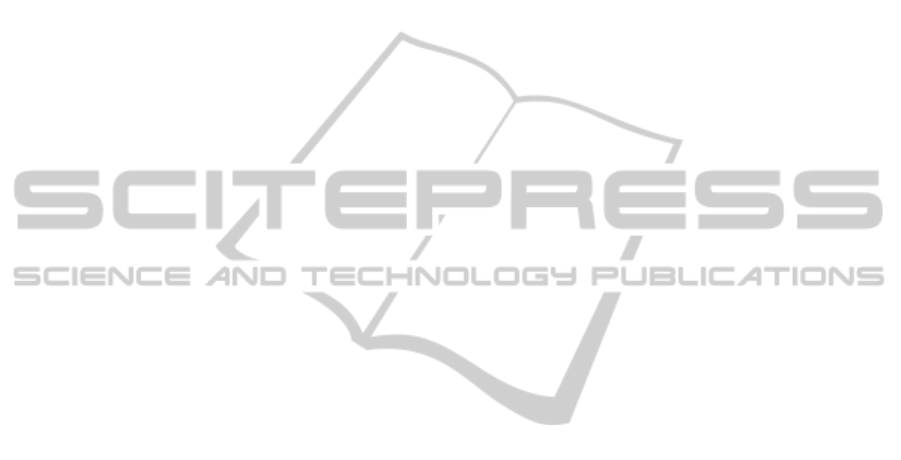
5 CONCLUSIONS
In this paper, we proposed a novel interest point
detector named as Robust Local Zernike Moment
based Features or R-LZMF. This detector is based
on local Zernike moments and invariant to geometric
transformations such as scale, rotation and
translation. We validated its robustness to these
transformations by testing it with the Inria Dataset
and reported that R-LZMF outperforms SIFT,
SURF, CenSurE (STAR), BRISK and ORB for all
image sets in the experiments. As a future work, we
plan to analyse the performance of R-LZMF for
affine transformation as well. Furthermore, we will
extend R-LZMF to have a descriptor by using LZM
again to utilize from its descriptive power so that it
will be a complete schema (detector and descriptor)
as in SIFT and SURF.
REFERENCES
Agrawal, M., Konolige, K., Blas, M. R., 2008. CenSurE:
Center surround extremas for realtime feature
detection and matching. In European Conference on
Computer Vision, pp. 102-115.
Bay, H., Ess, A., Tuytelaars, T., Gool, L.V., 2008. SURF:
Speeded Up Robust Features. In Computer Vision and
Image Understanding, vol. 110, no. 3, pp. 346-359.
Calonder, M., Lepetit V., Strecha, C., Fua, P., 2010.
BRIEF: Binary Robust Independent Elementary
Features. In European Conference on Computer
Vision, pp. 778-792.
Ghosal, S., Mehrotra, R., 1997. A moment based unified
approach to image feature detection. In IEEE Trans.
Image Processing, vol. 6, no. 6, pp. 781-793.
Harris, C., Stephens, M., 1988. A combined corner and
edge detector. Alvey Vision Conference, pp. 147-151.
Khotanzad, A., Hong, Y. H., 1990. Invariant image
recognition by Zernike moments. In IEEE
Trans.Pattern Analysis and Machine Intelligence, vol.
12, pp. 489-497.
Koenderink, J.J., 1984. The structure of images.
Biological Cybernetics, 50:363–396.
Leutenegger, S., Chli, M., Siegwart R., 2011. BRISK:
Binary Robust Invariant Scalable Keypoints. In
International Conference on Computer Vision, pp.
2548-2555.
Lindeberg, T., 1998. Feature detection with automatic
scale selection. In International Journal of Computer
Vision, 30(2):79-116.
Lowe, D.G., 2004. Distinctive image features from scale-
invariant keypoints. In International Journal of
Computer Vision, vol. 60, no. 2, pp. 91-110.
Mikolajczyk, K., Schmid, C., 2001. Indexing based on
scale invariant interest points. In International
Conference on Computer Vision, pp. 525-531.
Mikolajczyk, K., Schmid, C., 2002. An affine invariant
interest point detector. In European Conference on
Computer Vision, pp. 128-142.
Mikolajczyk, K., Schmid, C., 2004. Scale and affine
invariant interest point detectors. In International
Journal of Computer Vision, vol. 60, no. 1, pp. 63-86.
Özbulak, G., Gökmen, M., 2014. A rotation invariant local
Zernike moment based interest point detector. In Proc.
SPIE of International Conference on Machine Vision.
Rosten, E., Drummond, T., 2006. Machine learning for
high-speed corner detection. In European Conference
on Computer Vision, pp. 430-443.
Rublee, E., Rabaud, V., Konolige, K., Bradski, G., 2011.
ORB: an efficient alternative to SIFT or SURF. In
Internatioanl Conference on Computer Vision, pp.
2564-2571.
Sariyanidi, E., Dagli, V., Tek, S.C., Tunc, B., Gokmen,
M., 2012. Local Zernike Moments: A new
representation for face recognition. In International
Conference on Image Processing, pp. 585-588.
Schmid, C., Mohr, R., Bauckhage, C., 1998. Comparing
and evaluating interest points. In IEEE International
Conference on Computer Vision, pp. 230-235.
Teague, M.R., 1980. Image analysis via the general theory
of moments, In J. Optical Soc. Am., Vol. 70, pp. 920-
930.
The Inria Dataset, http://lear.inrialpes.fr/people/
mikolajczyk/Database.
Witkin, A.P., 1983. Scale-space filtering. In International
Joint Conference on Artificial Intelligence, Karlsruhe,
Germany, pp. 1019–1022.
Zernike, F., 1934. Physica, vol. 1.
RobustInterestPointDetectionbyLocalZernikeMoments
651