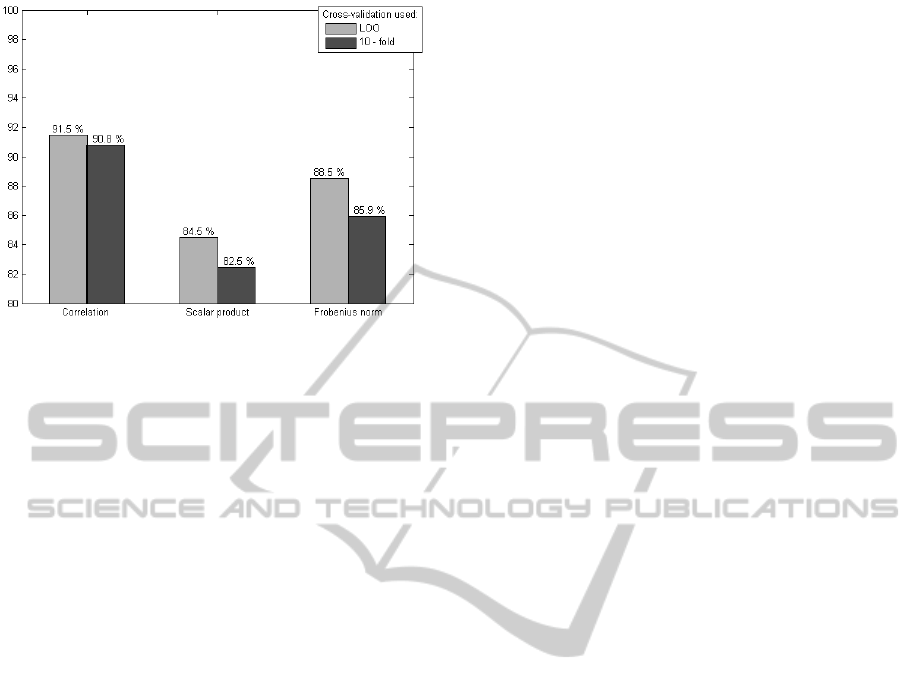
Figure 4: Obtained classification rates (%) for the three
different distances when LDA classification is used for the
class association. Exact experimental values are presented
at the top of each bar.
Figure 3 summarizes the obtained classification
results only based on the criterion of the minimum
distance. 90.0% of accuracy is obtained with
correlation, 83.0% is obtained when the used
measure is the scalar product, and 86.5% is obtained
with the Frobenius norm.
Figure 4 summarizes the obtained classification
results using LDA in the classification step. Results
obtained using LOOCV and 10-fold cross-validation
are presented. Interestingly, the use of LDA and
LOO cross-validation presents some small
improvement in the results. Classification rates (CR)
obtained using LOO cross-validation are 91.5%,
84.5% and 88.5% for correlation, scalar product and
Frobenius norm respectively. These results are
higher than results presented in Figure 3. However,
when the 10-fold cross-validation is applied, only
Correlation (which already presented the best result)
presents better CR than those obtained when the
class association is done only with the criterion of
the minimum distance. Results obtained for scalar
product and Frobenius norm present a decrease in
comparison with results showed in Figure 3.
These experiments show that results obtained
without any classifier are improved when LDA with
LOO cross-validation is used. However, the
variation is very small in these first experiments and
we will try to increase it in future research.
4 CONCLUSION AND
DISCUSSION
This study explores the use of FSBEMD for face
recognition. Distance measures are computed
between BIMFs obtained from reference images and
the image to classify. Then, class is assigned based
on the minimum distance between references and the
images of example, and also using a LDA classifier.
Obtained results do not overcome existing results
in the literature. Results presented in (Travieso et al.
2008) and in (Gallego-Jutglà et al. 2013) achieve a
98% of performance in the classification, which is
better than results presented in this work. However,
in the present work a more simple approach is used.
Results obtained in (Travieso et al. 2008) use a
DCT or DWT (Biorthonal 4.4 family)
parameterization, which is combined with a support
vector machine classifier. Here, the new FSBEMD
technique is used to decompose each image of the
data set and the vector of distance measures is used
alone or is given to a classifier (LDA or ANN). In
this case, the proposed system does not use any kind
of transformation (DCT, DWT or others).
On the other hand, results presented in (Gallego-
Jutglà et al. 2013) also present a CR of 98.25%. In
this work no transformation such as DCT, DWT or
others was applied. Methodology used in (Gallego-
Jutglà et al. 2013) was based on mEMD to compute
the joint decomposition of two images. Best result
obtained was found by classifying obtained
distances with an ANN. 98.25% of CR was achieved
when all measures were combined as input features
for the ANN, which may lead to overfitting.
However, when each measure was used alone, the
best result obtained was 97.25%. Another
shortcoming of the work presented in (Gallego-
Jutglà et al. 2013) is that if an implementation would
be done in a real system the mEMD of the image to
classify and all the existing images of the data base
would have to be computed. This procedure is time
consuming and therefore if the data base is big could
not be implemented in real time. Methods defined in
this work, however, compute the FSBEMD of an
image which is time consuming, but this
decomposition is only computed once.
Results presented here explore the two
dimensional information contained in the image.
Even though more information is used, results do not
overcome previous results that used only one
dimension. One possible shortcoming of the
presented methodology is that some information
may be lost in the average done to obtain the
distance value D
s
. Moreover, all extracted FSIMF
are used whereas some of them may not add any
new information but noise. This will be further
analysed in future work.
On the other hand, in this study the original size
BIOSIGNALS2015-InternationalConferenceonBio-inspiredSystemsandSignalProcessing
390