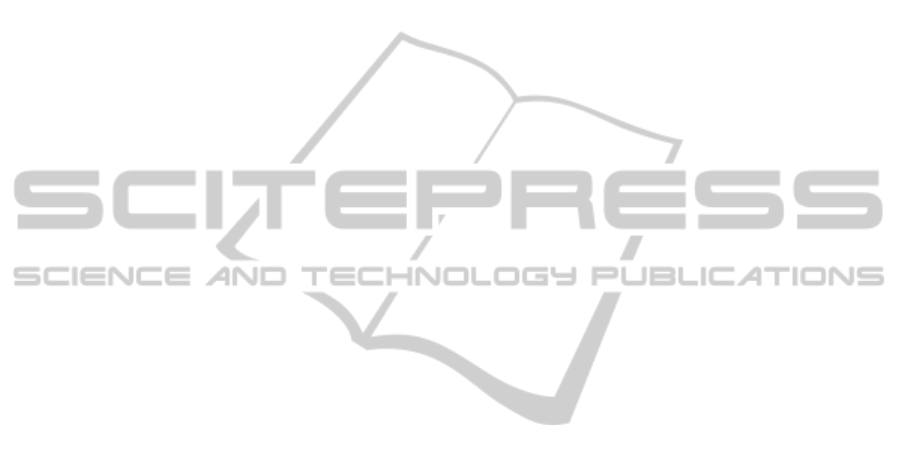
• Identification of features and sublanguage struc-
tures that are associated to clinical concepts, such
as homelessness and psycho-social factors.
• Discovery of phrases and terms associated to the
clinical domain.
• Minimizing supervised learning in lexicon build-
ing.
• Provide a standardized ontology based semantic
lexicon for interoperability and improved NLP
performance.
• Allow re-use of the ontology based semantic lexi-
con in more than one natural language processing
system.
• Enabling maintainability, where new items could
be added to the semantic lexicon in a consistent
manner.
In addition to promote semantic interoperability
for NLP applications, this research study has also re-
vealed practical implications for the VA. A sugges-
tion for future study would be to determine accu-
rate estimates of patients from secondary use of EHR
data. For instance, a practical implication would be to
study the extent of homeless Veterans among different
VISNS and refined estimates of homelessness could
be established. Above all, the proposed methodology
could be an opportunity to standardize the terminol-
ogy related to homelessness in different VA medical
facilities around the country.
REFERENCES
Abacha, A. and Zweigenbaum, P. (2011). Medical entity
recognition: A comparison of semantic and statisti-
cal methods. Proceedings of BioNLP 2011 Workshop,
pages 56–64.
Batet, M., S´anchez, D., and Valls, A. (2011). An ontology-
based measure to compute semantic similarity in
biomedicine. J Biomed Inform, 44(1):118–25.
Birman-Deych, E., Waterman, A. D., Yan, Y., Nilasena,
D. S., Radford, M. J., and Gage, B. F. (2005). Accu-
racy of ICD-9-CM codes for identifying cardiovascu-
lar and stroke risk factors. Medical care, 43(5):480–5.
Buitelaar, P., Cimiano, P., Haase, P., and Sintek, M.
(2009). Towards linguistically grounded ontologies.
The Semantic Web: Research and Applications Lec-
ture Notes in Computer Science, 5554:111–125.
Chou, Y.-M. and Huang, C.-R. (2010). Hantology: concep-
tual system discovery based on orthographic conven-
tion. CAMBRIDGE University Press.
Cimiano, P. (2005). Text2onto–a framework for ontology
learning and data-driven change discovery. NLDB’05
Proc 10th Int Conf Nat Lang Process Inf Syst.
Coden, A. R., Pakhomov, S. V., Ando, R. K., Duffy, P. H.,
and Chute, C. G. (2005). Domain-specific language
models and lexicons for tagging. J Biomed Inform,
38(6):422–30.
Cohen, A. M., Hersh, W. R., Dubay, C., and Spackman, K.
(2005). Using co-occurrence network structure to ex-
tract synonymous gene and protein names from med-
line abstracts. BMC Bioinformatics, 6:103.
Friedlin, J. and Overhage, M. (2011). An evaluation of the
umls in representing corpus derived clinical concepts.
AMIA Annu Symp Proc, 2011:435–44.
Friedman, C., Kra, P., and Rzhetsky, A. (2002). Two
biomedical sublanguages: a description based on the
theories of zellig harris. Journal of Biomedical Infor-
matics, 35(4):222–235.
Friedman, C., Rindflesch, T. C., and Corn, M. (2013). Natu-
ral language processing: state of the art and prospects
for significant progress, a workshop sponsored by the
National Library of Medicine. Journal of biomedical
informatics, 46(5):765–73.
Grishman, R. (2001). Adaptive information extraction and
sublanguage analysis. Proc. of IJCAI 2001, pages 1–4.
Guthrie, L., Pustejovsky, J., Wilks, Y., and Slator, B. M.
(1996). The role of lexicons in natural language pro-
cessing. Commun. ACM, 39(1):63–72.
Howes, C., Purver, M., and McCabe, R. (2013). Investi-
gating topic modelling for therapy dialogue analysis.
WCS 2013 Workshop on Computational Semantics in
Clinical Text.
Huang, J., Dou, D., Dang, J., Pardue, J. H., Qin, X., Huan,
J., Gerthoffer, W. T., and Tan, M. (2012). Knowledge
acquisition, semantic text mining, and security risks
in health and biomedical informatics. World J Biol
Chem, 3(2):27–33.
Jiang, G., Ogasawara, K., Endoh, A., and Sakurai, T.
(2003). Context-based ontology building support in
clinical domains using formal concept analysis. Int J
Med Inform, 71(1):71–81.
Johnson, S. B. (1999). A semantic lexicon for medical lan-
guage processing. J Am Med Inform Assoc, 6(3):205–
18.
Jonnalagadda, S., Cohen, T., Wu, S., Liu, H., and Gonzalez,
G. (2013). Using empirically constructed lexical re-
sources for named entity recognition. Biomed Inform
Insights, 6(Suppl 1):17–27.
Kate, R. J. (2013). Towards converting clinical phrases
into snomed ct expressions. Biomed Inform Insights,
6(Suppl 1):29–37.
Liu, H., Wu, S. T., Li, D., Jonnalagadda, S., Sohn, S.,
Wagholikar, K., Haug, P. J., Huff, S. M., and Chute,
C. G. (2012a). Towards a semantic lexicon for clinical
natural language processing. AMIA Annu Symp Proc,
2012:568–76.
Liu, Y., McInnes, B. T., Pedersen, T., Melton-Meaux, G.,
and Pakhomov, S. (2012b). Semantic relatedness
study using second order co-occurrence vectors com-
puted from biomedical corpora, umls and wordnet.
Proceedings of the 2nd ACM SIGHIT symposium on
International health informatics - IHI ’12.
BIOSTEC2015-DoctoralConsortium
50