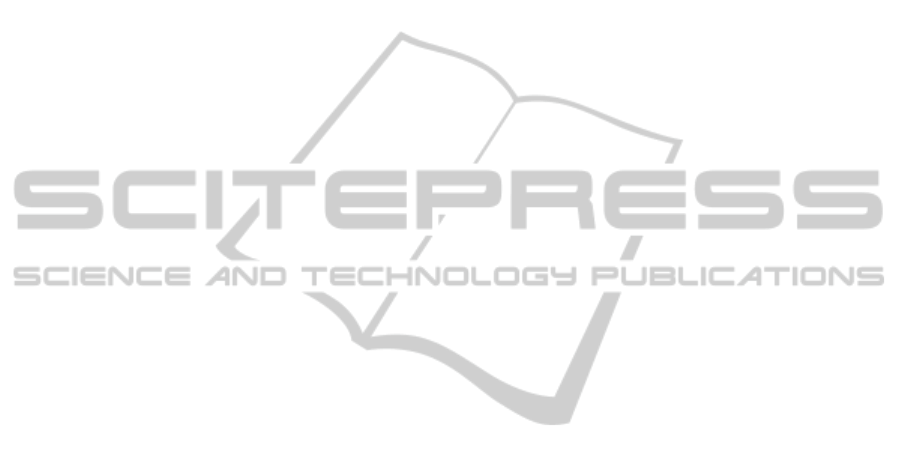
cation domains in which linguistic descriptions may
prove useful, but are mainly focused on research-
ing a preliminary linguistic description generic model
based on our previous experience. We have also re-
cently explored the current state of the art in both nat-
ural language generation systems and linguistic de-
scriptions of data in order to ascertain the role that
generic LDD approaches (and thus our model) could
play integrated into NLG systems (Ramos-Soto et al.,
2014b).
We intend to provide a model which can be di-
rectly used in practical cases but can also be formally
described, thus maintaining both the theoretical and
practical aspects of the Ph.D. objectives. Our aim is
that this model can create linguistic descriptions from
heterogeneous data-sets, although at first we will fo-
cus on time series data. We expect to further extend
this model to support data with spatial components
and also to include new concepts and capabilities as
new practical problems arise.
Furthermore, we will also explore the genera-
tion of linguistic descriptions using meta-heuristic ap-
proaches. This is a task which has been scarcely ex-
plored (Castillo-Ortega et al., 2011b) and which may
prove useful in the sense of providing a general algo-
rithm for creating linguistic descriptions. This could
also be one of the possible extensions to our model.
REFERENCES
Alvarez-Alvarez, A. and Trivino, G. (2013). Linguistic de-
scription of the human gait quality. Engineering Ap-
plications of Artificial Intelligence, 26(1):13 – 23.
Castillo-Ortega, R., Mar
´
ın, N., and S
´
anchez, D. (2011a).
A fuzzy approach to the linguistic summarization of
time series. Multiple-Valued Logic and Soft Comput-
ing, pages 157–182.
Castillo-Ortega, R., Mar
´
ın, N., S
´
anchez, D., and Tetta-
manzi, A. (2012). Quality assessment in linguistic
summaries of data. In Greco, S., Bouchon-Meunier,
B., Coletti, G., Fedrizzi, M., Matarazzo, B., and
Yager, R., editors, Advances in Computational Intel-
ligence, volume 298 of Communications in Computer
and Information Science, pages 285–294. Springer
Berlin Heidelberg.
Castillo-Ortega, R., Mar
´
ın, N., S
´
anchez, D., and Tetta-
manzi, A. G. (2011b). Linguistic summarization of
time series data using genetic algorithms. In Advances
in Intelligent Systems Research, volume 1 - 1, pages
416 – 423.
Coch, J. (1998). Multimeteo: multilingual production of
weather forecasts. ELRA Newsletter, 3(2).
Delgado, M., Ruiz, M. D., S
´
anchez, D., and Vila, M. A.
(2014). Fuzzy quantification: a state of the art. Fuzzy
Sets and Systems, 242(0):1 – 30. Theme: Quantifiers
and Logic.
Diaz-Hermida, F. and Bugarin, A. (2011). Semi-fuzzy
quantifiers as a tool for building linguistic summaries
of data patterns. In IEEE Symposium on Foundations
of Computational Intelligence, pages 45–52.
D
´
ıaz-Hermida, F., Ramos-Soto, A., and Bugar
´
ın, A. (2011).
On the role of fuzzy quantified statements in linguistic
summarization. In Proceedings of 11th International
Conference on. Intelligent Systems Design and Appli-
cations (ISDA), pages 166–171.
Eciolaza, L. and Trivino, G. (2011). Linguistic reporting of
driver behavior: Summary and event description. In
Intelligent Systems Design and Applications (ISDA),
2011 11th International Conference on, pages 148–
153.
Gamut, L. T. F. (1991). Logic, Language and Meaning.
University of Chicago Press.
Goldberg, E., Driedger, N., and Kittredge, R. (1994). Using
natural-language processing to produce weather fore-
casts. IEEE Expert, 9(2):45–53.
Kacprzyk, J. (2010). Computing with words is an im-
plementable paradigm: Fuzzy queries, linguistic data
summaries, and natural-language generation. IEEE
Trans. Fuzzy Systems, pages 451–472.
Kacprzyk, J. and Wilbik, A. (2009). Using fuzzy linguis-
tic summaries for the comparison of time series: an
application to the analysis of investment fund quo-
tations. In Proceedings IFSA/EUSFLAT Conf. 2009,
pages 1321–1326.
Kacprzyk, J. and Zadrozny, S. (2005). Linguistic database
summaries and their protoforms: towards natural lan-
guage based knowledge discovery tools. Inf. Sci. Inf.
Comput. Sci., 173(4):281–304.
Kacprzyk, J. and Zadrozny, S. (2010). Linguistic data
summarization: A high scalability through the use
of natural language? Scalable Fuzzy Algorithms for
Data Management and Analysis: Methods and De-
sign, pages 214–237.
Kobayashi, I. and Okumura, N. (2009). Verbalizing time-
series data: With an example of stock price trends. In
Proceedings IFSA/EUSFLAT Conf., pages 234–239.
Menendez, C. and Trivino, G. (2012). Selection of the best
suitable sentences in linguistic descriptions of data. In
Greco, S., Bouchon-Meunier, B., Coletti, G., Fedrizzi,
M., Matarazzo, B., and Yager, R., editors, Advances in
Computational Intelligence, volume 298 of Communi-
cations in Computer and Information Science, pages
295–304. Springer Berlin Heidelberg.
Menendez-Gonzalez, C. and Trivino, G. (2011). Olap
navigation in the granular linguistic model of a phe-
nomenon. In Computational Intelligence and Data
Mining (CIDM), 2011 IEEE Symposium on, pages
260–267.
Ramos-Soto, A., Bugar
´
ın, A., and Barro, S. (2014a). Gen-
eraci
´
on autom
´
atica de predicciones a corto plazo:
Metodolog
´
ıa y validaci
´
on. In Actas XVII Congreso
Espa
˜
nol sobre Tecnolog
´
ıas y L
´
ogica Fuzzy (ESTYLF),
pages 405–410.
Ramos-Soto, A., Bugarin, A., and Barro, S. (2014b). On the
role of linguistic descriptions of data in the building of
natural language generation systems. Submitted.
ICAART2015-DoctoralConsortium
8