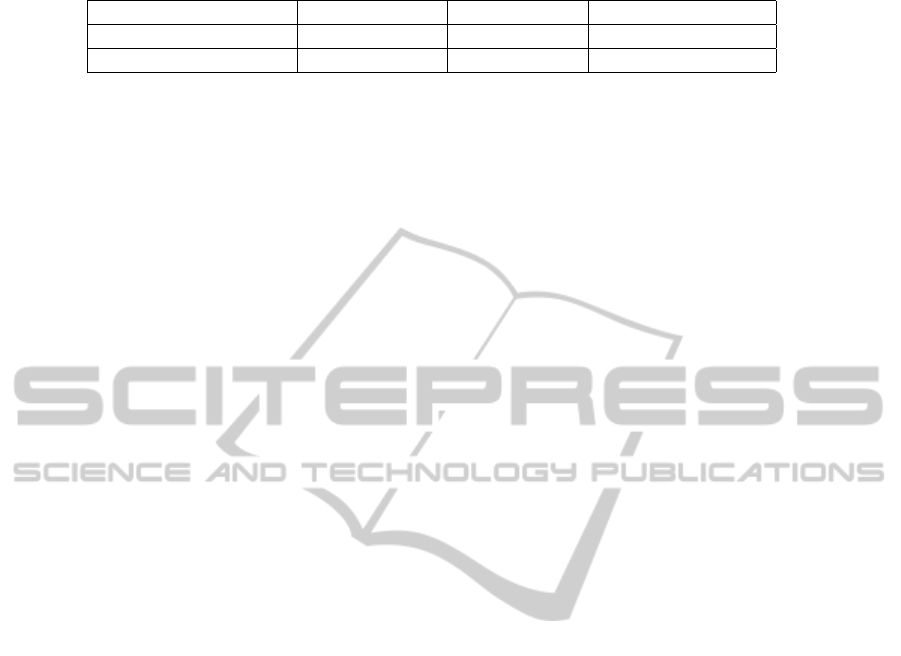
Table 3: Results comparison between this paper and the one published in (Costa Filho et al., 2012).
Publication Sensitivity (%) Precision (%) False detection (%)
Present paper 94.25 92.50 7.50
Costa Filho et al., 2012 91.53 91.49 8.51
ages segmentation to be fast, and the application of
cross validation to analyze the effectiveness of the
classification of the structures.
ACKNOWLEDGEMENTS
The authors would like to acknowledge the finan-
cial support received from the National Council for
Scientific and Technological Development CNPq,
Brazil. The authors also gratefully acknowledge
CAPES (Brazil) by the financial support to attend at
VISAPP 2015.
REFERENCES
Bishop, C. M. et al. (2006). Pattern recognition and ma-
chine learning, volume 1. springer New York.
CETELI (2014). An image database of conven-
tional sputum smear microscopy for tuber-
culosis. Center for Research and Develop-
ment in Electronic and Information Technology.
http://http://www.tbimages.ufam.edu.br/.
Chayadevi, M. and Raju, G. (2014). Automated colour seg-
mentation of tuberculosis bacteria thru region grow-
ing: A novel approach. In Applications of Digital
Information and Web Technologies (ICADIWT), 2014
Fifth International Conference on the, pages 154–159.
IEEE.
Costa, L. F. and Cesar Jr, R. M. (2000). Shape analysis and
classification: theory and practice. CRC press.
Costa, M. G., Costa Filho, C. F., Sena, J. F., Salem, J., and
de Lima, M. O. (2008). Automatic identification of
mycobacterium tuberculosis with conventional light
microscopy. In Engineering in Medicine and Biology
Society, 2008. EMBS 2008. 30th Annual International
Conference of the IEEE, pages 382–385. IEEE.
Costa Filho, C. F. F., Levy, P. C., Xavier, C. M., Costa,
M. G., Fujimoto, L. B., and Salem, J. (2012). My-
cobacterium tuberculosis recognition with conven-
tional microscopy. In Engineering in Medicine and
Biology Society (EMBC), 2012 Annual International
Conference of the IEEE, pages 6263–6268. IEEE.
Desikan, P. (2013). Sputum smear microscopy in tubercu-
losis: Is it still relevant? volume 137, pages 442–444.
Duda, R. O., Hart, P. E., and Stork, D. G. (2001). Pattern
classification. Wiley, 2nd edition.
Gonzalez, R. C. and Woods, R. E. (2007). Digital Image
Processing. Prentice Hall, 3rd edition.
Haykin, S. S., Haykin, S. S., Haykin, S. S., and Haykin,
S. S. (2009). Neural networks and learning machines,
volume 3. Pearson Education Upper Saddle River.
Kusworo, A., Rahmat, G., Aris, S., Adi, P., Ari, B., and
Nelly, M. (2013). Autothresholding segmentation
for tuberculosis bacteria identification in the ziehl-
neelsen sputum sample. In Proceedings The 7th In-
ternational Conference on Information & Communi-
cation Technology and Systems (ICTS), pages 15–16.
Makkapati, V., Agrawal, R., and Acharya, R. (2009). Seg-
mentation and classification of tuberculosis bacilli
from zn-stained sputum smear images. In Automation
Science and Engineering, 2009. CASE 2009. IEEE In-
ternational Conference on, pages 217–220. IEEE.
Nayak, R., Shenoy, V. P., and Galigekere, R. R. (2010).
A new algorithm for automatic assessment of the de-
gree of tb-infection using images of zn-stained spu-
tum smear. In Systems in Medicine and Biology (IC-
SMB), 2010 International Conference on, pages 294–
299. IEEE.
Sadaphal, P., Rao, J., Comstock, G., and Beg, M. (2008).
Image processing techniques for identifying my-
cobacterium tuberculosis in ziehl-neelsen stains [short
communication]. The International Journal of Tuber-
culosis and Lung Disease, 12(5):579–582.
Siena, I., Adi, K., Gernowo, R., and Miransari, N. (2012).
Development of algorithm tuberculosis bacteria iden-
tification using color segmentation and neural net-
works. International Journal of Video and Image Pro-
cessing and Network Security, 12(4):9–13.
Smart, T. (2007). Background on smear microscopy in tb
diagnosis. http://www.aidsmap.com/Background-on-
smear-microscopy-in-TB-diagnosis/page/1426650/.
Sotaquir
´
a, M., Rueda, L., and Narvaez, R. (2009). Detec-
tion and quantification of bacilli and clusters present
in sputum smear samples: a novel algorithm for pul-
monary tuberculosis diagnosis. In Digital Image
Processing, 2009 International Conference on, pages
117–121. IEEE.
WHO (2014). Tuberculosis (tb). World Health Organiza-
tion. http://www.who.int/tb/.
Witten, I. H. and Frank, E. (2005). Data Mining: Practi-
cal machine learning tools and techniques. Morgan
Kaufmann.
Zhai, Y., Liu, Y., Zhou, D., and Liu, S. (2010). Automatic
identification of mycobacterium tuberculosis from zn-
stained sputum smear: Algorithm and system design.
In Robotics and Biomimetics (ROBIO), 2010 IEEE In-
ternational Conference on, pages 41–46. IEEE.
AutomaticIdentificationofMycobacteriumtuberculosisinZiehl-NeelsenStainedSputumSmearMicroscopyImagesusing
aTwo-stageClassifier
191