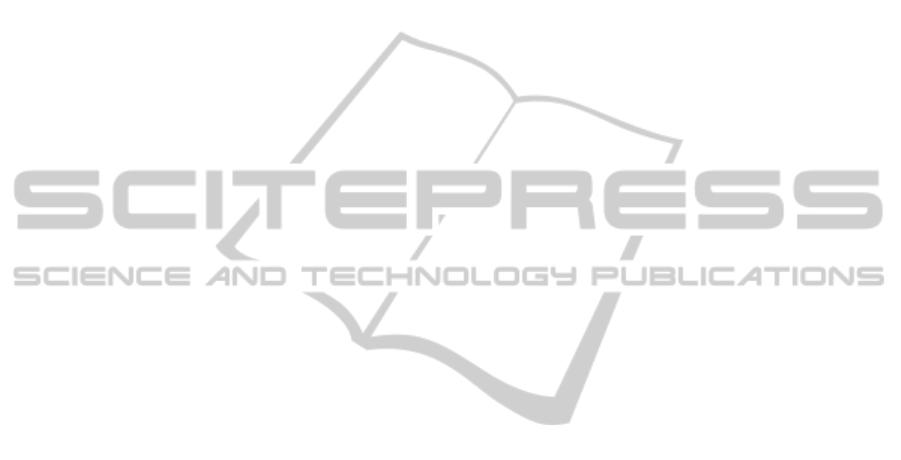
and online three-day ahead wind power forecasting.
Power Systems, IEEE Transactions on, 24(4):1657–
1666.
Bifet, A., Holmes, G., Kirkby, R., and Pfahringer, B.
(2010a). Moa: Massive online analysis. The Journal
of Machine Learning Research, 11:1601–1604.
Bifet, A., Holmes, G., and Pfahringer, B. (2010b). Leverag-
ing bagging for evolving data streams. In Machine
Learning and Knowledge Discovery in Databases,
pages 135–150. ECML PKDD.
Bifet, A., Holmes, G., Pfahringer, B., Kirkby, R., and
Gavaldà, R. (2009). New ensemble methods for evolv-
ing data streams. In Proc. of the 15th ACM SIGKDD
international conference on Knowledge discovery and
data mining, pages 139–148. ACM SIGKDD.
Brzezinski, D. and Stefanowski, J. (2013). Reacting to dif-
ferent types of concept drift: The accuracy updated
ensemble algorithm. IEEE Transactions on Neural
Networks and Learning Systems, 25(1):81–94.
Domingos, P. and Hulten, G. (2000). Mining high-speed
data streams. In Proc. of the sixth ACM SIGKDD in-
ternational conference on Knowledge discovery and
data mining, pages 71–80. ACM SIGKDD.
Ferreira, J., J. P. M. J. (2012). Gis for crime analysis: Ge-
ography for predictive models. 15:36–49.
Gama, J. and Rodrigues, P. (2009). Issues in evaluation
of stream learning algorithms. In Proc. of the 15th
ACM SIGKDD international conference on Knowl-
edge discovery and data mining, pages 329–338.
ACM SIGKDD.
Gama, J. a., Žliobait
˙
e, I., Bifet, A., Pechenizkiy, M., and
Bouchachia, A. (2014). A survey on concept drift
adaptation. ACM Comput. Surv., 46(4):44:1–44:37.
Gomes, H. M. and Enembreck, F. (2013). Sae: Social adap-
tive ensemble classifier for data streams. In Compu-
tational Intelligence and Data Mining (CIDM), 2013
IEEE Symposium on, pages 199–206.
Gomes, H. M. and Enembreck, F. (2014). Sae2: Ad-
vances on the social adaptive ensemble classifier for
data streams. In Proceedings of the 29th Annual ACM
Symposium on Applied Computing (SAC), SAC 2014.
ACM.
John, G. H. and Langley, P. (1995). Estimating continuous
distributions in bayesian classifiers. In Eleventh Con-
ference on Uncertainty in Artificial Intelligence, pages
338–345, San Mateo. Morgan Kaufmann.
Kolter, J. Z. and Maloof, M. A. (2005). Using additive ex-
pert ensembles to cope with concept drift. In ICML
’05 Proc. of the 22nd international conference on Ma-
chine learning, pages 449–456. ACM.
Kolter, J. Z. and Maloof, M. A. (2007). Dynamic weighted
majority: An ensemble method for drifting concepts.
In The Journal of Machine Learning Research, pages
123–130. JMLR.
Kuncheva, L. I. (2004). Combining Pattern Classifiers:
Methods and Algorithms. John Wiley and Sons, New
Jersey.
Kuncheva, L. I., Whitaker, C. J., Shipp, C. A., and Duin,
R. P. (2003). Limits on the majority vote accuracy
in classifier fusion. Pattern Analysis & Applications,
6(1):22–31.
Machado, D. M. S. (2009). O uso da informação na gestão
inteligente da segurança pública. In A força policial:
órgão de informação e doutrina da instituição policial
militar, pages 77–85.
Nath, S. V. (2006). Crime pattern detection using data min-
ing. In Web Intelligence and Intelligent Agent Tech-
nology Workshops, 2006. WI-IAT 2006 Workshops.
2006 IEEE/WIC/ACM International Conference on,
pages 41–44.
Oza, N. C. and Russell, S. (2001). Online bagging and
boosting. In Artificial Intelligence and Statistics,
pages 105–112. Society for Artificial Intelligence and
Statistics.
Pechenizkiy, M., Bakker, J., Žliobait
˙
e, I., Ivannikov, A., and
Kärkkäinen, T. (2010). Online mass flow prediction in
cfb boilers with explicit detection of sudden concept
drift. SIGKDD Explor. Newsl., 11(2):109–116.
Poelmans, J., Elzinga, P., Viaene, S., and Dedene, G.
(2011). Formally analysing the concepts of do-
mestic violence. Expert Systems with Applications,
38(4):3116 – 3130.
Schlimmer, J. C. and Granger, R. H. (1986). Incremen-
tal learning from noisy data. Machine Learning,
1(3):317–354.
Siklóssy, L. and Ayel, M. (1997). Datum discovery. In Ad-
vances in Intelligent Data Analysis, Reasoning about
Data, Second International Symposium, IDA-97, Lon-
don, UK, August 4-6, 1997, Proceedings, pages 459–
463.
Tsymbal, A. (2004). The problem of concept drift: defi-
nitions and related work. Technical Report TCD-CS-
2004-15, The University of Dublin, Trinity College,
Department of Computer Science, Dublin, Ireland.
Wang, T., Rudin, C., Wagner, D., and Sevieri, R. (2013).
Learning to detect patterns of crime. In Machine
Learning and Knowledge Discovery in Databases,
volume 8190 of Lecture Notes in Computer Science,
pages 515–530. Springer Berlin Heidelberg.
Widmer, G. and Kubate, M. (1996). Learning in the pres-
ence of concept drift and hidden contexts. Machine
Learning, 23(1):69–101.
Zliobaite, I. (2010). Learning under concept drift: an
overview. CoRR, abs/1010.4784.
ICEIS2015-17thInternationalConferenceonEnterpriseInformationSystems
24