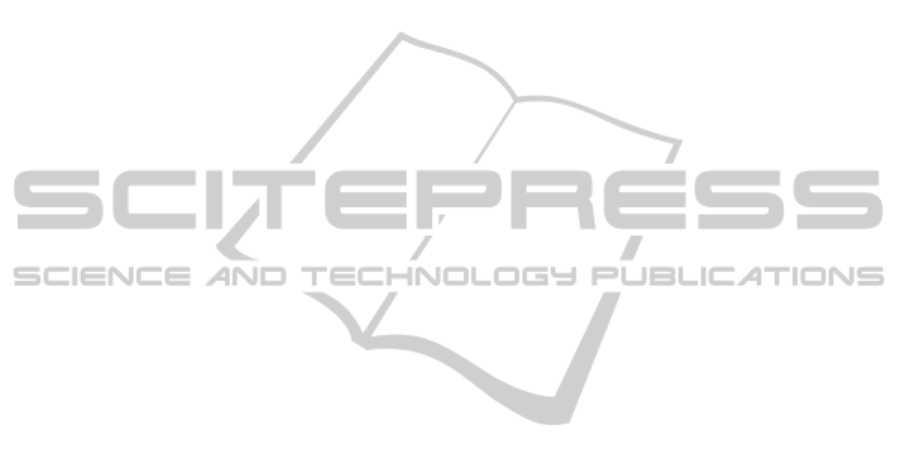
Yearly/Yearly). This happens because the location-
vector becomes sparser when increasing the time
period while the sparseness of the topic-vector
nearly remains the same.
5 CONCLUSIONS
In this paper we introduced a novel similarity
measurement framework that relies on the dynamic
model of twitter users. This framework utilizes the
dynamic attributes of user by extracting and ranking
their topics and visited location categories during a
specific time intervals. It also applied an integrated
method that linearly combines the similarity values
between weighted topical interests and locational
vectors during a predefined time intervals.
The experimental results show that the proposed
method for calculating the similarity outperforms
several traditional models that consider only a static
snapshot of user published content and behavior.
The results also prove that when considering the
time factor for calculating the similarity always gave
better accuracy than using static snapshots of
dynamic data. This superior accuracy is achieved
whenever each dynamic attributes was individually
considered and also when applying the integrated
model. In future work, in order to enhance the
proposed framework, we intend to make it more
adaptive to the changes in interests of users over any
time. We also consider linking topics and location
categories into higher ontology hierarchy that will
better represent their interest and behavior in twitter.
REFERENCES
Abel, F., Gao, Q., Houben, G.-j. and Tao, K. (2011)
'Analyzing User Modeling on Twitter for Personalized
News Recommendations', in User Modeling, Adaption
and Personalization, pp.1-12.
Benevenuto, F., Rodrigues, T., Cha, M. and Almeida, V.
(2009) 'Characterizing User Behavior in Online Social
Networks', Proceedings of the 9th ACM SIGCOMM
conference on Internet measurement conference.
ACM, 49-62.
Blanco-Fernández, Y., López-Nores, M., Pazos-Arias, J.J.
and García-Duque, J. (2011) 'An improvement for
semantics-based recommender systems grounded on
attaching temporal information to ontologies and user
profiles', Engineering Applications of Artificial
Intelligence, vol. 24, pp. 1385-1397.
Gangemi, A. (2013) 'A Comparison of Knowledge
Extraction Tools for the Semantic Web', in The
Semantic Web: Semantics and Big Data, Springer
Berlin Heidelberg, pp.351-366.
Kleanthous, S. and Dimitrova, V. (2008) 'Modelling
Semantic Relationships and Centrality to Facilitate
Community Knowledge Sharing', in Adaptive
Hypermedia and Adaptive Web-Based Systems,
Springer Berlin Heidelberg, pp. 123-132.
Kleanthous, S. and Dimitrova, V. (2010) 'Analyzing
Community Knowledge Sharing Behavior' in Adaptive
Hypermedia and Adaptive Web-Based Systems,
Springer Berlin Heidelberg, pp. 231-242.
Lee, M.-j. and Chung, C.-w. (2011) 'A User Similarity
Calculation Based on the Location for Social Network
Services', 16th international conference on Database
systems for advanced applications, 38-52.
Li, Q., Zheng, Y., Xie, X., Chen, Y., Liu, W. and Ma, W.-
Y. (2008) 'Mining user similarity based on location
history', Proceedings of the 16th ACM SIGSPATIAL
international conference on Advances in geographic
information system ,ACM, 34.
McKenzie, G., Adams, B. and Janowicz, K. (2013) 'A
thematic approach to user similarity built on geosocial
check-ins.', in Geographic Information Science at the
Heart of Europe., Springer International Publishing,
pp. 39-53.
Mislove, A., Viswanath, B., Gummadi, K.P. and Druschel,
P. (2010) 'You Are Who You Know : Inferring User
Profiles in Online Social Networks', Third ACM
International Conference on Web Search and Data
Mining - WSDM 2010, pp. 251-260.
Quercia, D., Askham, H. and Crowcroft, J. (2012)
'TweetLDA : Supervised Topic Classification and
Link Prediction in Twitter', Proceedings of the 3rd
Annual ACM Web Science Conference, 247-250.
Takahashi, T., Tomioka, R. and Yamanishi, K. (2014)
'Discovering Emerging Topics in Social Streams via
Link-Anomaly Detection', IEEE Transactions on
Knowledge and Data Engineering, vol. 26, no. 1, pp.
120-130.
DynamicModelingofTwitterUsers
593