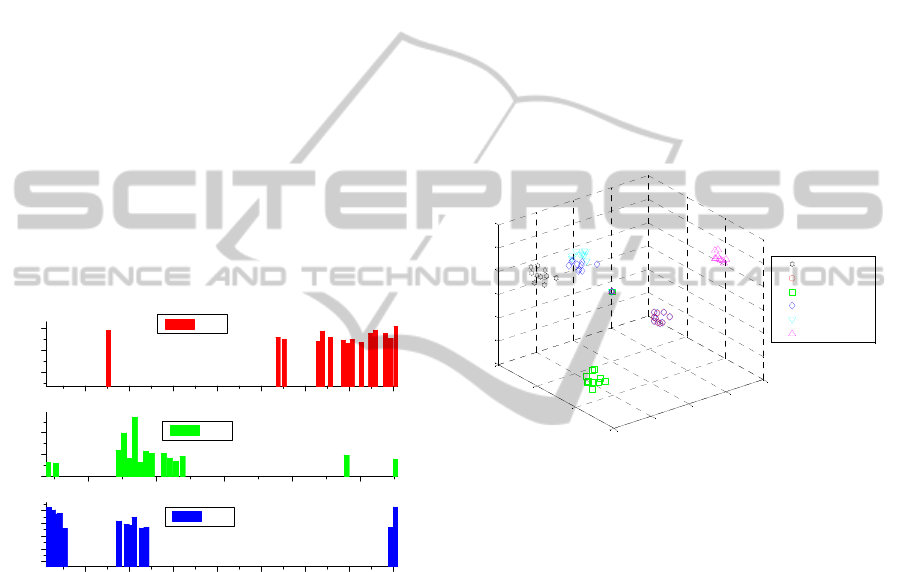
number of significant components (Giubileo et al.
2012).
After performing the PCA resulted that the first
three principal components are explaining 78.3% of
the overall spectral variation. The corresponding
loading plots, which indicate the specific
contribution of each absorption line in the total
variance of the spectral data, are reported in the
Figure 7. The first principal component, PC1, which
accounts for the 42.8 % of spectral variance, has one
isolated peak around 9.5 µm that correspond to some
vibrations in the two nitro-compounds (NH
4
NO
3
and KNO
3
). Generally, the fingerprints of inorganic
compounds fall in the region 25 µm – 1 mm (400 –
10 cm
-1
) due to the presence of the lattice modes of
vibration which are characteristic of a specific
crystal geometry (Nyquist et al. 1997). These modes
result from the motion of one polyatomic group
relative to another within the unit cell. Thus, there
are no absorption peaks in the interval 9-11 µm
which can be ascribed to a specific mode of
vibration of some functional groups inside the
sample.
Figure 7: Loadings plots for the first three components.
In the second component, PC2, with an explained
spectral variance of 19.8 %, there is a wider band
centered around one isolated peak around 9.5 µm
that correspond to some vibrations in the NH
4
NO
3
,
NH
4
ClO
4
and KNO
3
.
The loading plot for PC3, which explains the
15.7 % of spectral variance, shows, besides the
peaks in position equivalent to those in the PC1 or
PC2, a strong band in the 9P branch of the CO
2
laser
emission wavelengths.
In general, a low number of PC able to explain
more than 60% of the spectral variances is correlated
with a large spectral difference among the samples.
Thus, in our case only two components can be
sufficient to describe the data set, as evidenced in
Figure 6, which shows the PCA plot for the PC1 and
PC2, the two largest principal components of the
dataset, which explain 62.6 % of the spectral
variance between the samples. In this plot each
sample is represented by a point, and the six groups,
each corresponding to a precursor substance, are
clearly separated.
In Figure 8 we report the 3D plot of PC1, PC2
and PC3 (78.3 % explained variance), which clearly
shows that each compound can be correctly grouped
with no misassignment. Therefore, the application of
PCA to the LPAS spectra expressed by 55 different
wavelengths allowed to reduce the output to only
three components. The score plots indicate that the
proposed model is able to correctly group the IED’s
precursors in spite of the oversimplification of LPAS
spectra.
Figure 8: PCA results in the 3D space generated by the
first three components (PC1, PC2, and PC3).
5 CONCLUSIONS
The concept of LPAS recognition of IED precursors
has been demonstrated by performing measurements
on the selected set of chemicals reported in Table 2.
PA spectroscopy put in evidence different spectral
patterns characteristic for each substance in the
spectral interval covered by the CO
2
laser.
Nevertheless, in order to simplify the daunting task
of substance identification, a multivariate statistical
analysis tool based on PCA was developed in
MatLab. In spite of the fact that inorganic
compounds identification by infrared spectroscopy is
considered somewhat less successful in the middle
infrared (MIR) region, the recognition ability of
MIR-LPAS technique coupled with a PCA data
treatment was demonstrated by the reported results.
Applying PCA to the LPAS spectra, it was found
that 78.3 % of the spectral variation was accounted
for by the first three principal components. This
9,4 9,6 9,8 10,0 10,2 10,4 10,6 10,8
-0,2
-0,1
0,0
0,1
0,2
9,4 9,6 9,8 10,0 10,2
0,0
0,1
0,2
9,4 9,6 9,8 10,0 10,2 10,4 10,6 10,8
-0,2
-0,1
0,0
loadings
Wavenumber [cm
-1
]
PC3
PC2
PC1
-2
-1
0
1
2
-2
-1
0
1
-1.5
-1
-0.5
0
0.5
1
1.5
PC1
PC2
K2SO4 - (1)
KNO3 - (2)
MgSO4 - (3)
NH4NO3 - (4)
ClO4NO4 - (5)
acetone - (6)
SpectroscopicStudyofSomeIED'sPrecursorsbyMeansofLaserPhotoacousticSpectroscopyCombinedwithMultivariate
Analysis
29