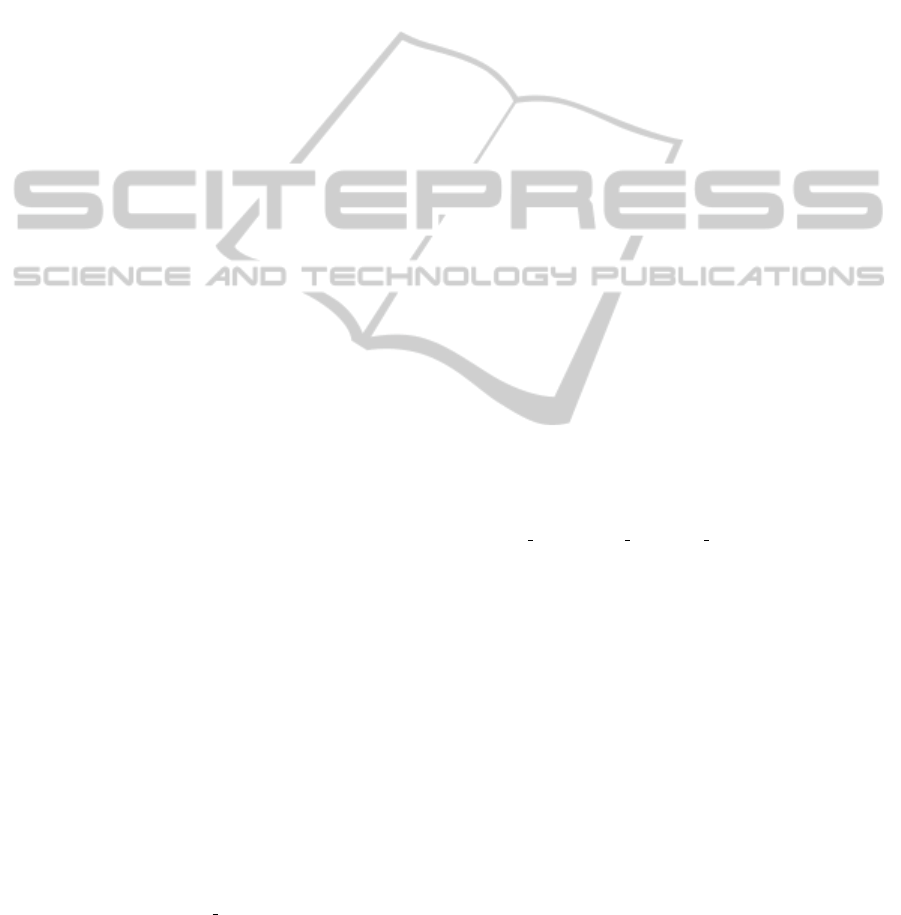
matching record ranked within the top 5, 79% of the
time. Although this percentage is currently low for a
real application deployment, this is work in progress
that lays the foundation for future work described in
the previous section, and for a feasibile application
deployment we believe.
We hope that this work will serve as a catalyst to
solve patient identification problems at on-site med-
ical clinics in less developed countries, and have a
positive impact on global health. Although the main
target of the app is identifying subjects for the pur-
pose of cataloging their medical data in field settings,
it would also be useful for on-site identification of ca-
sualties complicated by facial injury and lack of iden-
tifying documents.
ACKNOWLEDGEMENTS
We would like to thank the Biometrics Research Lab-
oratory at IIT Delhi for providing us with the IIT
Delhi Ear Image Database. We would also like to
thank Fatih Cakir for bringing together the public
health and computer science teams.
REFERENCES
Abate, A., Nappi, M., Riccio, D., and Andricciardi, S.
(2006). Ear recognition by means of a rotation in-
variant descriptor. Pattern Recognition, ICPR. 18th
International Conference on, 4:437–440.
Abaza, A., Ross, A., Hebert, C., Harrison, M. F., and Nixon,
M. S. (2013). A survey on ear biometrics. ACM Com-
puting Surveys (CSUR) Journal, 45(2):22.
Abdel-Mottaleb, M. and Zhou, J. (2005). Human ear recog-
nition from face profile images. Advances in biomet-
rics, pages 786–792.
Azfar, R. S., Weinberg, J. L., Cavric, G., Lee-Keltner, I. A.,
Bilker, W. B., Gelfand, J. M., and Kovarik, C. L.
(2011). HIV-positive patients in botswana state that
mobile teledermatology is an acceptable method for
receiving dermatology care. Journal of telemedicine
and telecare, 17(6):338–340.
Biometrics Metrics Report v3.0 (2012). Pre-
pared for: U.S. Military Academy (USMA)
- West Point. http://www.usma.edu/ietd/docs/
BiometricsMetricsReport.pdf.
Biometrics Research Laboratory (2013). IIT Delhi
Ear Database. http://www4.comp.polyu.edu.hk/
∼csajaykr/IITD/Database Ear.htm.
Boodoo-Jahangeer, N. B. and Baichoo, S. (2013). LBP-
based ear recognition. Bioinformatics and Bioengi-
neering (BIBE), IEEE 13th International Conference
on, pages 1–4.
Bradski, G. (2000). Dr. Dobb’s Journal of Software Tools.
Chang, K., Bowyer, K., Sarkar, S., and Victor, B. (2003).
Comparison and combination of ear and face im-
ages in appearance-based biometrics. Pattern Anal-
ysis and Machine Intelligence, IEEE Transactions on,
25(9):1160–1165.
Cummings, A., Nixon, M., and Carter, J. (2010). A novel
ray analogy for enrollment of ear biometrics. Biomet-
rics: Theory Applications and Systems (BTAS), Fourth
IEEE International Conference on, pages 1–6.
Delac, K. and Grgic, M. (2004). A survey of biometric
recognition methods. Electronics in Marine, 2004.
Proceedings Elmar 2004. 46th International Sympo-
sium, pages 184–193.
Fahmi, A., Kodirov, E., Choi, D., Lee, G., M. F. Azli A.,
and Sayeed, S. (2012). Implicit authentication based
on ear shape biometrics using smartphone camera dur-
ing a call. Systems, Man, and Cybernetics (SMC),
IEEE International Conference on, pages 2272–2276.
Goode, A. (2014). Bring your own finger–how mobile is
bringing biometrics to consumers. Biometric Technol-
ogy Today, 2014(5):5–9.
Iannarelli, A. (1989). Ear identification, forensic identifica-
tion series, fremont. Paramont Publishing Company,
Calif, ISBN, 10:0962317802.
Kisku, D. R., Mehrota, H., Gupta, P., and Sing, J. K. (2009).
SIFT-based ear recognition by fusion of detected key-
points from color similarity slice regions. Advances
in Computational Tools for Engineering Applications,
2009. ACTEA’09. International Conference on, pages
380–385.
Kumar, A. and Wu, C. (2012). Automated human iden-
tification using ear imaging. Pattern Recognition,
45(3):956–968.
Kumar, M. (2014). Hanseatic institute of
technology. cell phone-based intelligent
biometrics. http://www.appropedia.org/
Cell
phone-based intelligent biometrics .
Kwapisz, J. R., Weiss, G. M., and Moore, S. A. (2010).
Cell phone-based biometric identification. Biomet-
rics: Theory Applications and Systems (BTAS), Fourth
IEEE International Conference on, pages 1–7.
Lowe, D. (1999). Object recognition from local scale-
invariant features. Computer vision. The proceed-
ings of the seventh IEEE international conference on,
2:1150–1157.
M
¨
aenp
¨
a
¨
a, T., Ojala, T., Pietik
¨
ainen, M., and Soriano, M.
(2000). Robust texture classification by subsets of lo-
cal binary patterns. Pattern Recognition, 2000. Pro-
ceedings. 15th International Conference on, 3:935–
938.
Ojala, T., Pietikainen, M., and Harwood, D. (1996). A com-
parative study of texture measures with classification
based on feature distributions. Pattern Recognition,
29(1):51–59.
Takala, V., Ahonen, T., and Pietik
¨
ainen, M. (2005). Block-
based methods for image retrieval using local binary
patterns. Image Analysis, pages 882–891.
Vedaldi, A. and Fulkerson, B. (2008). VLFeat: An open
and portable library of computer vision algorithms.
http://www.vlfeat.org/.
VISAPP2015-InternationalConferenceonComputerVisionTheoryandApplications
178