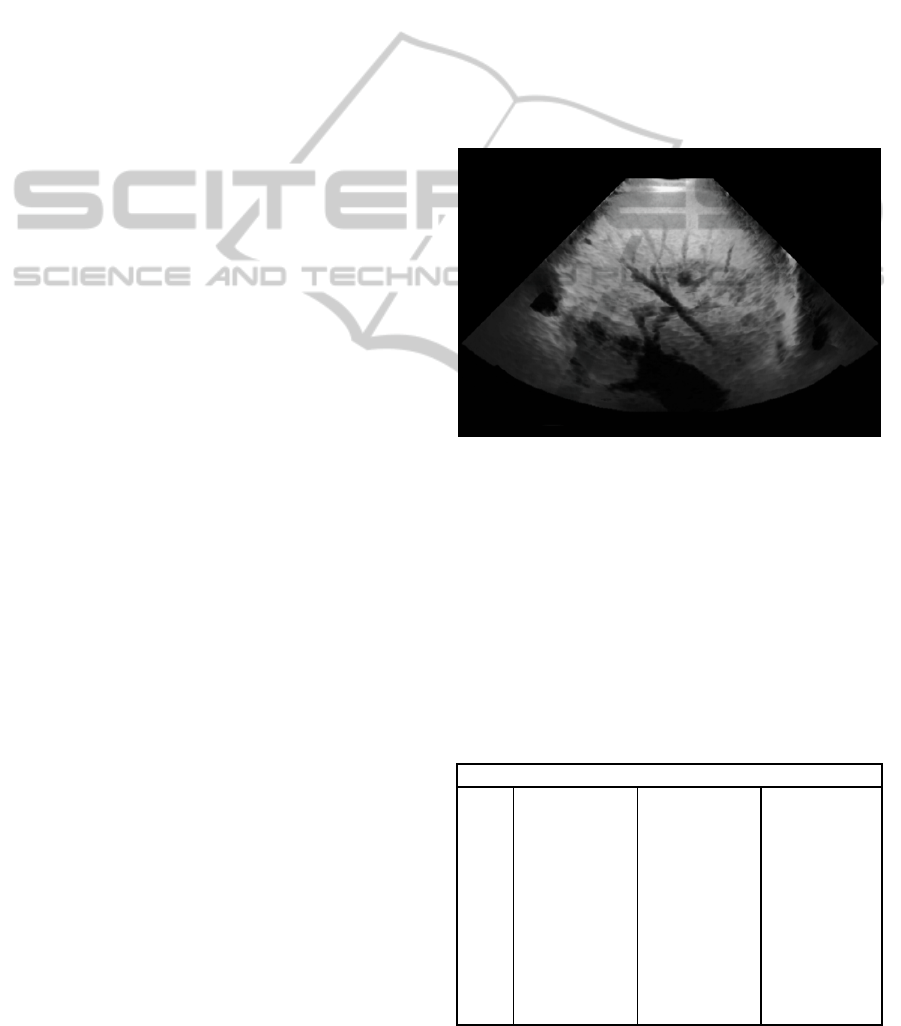
saving of many OpenGL calls regarding the volume
bounding box creation, it should be faster than
multi-pass raycasting. The disadvantage is the full
computational load on the fragment shader
(Venkataraman, 2009) which could slow down the
raycasting.
Different optimization techniques can be
implemented to lower execution times (ETs). One of
the most common techniques is the “Early Ray
Termination” (ERT) (Matsui, et al., 2005). It was
implemented because of its high potential of
lowering the ET while being easy to realize in
OpenGLSL.
Currently the required parameters to choose the
optimal raycasting technique for a given application
are unknown. The comparison reported here shall be
a first contribution to clarify this issue.
2 MATERIALS AND METHODS
Both raycasting techniques were implemented using
C# and OpenGLSL 4.3 (with OpenGL4Net
(Vanecek, 2014)). The engaged hardware consists of
a notebook (Windows 7 SP1, Intel Core i5-4200M
CPU, 2.5 GHz, 16 GB RAM) with an NVIDIA
Quadro K3100M (driver version 312.32) graphics
card. The raycasting is performed using an
ultrasound image volume with 512×378×222
grayscale values (8bit, unsigned integer), a voxel
spacing of 0.42 mm×0.39 mm×0.63 mm and a
viewport size of 1440×900.
For the comparison three different raycasting
types were implemented with and without ERT.
1. “Minimum Intensity Projection” (MINIP)
(Radiopaedia.org, 2014) - a simple rendering
technique to visualize e.g. liver vessels in
ultrasound volumes (figure 2) – as a technique
with low computing time.
2. Alpha Blending (Porter & Duff, 1984) as a
technique with medium computing time.
3. Gradient Calculation using the left and right
neighbor of each pixel along the ray as a
technique with high computing time due to many
texture fetches.
To measure the ET of the raycasting techniques the
following steps were realized:
1. Implement both raycasting techniques as
described above.
2. Implement in-application profiling using
OpenGL “elapsed time queries” (Shreiner, et al.,
2013)
a. Single-pass: At the end of each “paint”-call
b. Multi-pass: At the end of the volume
bounding box building and at the end of each
“paint”-call
3. Load the ultrasound dataset.
4. Choose the raycasting type.
5. Activate or deactivate ERT.
6. Set the step size to a defined value from 0.001 to
0.05 mm (table 1).
7. Perform an arc shot with an angular step size of 1
(= sum of 360 steps) and call “paint” after each
angular step.
8. Repeat step 5 ten times to have a sum of 3600
(single-pass) respectively 7200 (multi-pass)
profiling values per step size.
9. Average the ETs and add them to table 1.
10. Repeat step 4 to 9 for every raycasting type.
Figure 2: Ultrasound volume visualization (MINIP) of
liver vessels.
3 RESULTS
The results of the ET measurements for the three
raycasting types can be seen in tables 1 to 3. To
show the change of ET ratio, the quotients of multi-
pass ETs divided by single-pass ETs were
calculated.
Table 1: MINIP ET (times in milliseconds).
MINIP
step
size
[mm]
single-pass multi-pass quotient
raycast ERT raycast ERT raycast ERT
0.001 226.3 180.4 126.0 101.4 0.6 0.6
0.002 118.7 99.1 78.6 67.8 0.7 0.7
0.005 49.3 43.8 47.6 43.3 1.0 1.0
0.01 26.6 23.5 36.8 33.7 1.4 1.4
0.02 15.5 14.1 30.5 28.4 2.0 2.0
0.05 8.6 8.6 27.9 26.0 3.2 3.0
IVAPP2015-InternationalConferenceonInformationVisualizationTheoryandApplications
308