure 2(f). From left to
right, the five levels (15). From top to down, the LL,
LH, HL, and HH components, respectively. This work
assumes that it is possible to discover more pertinent sub-
bands (to address different classification scenarios) by
combining the base UWT sub-bands.
(a) (b)
(c)
Figure 4: an example of a pertinent handcrafted
combination using the db2 wavelet: (a) the approximation
coefficients of the first UWT decomposition level of
Figure 2(b), (b) the approximation coefficients of the fifth
UWT decomposition level of Figure 2(b), and (c) result of
(a) minus (b). From (c), one can note that a large part of
the hematoma is highlighted (next to some other small
non-pertinent regions).
new useful sub-bands (from the base UWT sub-
bands) using GP. The newly created representations
can be used as they are, or they can serve as a base
for an efficient feature extraction or reduction phase.
We are currently testing the proposed method
according to the different scenarios defined earlier.
Note that the fallout out of the proposed scheme
goes far beyond the problem treated in this paper,
since it could allow the creation of sub-bands that
can efficiently highlight a given structure (thalamus,
caudate nucleus, etc.) or a given cerebral matter
(White Matter, Gray Matter, or Cerebrospinal Fluid).
Thereby, some important tasks like segmentation or
quantification could be greatly enhanced.
The current state the of the system enables to
exploit the sub-bands that are produced by only one
wavelet at a time. However, one can envisage to use
the outputs of different wavelets simultaneously.
REFERENCES
Breiman, L., 2001. Random forests, Machine Learning.
45(1), 5-32.
Chaplot, S., Patnaik, L. M., Jagannathan, N. R., 2006.
Classification of magnetic resonance brain images
using wavelets as input to support vector machine and
neural network. Biomedical Signal Processing and
Control 1(1), 86-92 (2006).
Darwin, C., 1864. On the origin of species by means of
natural selection or the preservation of favoured races
in the struggle for life. Cambridge University Press.
Cambridge, UK.
El-Dahshan, E. S. A., Hosny. T., Salem, A. B. M., 2010.
Hybrid intelligent techniques for MRI brain images
classification. Digital Signal Processing 20(2), 433-
441.
Kalbkhani, H., Shayesteh, M. G., Zali-Vargahan, B., 2013.
Robust algorithm for brain magnetic resonance image
(MRI) classification based on GARCH variances
series. Biomedical Signal Processing and Control 8(6),
909-919.
Koza, J., 1992. Genetic programming: On the
programming of computers by means of natural
selection. MIT Press. Cambridge, MA, USA.
Lahmiri, S., Boukadoum, M., 2011. Classification of brain
MRI using the LH and HL wavelet transform sub-
bands. In IEEE International Symposium on Circuits
and Systems 1025-1028 (Rio de Janeiro).
Langdon, W. B., Poli, R., MacPhee, N. F., Koza, J. R.,
2008. Genetic Programming: An Introduction and
Tutorial, with a Survey of Techniques and
Applications, in Computational Intelligence: A
Compendium, Studies in Computational Intelligence
115, 927-1028, Springer Heidelberg. Berlin,
Deutschland.
Mallat, S. G., 1989. A theory for multiresolution signal
decomposition: the wavelet representation. In IEEE
Transactions on Pattern Analysis and Machine
Intelligence 11(7), 674-693.
AutomaticGenerationofSuitableDWTSub-band-AnApplicationtoBrainMRIClassification
169